Up Next: Retrieval Methods For Large Scale Related Video Suggestion
KDD '14: The 20th ACM SIGKDD International Conference on Knowledge Discovery and Data Mining New York New York USA August, 2014(2014)
摘要
The explosive growth in sharing and consumption of the video content on the web creates a unique opportunity for scientific advances in video retrieval, recommendation and discovery. In this paper, we focus on the task of video suggestion, commonly found in many online applications. The current state-of-the-art video suggestion techniques are based on the collaborative filtering analysis, and suggest videos that are likely to be co-viewed with the watched video. In this paper, we propose augmenting the collaborative filtering analysis with the topical representation of the video content to suggest related videos. We propose two novel methods for topical video representation. The first method uses information retrieval heuristics such as tf-idf, while the second method learns the optimal topical representations based on the implicit user feedback available in the online scenario. We conduct a large scale live experiment on YouTube traffic, and demonstrate that augmenting collaborative filtering with topical representations significantly improves the quality of the related video suggestions in a live setting, especially for categories with fresh and topically-rich video content such as news videos. In addition, we show that employing user feedback for learning the optimal topical video representations can increase the user engagement by more than 80% over the standard information retrieval representation, when compared to the collaborative filtering baseline.
更多查看译文
关键词
Video retrieval,related video suggestion,video representation
AI 理解论文
溯源树
样例
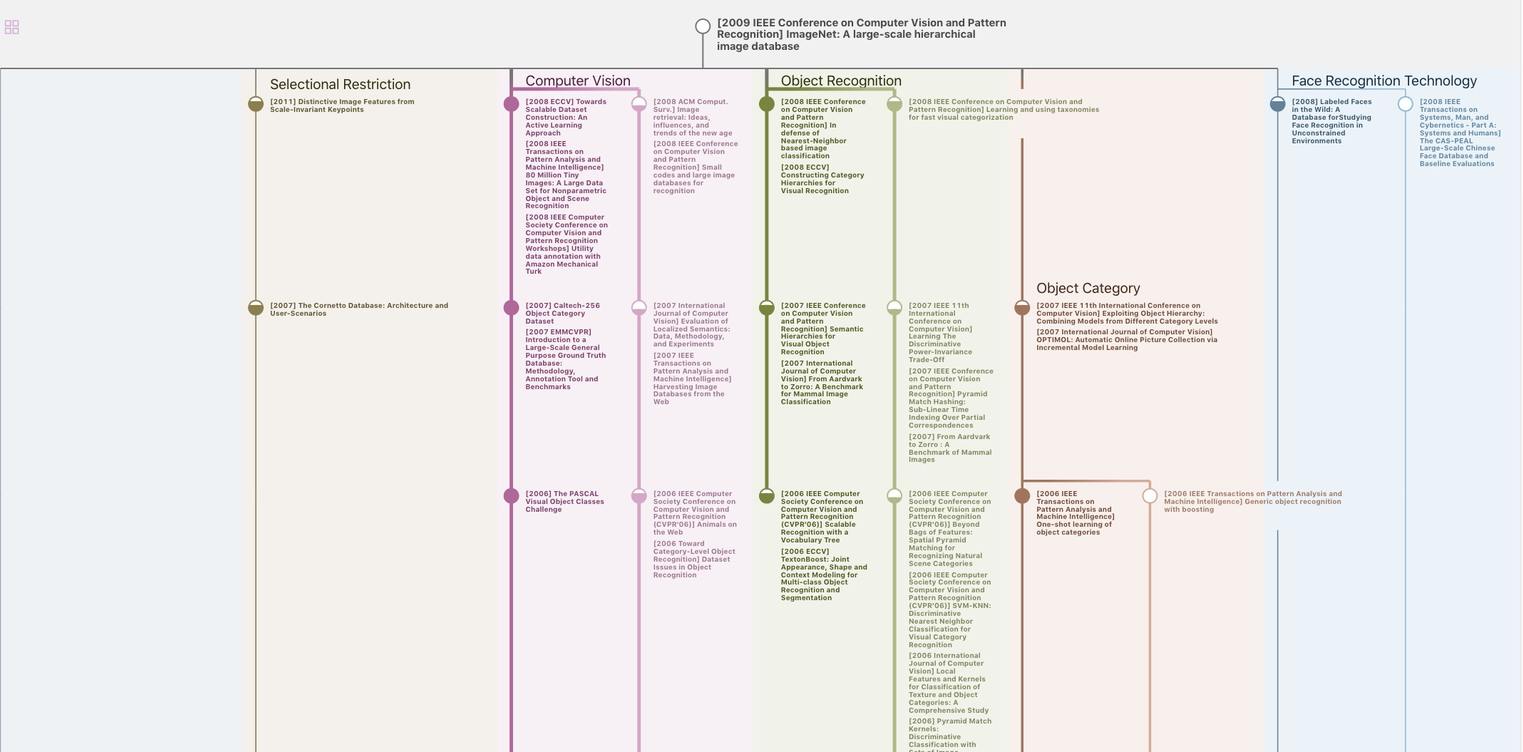
生成溯源树,研究论文发展脉络
Chat Paper
正在生成论文摘要