A Probabilistic Associative Model For Segmenting Weakly Supervised Images
IEEE TRANSACTIONS ON IMAGE PROCESSING(2014)
摘要
Weakly supervised image segmentation is an important yet challenging task in image processing and pattern recognition fields. It is defined as: in the training stage, semantic labels are only at the image-level, without regard to their specific object/scene location within the image. Given a test image, the goal is to predict the semantics of every pixel/superpixel. In this paper, we propose a new weakly supervised image segmentation model, focusing on learning the semantic associations between superpixel sets (graphlets in this paper). In particular, we first extract graphlets from each image, where a graphlet is a small-sized graph measures the potential of multiple spatially neighboring superpixels (i.e., the probability of these superpixels sharing a common semantic label, such as the sky or the sea). To compare different-sized graphlets and to incorporate image-level labels, a manifold embedding algorithm is designed to transform all graphlets into equal-length feature vectors. Finally, we present a hierarchical Bayesian network to capture the semantic associations between postembedding graphlets, based on which the semantics of each superpixel is inferred accordingly. Experimental results demonstrate that: 1) our approach performs competitively compared with the state-of-the-art approaches on three public data sets and 2) considerable performance enhancement is achieved when using our approach on segmentation-based photo cropping and image categorization.
更多查看译文
关键词
Probabilistic model, weakly-supervised, segmentation, associations
AI 理解论文
溯源树
样例
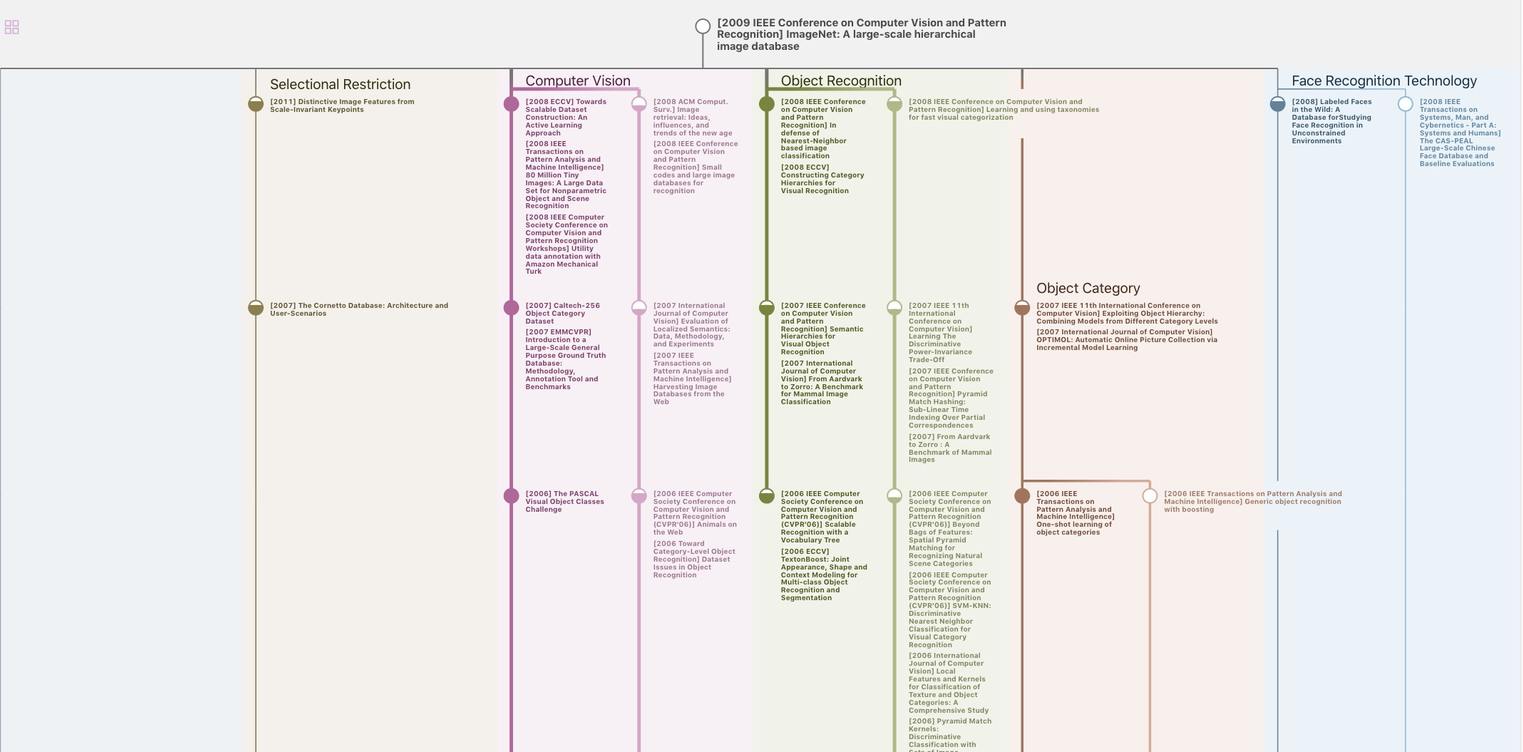
生成溯源树,研究论文发展脉络
Chat Paper
正在生成论文摘要