Reweighted low-rank matrix recovery and its application in image restoration.
IEEE T. Cybernetics(2014)
摘要
In this paper, we propose a reweighted low-rank matrix recovery method and demonstrate its application for robust image restoration. In the literature, principal component pursuit solves low-rank matrix recovery problem via a convex program of mixed nuclear norm and l1 norm. Inspired by reweighted l1 minimization for sparsity enhancement, we propose reweighting singular values to enhance low rank of a matrix. An efficient iterative reweighting scheme is proposed for enhancing low rank and sparsity simultaneously and the performance of low-rank matrix recovery is prompted greatly. We demonstrate the utility of the proposed method both on numerical simulations and real images/videos restoration, including single image restoration, hyperspectral image restoration, and background modeling from corrupted observations. All of these experiments give empirical evidence on significant improvements of the proposed algorithm over previous work on low-rank matrix recovery.
更多查看译文
关键词
convex programming,image restoration,matrix algebra,minimisation,principal component analysis,singular value decomposition,video signal processing,ℓ1 norm,background modeling,convex program,hyperspectral image restoration,iterative reweighting scheme,mixed nuclear norm,numerical simulations,principal component pursuit,reweighted ℓ1 minimization,reweighted low-rank matrix recovery,reweighting singular values,sparsity enhancement,video restoration,Image restoration,iterative reweighting,low-rank matrix recovery,nonuniform singular value thresholding
AI 理解论文
溯源树
样例
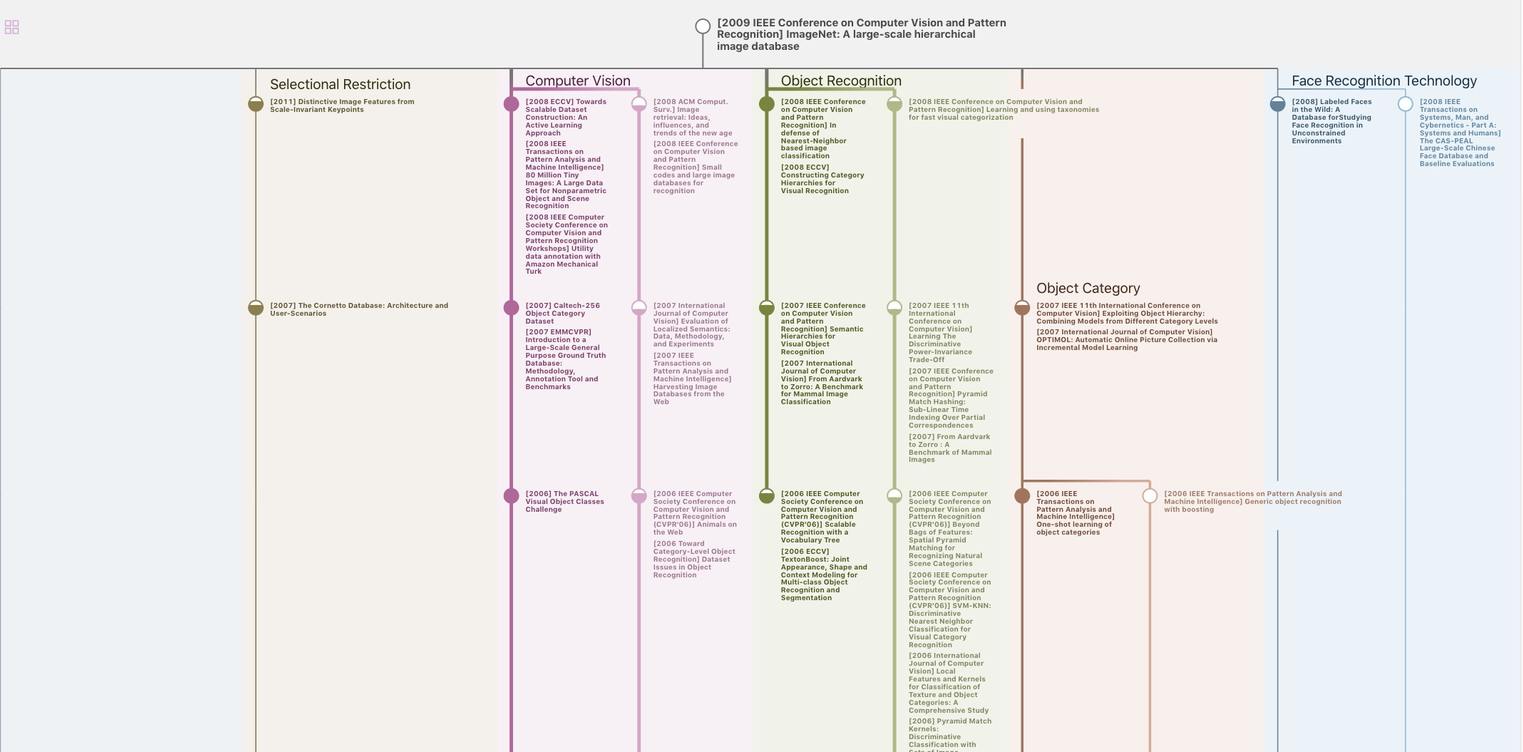
生成溯源树,研究论文发展脉络
Chat Paper
正在生成论文摘要