Hashing With List-Wise Learning To Rank
SIGIR '14: The 37th International ACM SIGIR Conference on Research and Development in Information Retrieval Gold Coast Queensland Australia July, 2014(2014)
摘要
Hashing techniques have been extensively investigated to boost similarity search for large-scale high-dimensional data. Most of the existing approaches formulate the their objective as a pair-wise similarity-preserving problem. In this paper, we consider the hashing problem from the perspective of optimizing a list-wise learning to rank problem and propose an approach called List-Wise supervised Hashing (LWH). In LWH, the hash functions are optimized by employing structural SVM in order to explicitly minimize the ranking loss of the whole list-wise permutations instead of merely the point-wise or pair-wise supervision. We evaluate the performance of LWH on two real-world data sets. Experimental results demonstrate that our method obtains a significant improvement over the state-of-the-art hashing approaches due to both structural large margin and list-wise ranking pursuing in a supervised manner.
更多查看译文
关键词
Hashing,Learning to rank,Structural SVM
AI 理解论文
溯源树
样例
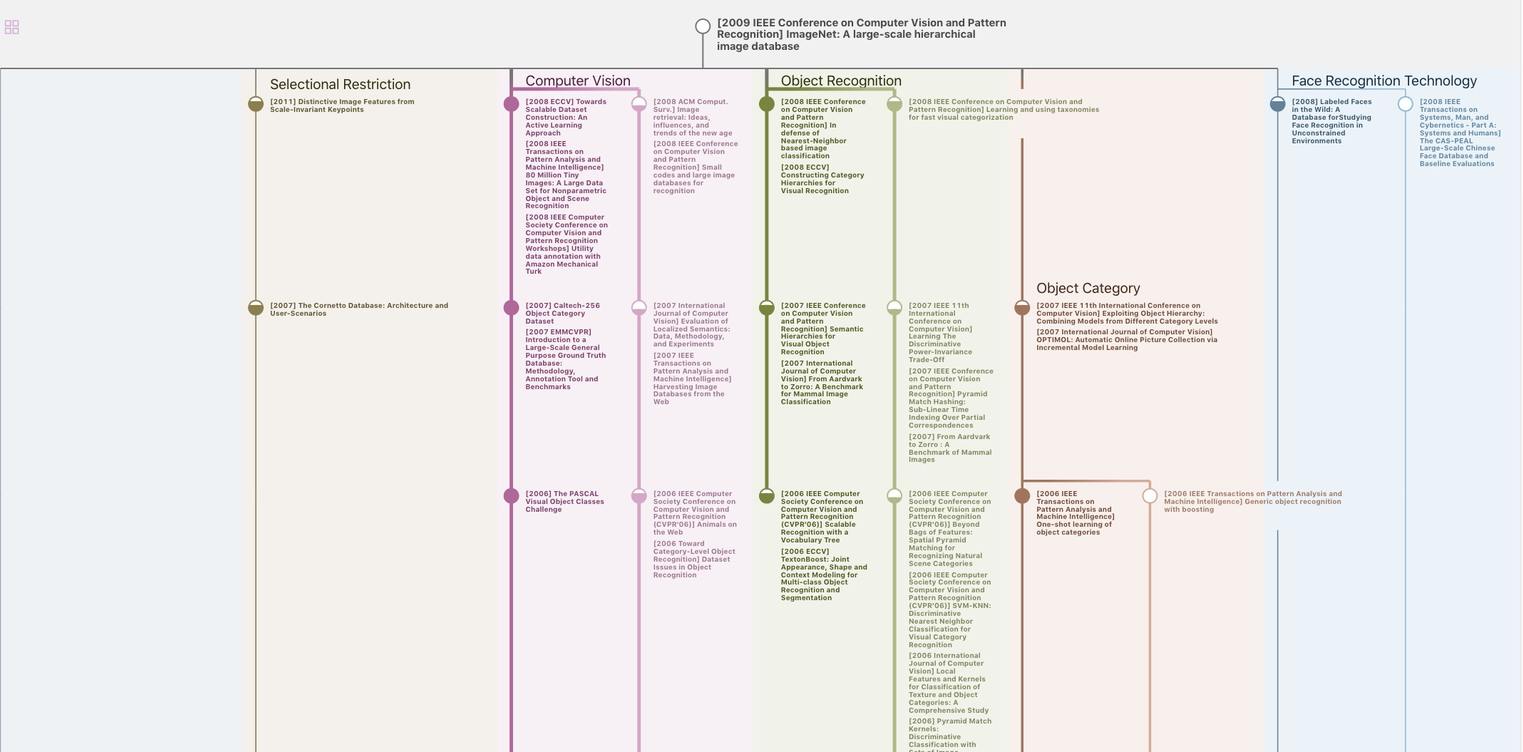
生成溯源树,研究论文发展脉络
Chat Paper
正在生成论文摘要