Clustering Spatio-Temporal Trajectories Based On Kernel Density Estimation
COMPUTATIONAL SCIENCE AND ITS APPLICATIONS - ICCSA 2014, PT 1(2014)
摘要
The data mining from spatio-temporal trajectories of moving objects has been paid much attention since the last decade and has been considered as one of the new research fields that are attracting great interest. As is known, clustering analysis is one of the most effective tools that are commonly used in data mining. Based on this, in this article, a kernel density estimation based approach is proposed towards the clustering of spatio-temporal trajectories, with the aim to investigate the spatio-temporal clustering of trajectories. In this approach, firstly, the spatio-temporal neighborhood of each trajectory unit is built. Secondly, the trajectory unit sets that are with high densities are extracted in terms of the number of trajectory units their neighborhoods contain. Thirdly, the spatio-temporal kernel density of each trajectory unit is calculated with the Gauss kernel function. What follows next is to search all the density-attracting lines from the extracted trajectory unit sets. Finally, the spatio-temporal clustering of trajectories is executed based on the density-attracting lines, each of which is regarded as the center of a cluster. Last but not least, the feasibility and efficiency of the approach is validated using a real trajectory dataset.
更多查看译文
关键词
spatio-temporal trajectories,spatio-temporal clustering,kernel density estimation,moving objects,data mining
AI 理解论文
溯源树
样例
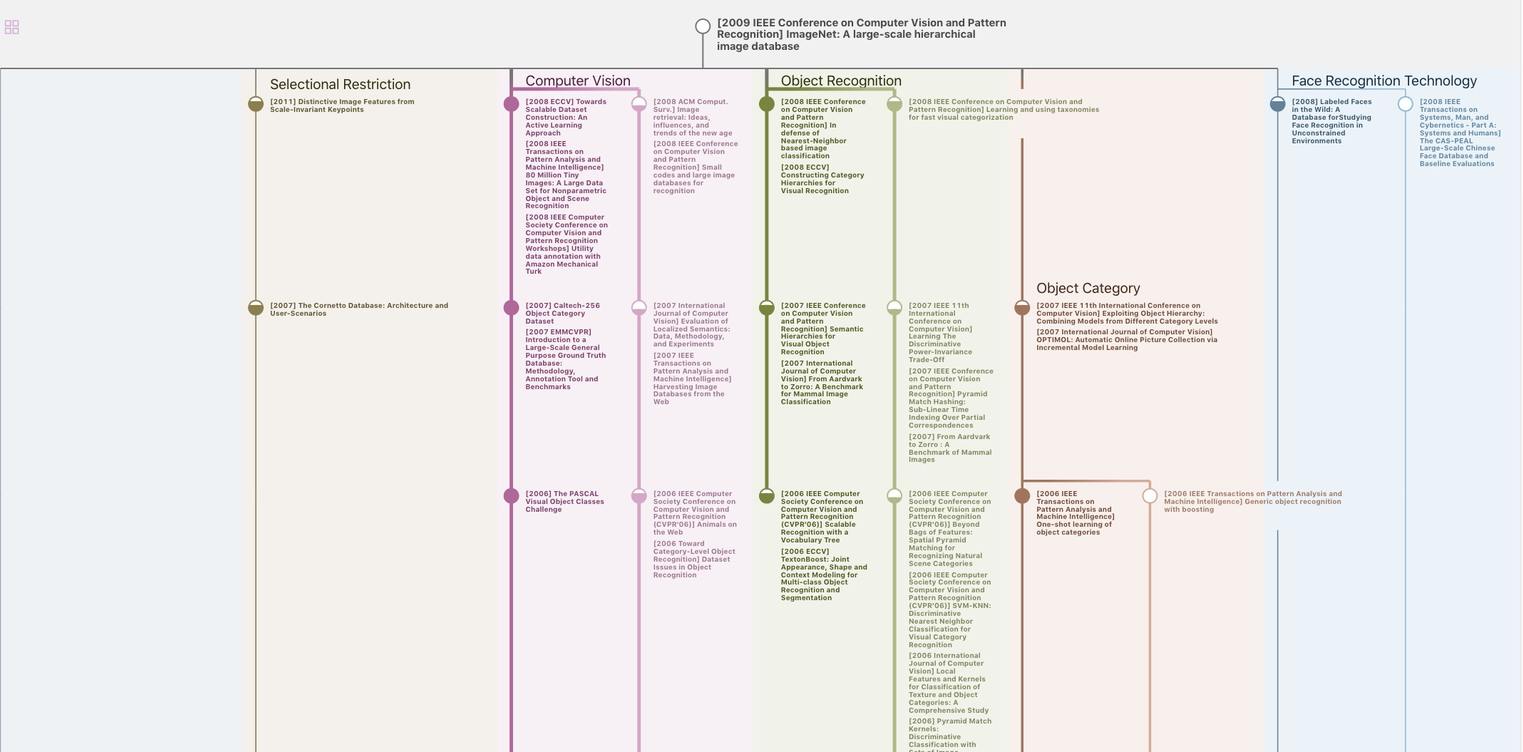
生成溯源树,研究论文发展脉络
Chat Paper
正在生成论文摘要