Bootstrap Resampling Feature Selection And Support Vector Machine For Early Detection Of Anastomosis Leakage
Biomedical and Health Informatics(2014)
摘要
We propose a Bootstrap resampling approach for Feature Selection (FS) using the weights obtained by a linear Support Vector Machine (SVM) when it is applied to high-dimensional input spaces. We build our approach on a practical application with an extremely high-dimensional input space. The application is the detection of Anastomosis Leakage (AL) after colorectal cancer surgery using free text Bag-of-Words in Electronic Health Records (EHRs). Colorectal cancer is the third most common cancer type, and surgery is the only curative treatment, making the detection of AL of prime importance. The reduced input space obtained by the proposed FS strategy in combination with the linear SVM provided a much improved performance for early detection AL after colorectal cancer (earlier/final sensitivity 97%/100% and specificity 47%/89%). Further extensions of the method can be the basis for a principled FS strategy in high-dimensional input spaces.
更多查看译文
关键词
cancer,data mining,electronic health records,sampling methods,support vector machines,EHR,anastomosis leakage detection,bootstrap resampling approach,bootstrap resampling feature selection,colorectal cancer surgery,electronic health records,free text bag-of-words,high-dimensional input space,linear SVM,principled FS strategy,support vector machine
AI 理解论文
溯源树
样例
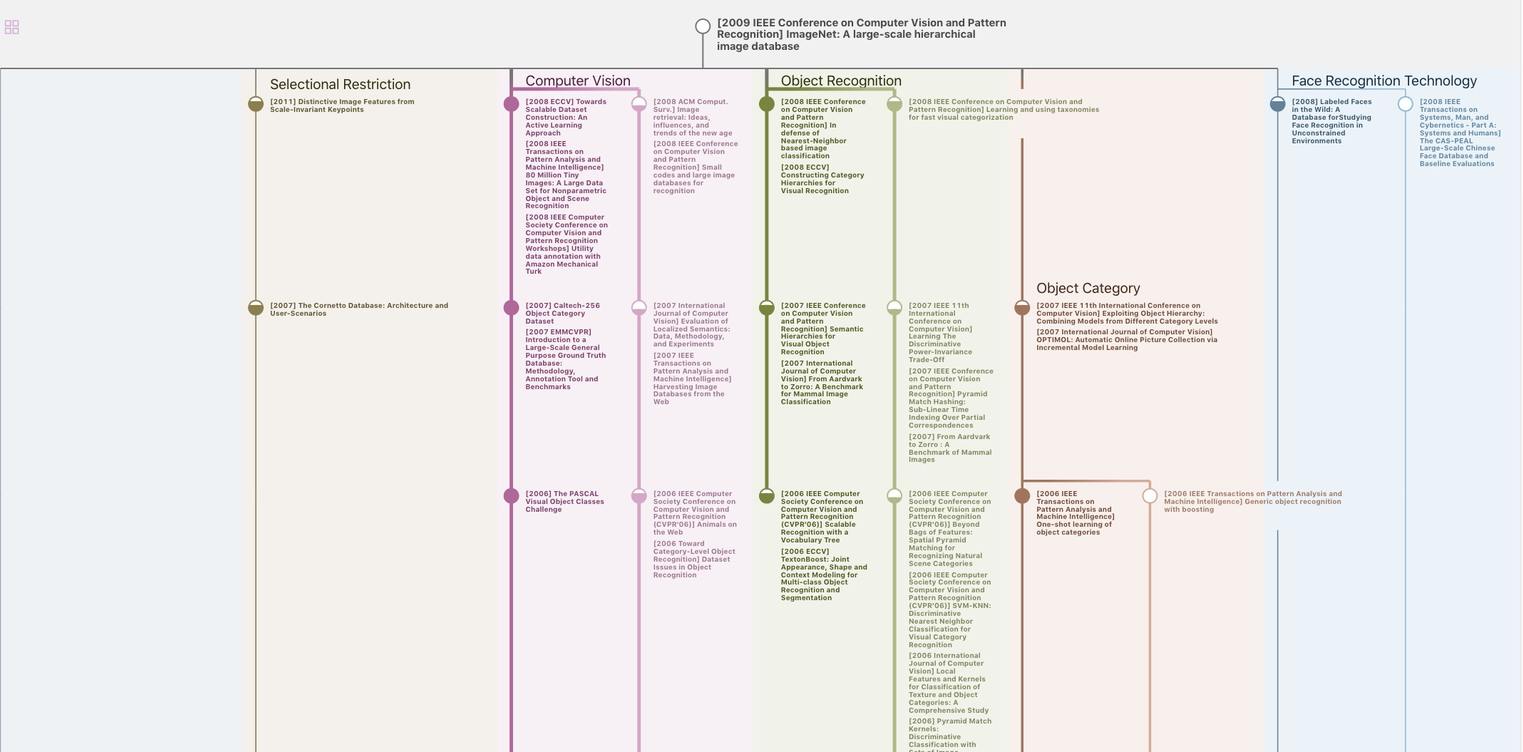
生成溯源树,研究论文发展脉络
Chat Paper
正在生成论文摘要