Memory-Efficient Tree Size Prediction For Depth-First Search In Graphical Models
PRINCIPLES AND PRACTICE OF CONSTRAINT PROGRAMMING, CP 2014(2014)
摘要
We address the problem of predicting the size of the search tree explored by Depth-First Branch and Bound (DFBnB) while solving optimization problems over graphical models. Building upon methodology introduced by Knuth and his student Chen, this paper presents a memory-efficient scheme called Retentive Stratified Sampling (RSS). Through empirical evaluation on probabilistic graphical models from various problem domains we show impressive prediction power that is far superior to recent competing schemes.
更多查看译文
关键词
Graphical Model, Problem Instance, Type System, Search Tree, Child Node
AI 理解论文
溯源树
样例
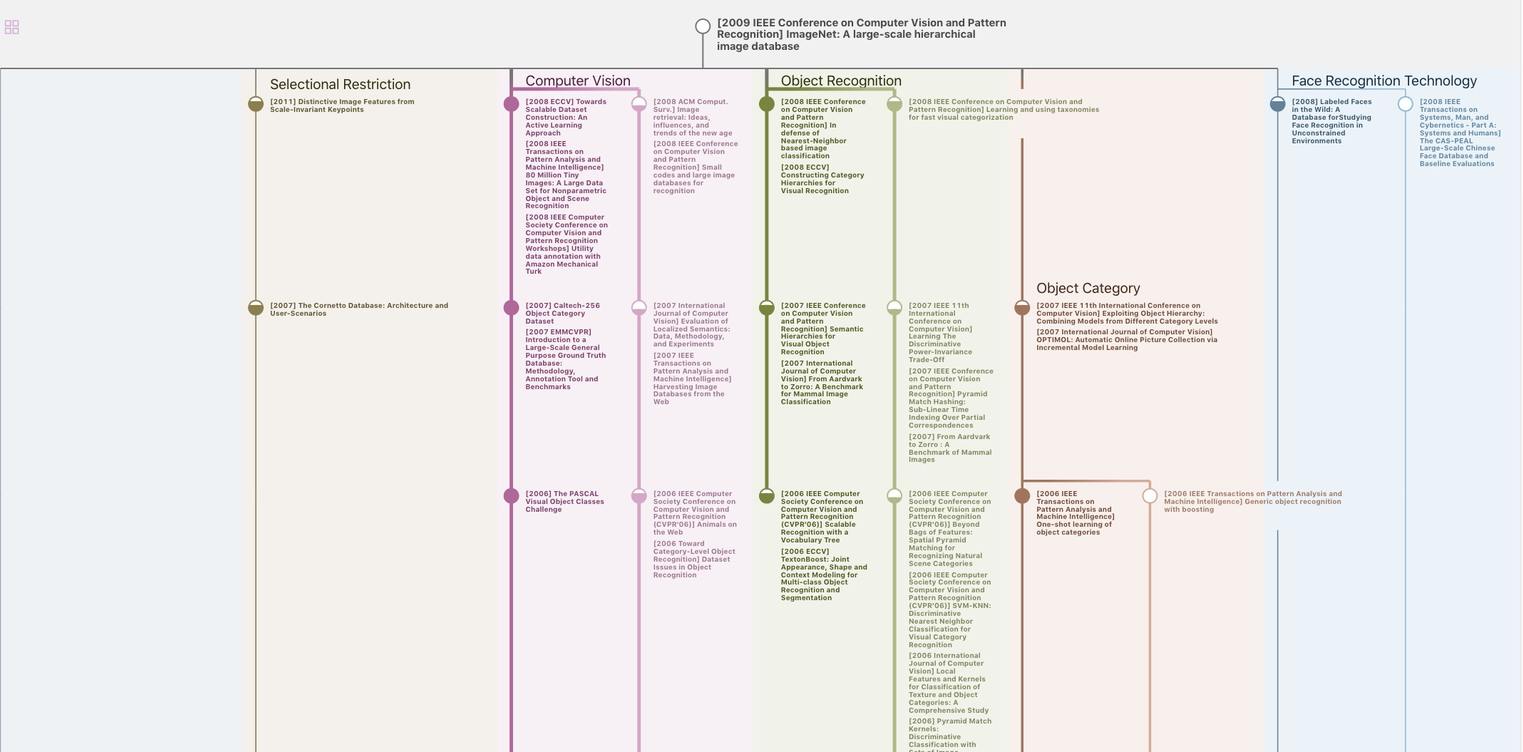
生成溯源树,研究论文发展脉络
Chat Paper
正在生成论文摘要