A modular approach for lexical normalization applied to Spanish tweets.
Expert Syst. Appl.(2015)
摘要
An extensible and modular approach for normalizing Spanish tweets is proposed.We make use of lightweight resources build with low manual effort.System performance is also analyzed module-wise and phenomenon-wise.The domain adaptability of our proposed system is easy and successful.The performance increases if a classifier-based reranking process is introduced. Twitter is a social media platform with widespread success where millions of people continuously express ideas and opinions about a myriad of topics. It is a huge and interesting source of data but most of these texts are usually written hastily and very abbreviated, rendering them unsuitable for traditional Natural Language Processing (NLP). The two main contributions of this work are: the characterization of the textual error phenomena in Twitter and the proposal of a modular normalization system that improves the textual quality of tweets. Instead of focusing on a single technique, we propose an extensible normalization system that relies on the combination of several independent \"expert modules\", each one addressing an very specific error phenomenon in its own way, thus increasing module accuracy and lowering the module building costs. Broadly speaking, the system resembles to an \"expert board\": modules independently propose correction candidates for each Out of Vocabulary (OOV) word, rank the candidates and the best one is selected. In order to evaluate our proposal, we perform several experiments using texts from Twitter written in Spanish about a specific topic. The flexibility of defining resources at different language levels (core language, domain, genre) combined with the modular architecture lead to lower costs and a good performance: requiring a minimal effort for building the resources and achieving more than 82 % of accuracy compared to the 31 % yielded by the baseline.
更多查看译文
关键词
Twitter,Text normalization,Domain adaptation
AI 理解论文
溯源树
样例
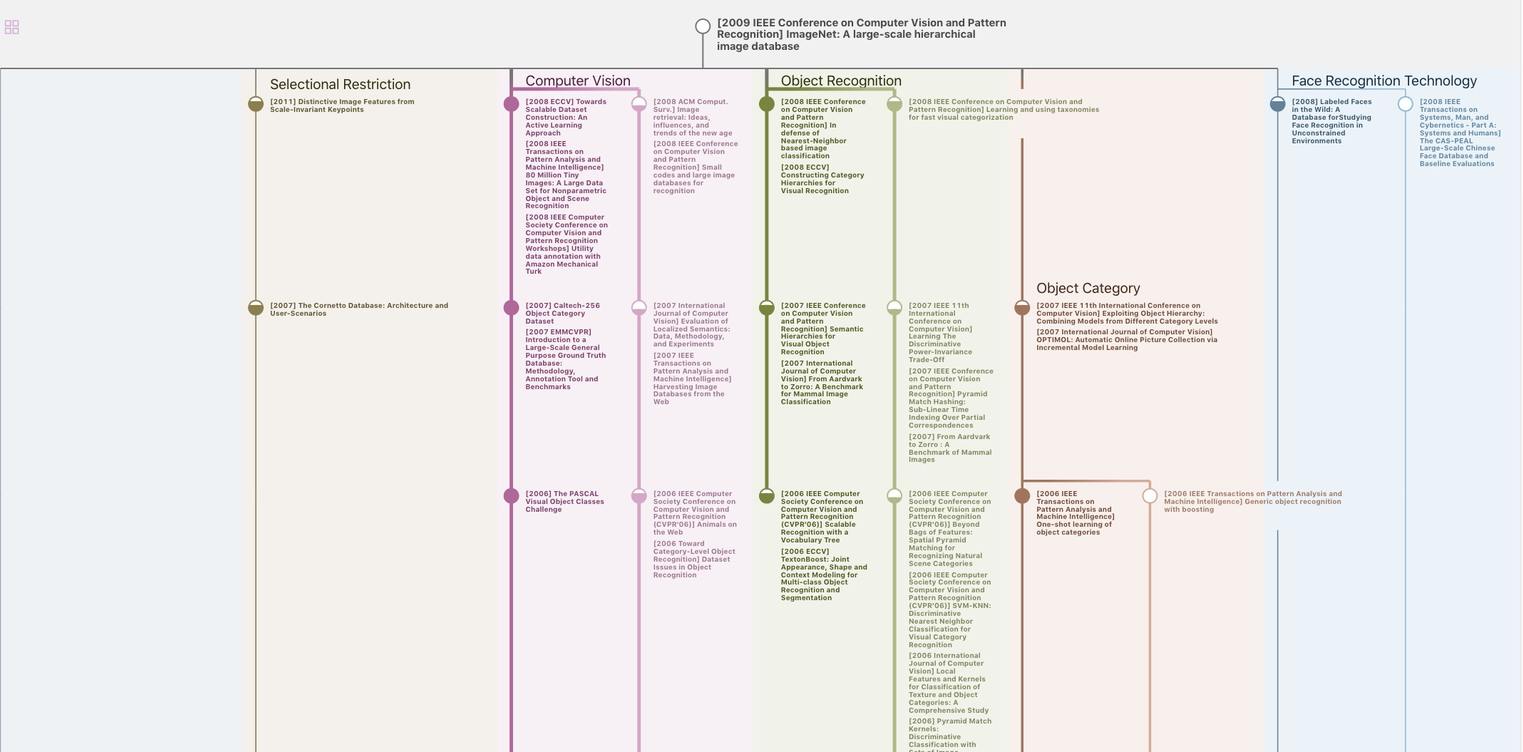
生成溯源树,研究论文发展脉络
Chat Paper
正在生成论文摘要