A Novel Context-Aware Topic Model For Category Discovery In Natural Scenes
COMPUTER VISION - ACCV 2014, PT IV(2014)
摘要
Automatic category discovery from images is a challenging problem in computer vision community especially from natural scene images due to the great variability in them. This paper proposes a novel context-aware topic model for category discovery in complex natural scenes. The proposed model constructs a generative probabilistic procedure from three-level features consisting of patch, region and the entire image by introducing latent topic variables to every patch and every region. Additionally, a new kind of scene context prior, namely, the spatial preference of categories, is also modeled using only a few parameters to reduce the ambiguity of categories in scene images. By regarding "topics" as "categories", category discovery is thus converted to the inference of the proposed probabilistic model, which will further be addressed under a Gibbs-EM framework effectively. Experimental results on two benchmark datasets comprising MSRC-v2 and SIFT Flow show its effectiveness and the advantages comparing with other methods.
更多查看译文
关键词
Visual Word, Topic Model, Latent Dirichlet Allocation, Natural Scene, Scene Context
AI 理解论文
溯源树
样例
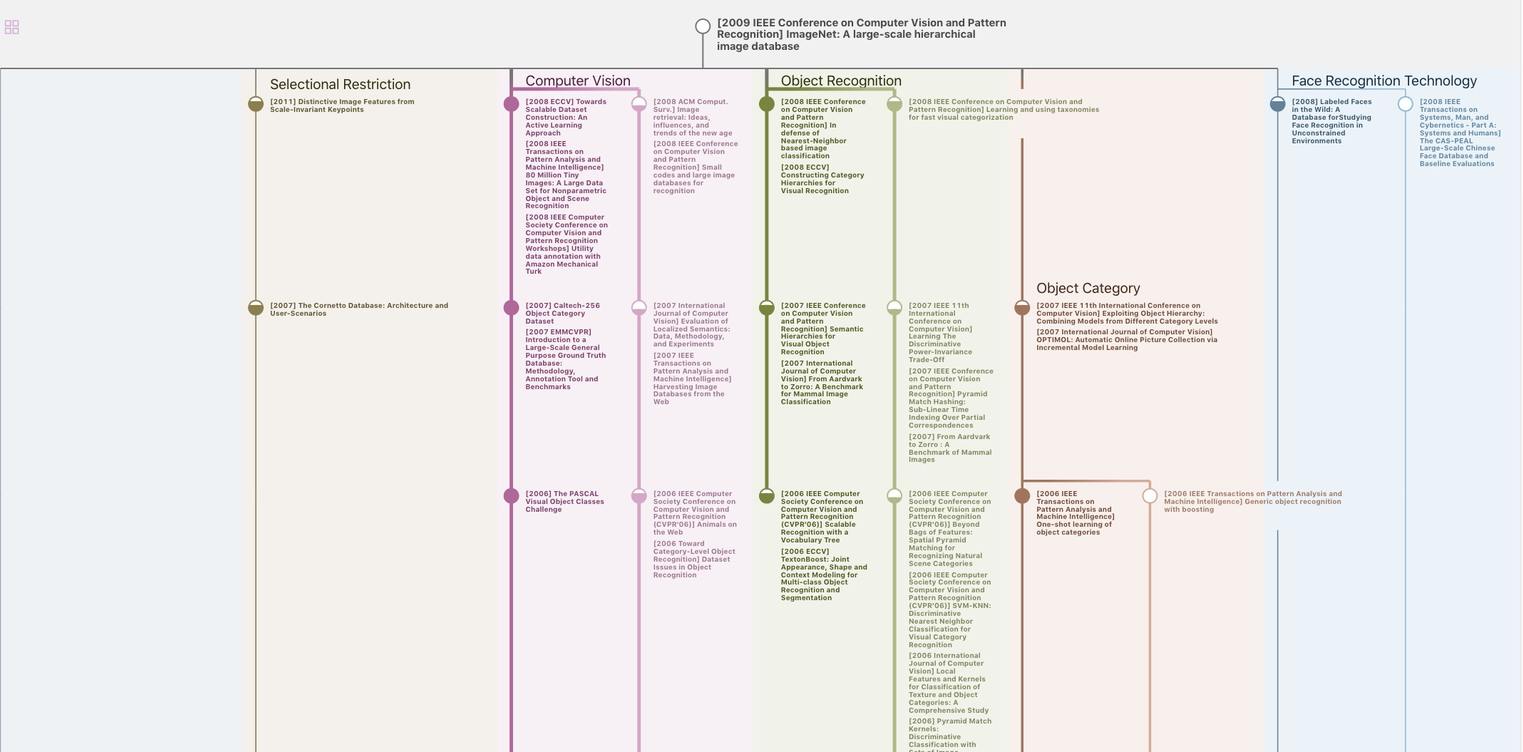
生成溯源树,研究论文发展脉络
Chat Paper
正在生成论文摘要