Recognition of deformable object category and pose
ICRA(2014)
摘要
We present a novel method for classifying and estimating the categories and poses of deformable objects, such as clothing, from a set of depth images. The framework presented here represents the recognition part of the entire pipeline of dexterous manipulation of deformable objects, which contains grasping, recognition, regrasping, placing flat, and folding. We first create an off-line simulation of the deformable objects and capture depth images from different view points as training data. Then by extracting features and applying sparse coding and dictionary learning, we build up a codebook for a set of different poses of a particular deformable object category. The whole framework contains two layers which yield a robust system that first classifies deformable objects on category level and then estimates the current pose from a group of predefined poses of a single deformable object. The system is tested on a variety of similar deformable objects and achieves a high output accuracy. By knowing the current pose of the garment, we can continue with further tasks such as regrasping and folding.
更多查看译文
关键词
off-line simulation,learning (artificial intelligence),dexterous manipulators,robust system,dictionary learning,sparse coding,pose estimation,deformable object category,feature extraction,clothing,depth images,object recognition,dexterous manipulation
AI 理解论文
溯源树
样例
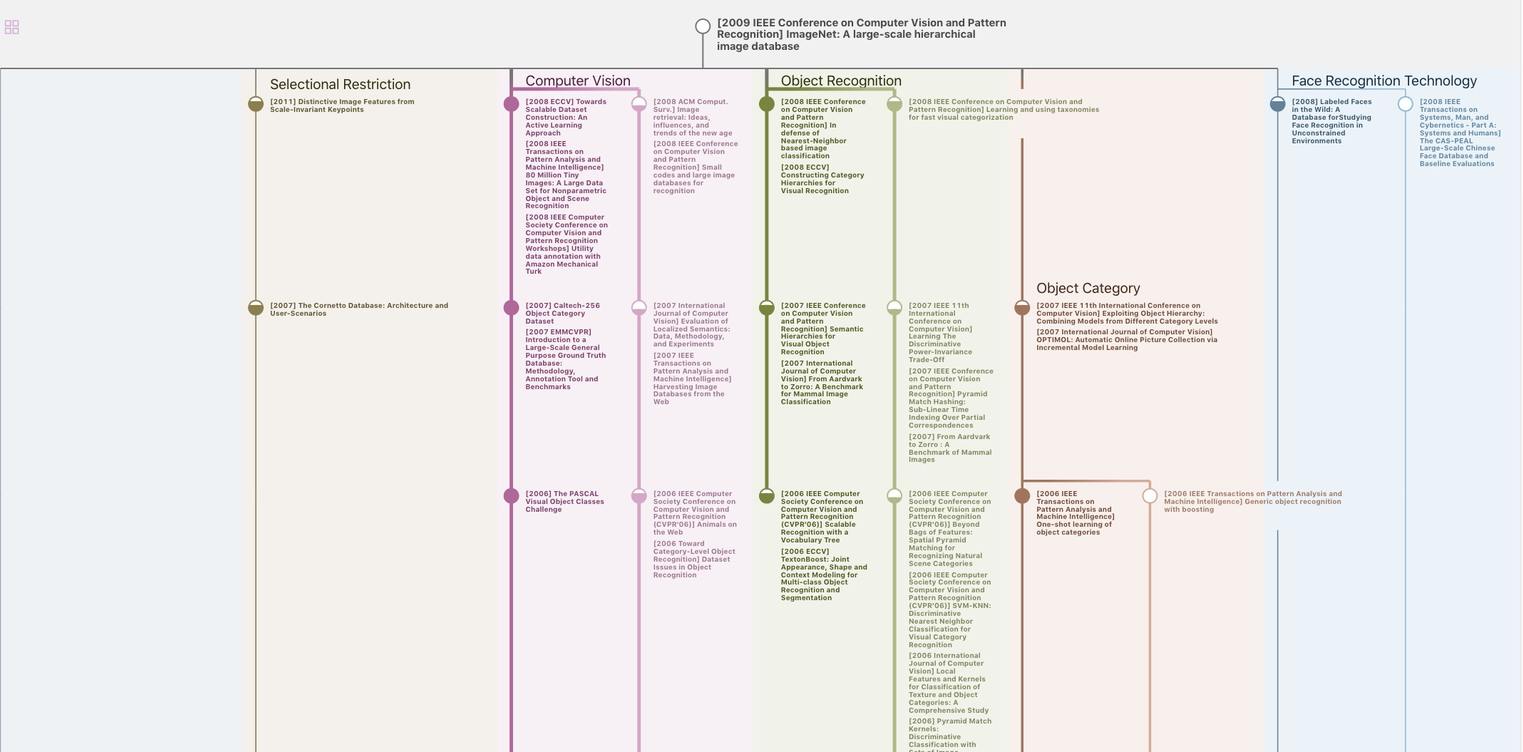
生成溯源树,研究论文发展脉络
Chat Paper
正在生成论文摘要