An Investigation On Statistical Machine Translation With Neural Language Models
CHINESE COMPUTATIONAL LINGUISTICS AND NATURAL LANGUAGE PROCESSING BASED ON NATURALLY ANNOTATED BIG DATA, CCL 2014(2014)
摘要
Recent work has shown the effectiveness of neural probabilistic language models(NPLMs) in statistical machine translation(SMT) through both reranking the n-best outputs and direct decoding. However there are still some issues remained for application of NPLMs. In this paper we further investigate through detailed experiments and extension of state-of-art NPLMs. Our experiments on large-scale datasets show that our final setting, i.e., decoding with conventional n-gram LMs plus un-normalized feedforward NPLMs extended with word clusters could significantly improve the translation performance by up to averaged 1.1 Bleu on four test datasets, while decoding time is acceptable. And results also show that current NPLMs, including feedforward and RNN still cannot simply replace n-gram LMs for SMT.
更多查看译文
关键词
statistical machine translation,neural probabilistic language model,recurrent neural network,feedforward neural network
AI 理解论文
溯源树
样例
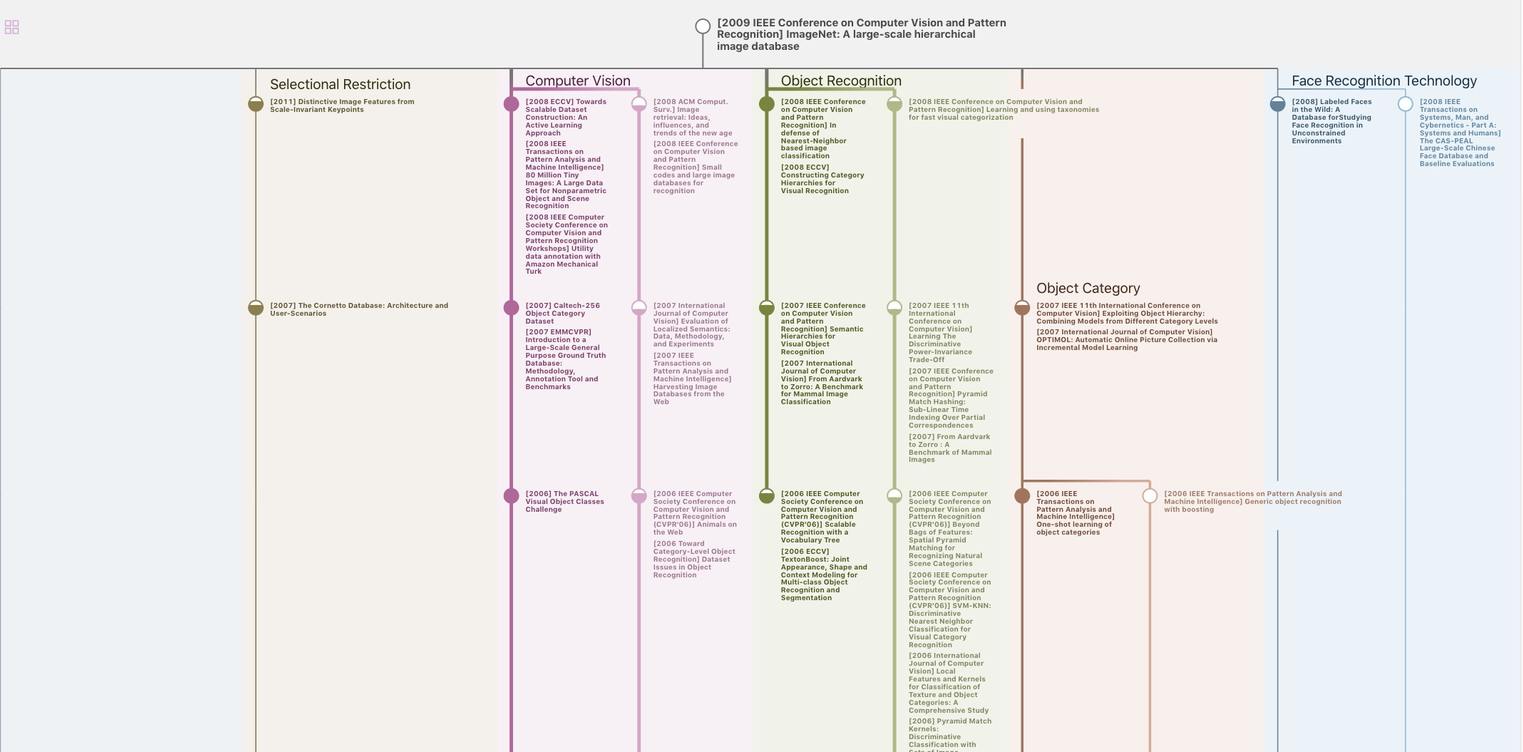
生成溯源树,研究论文发展脉络
Chat Paper
正在生成论文摘要