Influential Nodes Selection: A Data Reconstruction Perspective
SIGIR '14: The 37th International ACM SIGIR Conference on Research and Development in Information Retrieval Gold Coast Queensland Australia July, 2014(2014)
摘要
Influence maximization is the problem of finding a set of seed nodes in social network for maximizing the spread of influence. Traditionally, researchers view influence propagation as a stochastic process and formulate the influence maximization problem as a discrete optimization problem. Thus, most previous works focus on finding efficient and effective heuristic algorithms within the greedy framework. In this paper, we view the influence maximization problem from the perspective of data reconstruction and propose a novel framework named Data Reconstruction for Influence Maximization(DRIM). In our framework, we first construct an influence matrix, each row of which is the influence of a node to other nodes. Then, we select k most informative rows to reconstruct the matrix and the corresponding nodes are the seed nodes which could maximize the influence spread. Finally, we evaluate our framework on two real-world data sets, and the results show that DRIM is at least as effective as the traditional greedy algorithm.
更多查看译文
关键词
Social Networks,Influence Maximization,Data Reconstruction
AI 理解论文
溯源树
样例
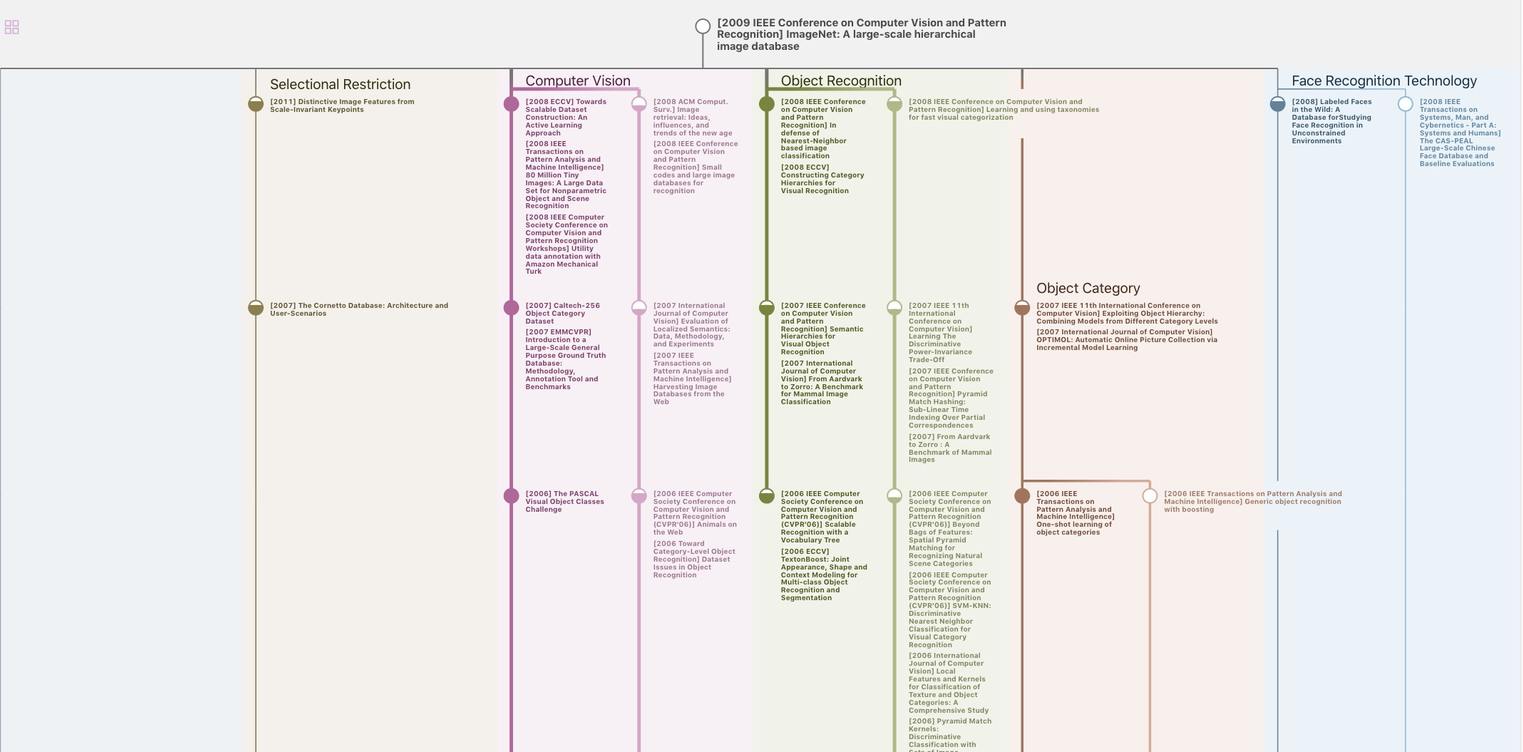
生成溯源树,研究论文发展脉络
Chat Paper
正在生成论文摘要