Predictive Analytics for Readmission of Patients with Congestive Heart Failure
Periodicals(2015)
摘要
AbstractMitigating preventable readmissions, where patients are readmitted for the same primary diagnosis within 30 days, poses a significant challenge to the delivery of high-quality healthcare. Toward this end, we develop a novel, predictive analytics model, termed as the beta geometric Erlang-2 BG/EG hurdle model, which predicts the propensity, frequency, and timing of readmissions of patients diagnosed with congestive heart failure CHF. This unified model enables us to answer three key questions related to the use of predictive analytics methods for patient readmissions: whether a readmission will occur, how often readmissions will occur, and when a readmission will occur. We test our model using a unique data set that tracks patient demographic, clinical, and administrative data across 67 hospitals in North Texas over a four-year period. We show that our model provides superior predictive performance compared to extant models such as the logit, BG/NBD hurdle, and EG hurdle models. Our model also allows us to study the association between hospital usage of health information technologies IT and readmission risk. We find that health IT usage, patient demographics, visit characteristics, payer type, and hospital characteristics, are significantly associated with patient readmission risk. We also observe that implementation of cardiology information systems is associated with a reduction in the propensity and frequency of future readmissions, whereas administrative IT systems are correlated with a lower frequency of future readmissions. Our results indicate that patient profiles derived from our model can serve as building blocks for a predictive analytics system to identify CHF patients with high readmission risk.
更多查看译文
关键词
patient readmissions, healthcare information technologies, congestive heart failure, predictive healthcare analytics
AI 理解论文
溯源树
样例
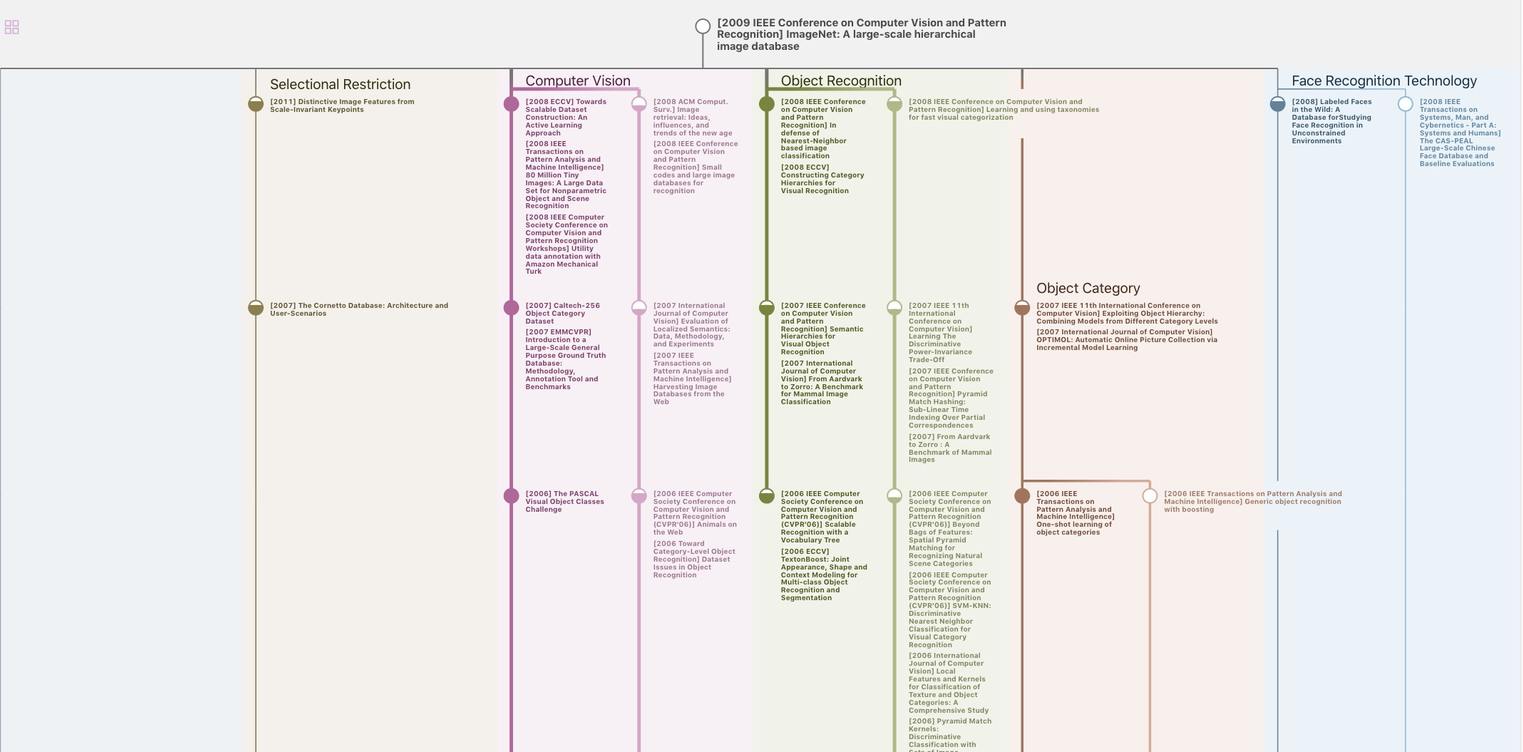
生成溯源树,研究论文发展脉络
Chat Paper
正在生成论文摘要