Context-Aware Energy Enhancements for Smart Mobile Devices.
IEEE Trans. Mob. Comput.(2014)
摘要
Within the past decade, mobile computing has morphed into a principal form of human communication, business, and social interaction. Unfortunately, the energy demands of newer ambient intelligence and collaborative technologies on mobile devices have greatly overwhelmed modern energy storage abilities. This paper proposes several novel techniques that exploit spatiotemporal and device context to predict device wireless data and location interface configurations that can optimize energy consumption in mobile devices. These techniques, which include variants of linear discriminant analysis, linear logistic regression, non-linear logistic regression with neural networks, k-nearest neighbor, and support vector machines are explored and compared on synthetic and user traces from real-world usage studies. The experimental results show that up to 90% successful prediction is possible with neural networks and k-nearest neighbor algorithms, improving upon prediction strategies in prior work by approximately 50%. Further, an average improvement of 24% energy savings is achieved compared to state-of-the-art prior work on energy-efficient location-sensing.
更多查看译文
关键词
Context,Machine learning algorithms,Mobile handsets,Algorithm design and analysis,Global Positioning System,Sensors,Mathematical model
AI 理解论文
溯源树
样例
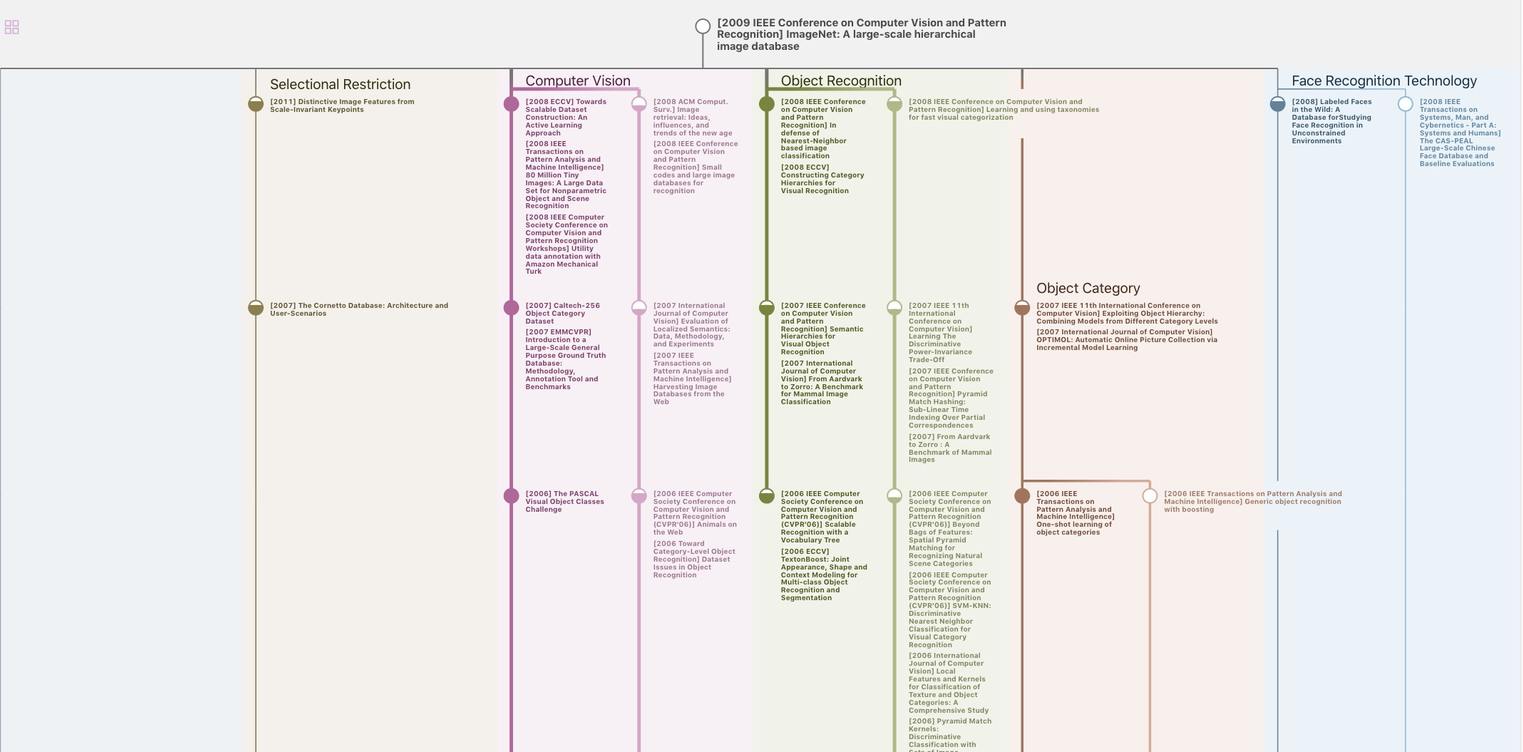
生成溯源树,研究论文发展脉络
Chat Paper
正在生成论文摘要