Multi-Scale Joint Encoding Of Local Binary Patterns For Texture And Material Classification
PROCEEDINGS OF THE BRITISH MACHINE VISION CONFERENCE 2013(2013)
摘要
In the current multi-scale LBP (MS-LBP) on texture and material classification, each scale is encoded into histograms individually. This strategy ignores the correlation between different scales, and loses a lot of discriminative information. In this paper, we propose a novel and effective multi-scale joint encoding of local binary patterns (MSJ-LBP) for texture and material classification. In MSJ-LBP, the joint encoding strategy can capture the correlation between different scales and hence depict richer local structures. In addition, the proposed MSJ-LBP is computationally simple and rotation invariant. Extensive experiments on four challenging databases (Outex_TC_00012, Brodatz, KTH-TIPS, KTH-TIPS2a) show that the proposed MSJ-LBP significantly outperforms the classical MS-LBP and achieves the state-of-the-art performance.
更多查看译文
AI 理解论文
溯源树
样例
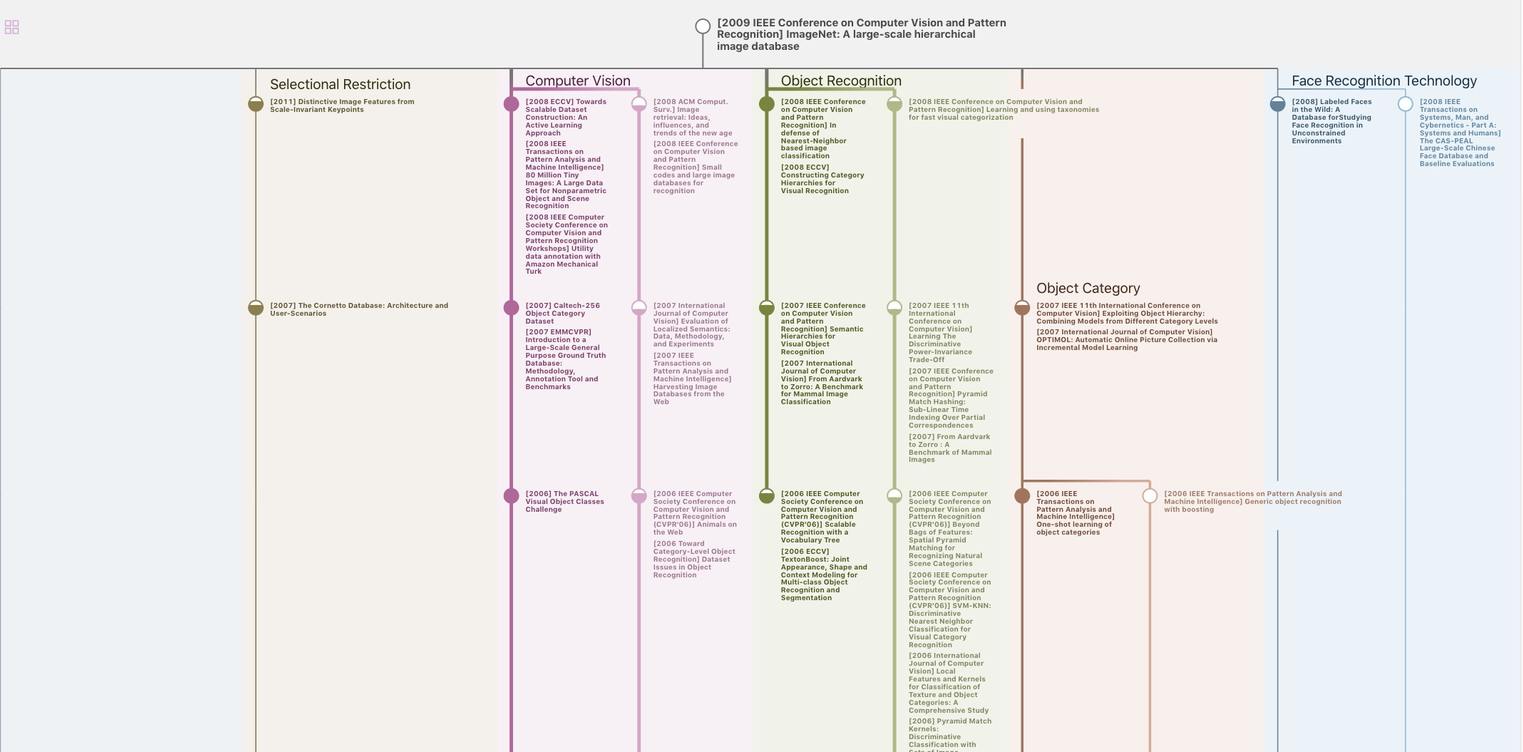
生成溯源树,研究论文发展脉络
Chat Paper
正在生成论文摘要