Video summarization via minimum sparse reconstruction.
Pattern Recognition(2015)
摘要
The rapid growth of video data demands both effective and efficient video summarization methods so that users are empowered to quickly browse and comprehend a large amount of video content. In this paper, we formulate the video summarization task with a novel minimum sparse reconstruction (MSR) problem. That is, the original video sequence can be best reconstructed with as few selected keyframes as possible. Different from the recently proposed convex relaxation based sparse dictionary selection method, our proposed method utilizes the true sparse constraint L0 norm, instead of the relaxed constraint L2,1 norm, such that keyframes are directly selected as a sparse dictionary that can well reconstruct all the video frames. An on-line version is further developed owing to the real-time efficiency of the proposed MSR principle. In addition, a percentage of reconstruction (POR) criterion is proposed to intuitively guide users in obtaining a summary with an appropriate length. Experimental results on two benchmark datasets with various types of videos demonstrate that the proposed methods outperform the state of the art.
更多查看译文
关键词
Video summarization,Keyframe extraction,Sparse reconstruction,Dictionary selection
AI 理解论文
溯源树
样例
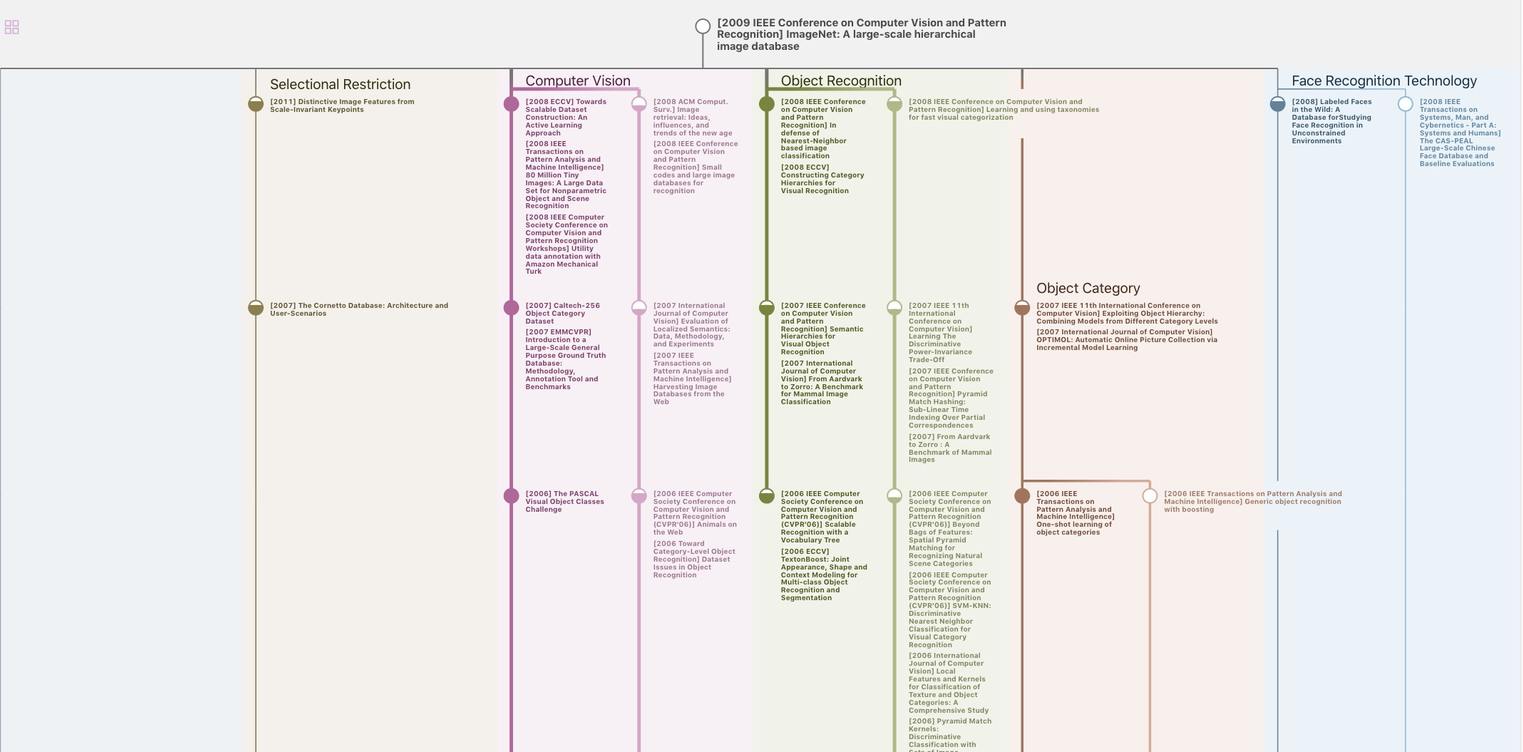
生成溯源树,研究论文发展脉络
Chat Paper
正在生成论文摘要