Using mobile phones to simulate pulse oximeters: gait analysis predicts oxygen saturation.
BCB(2014)
摘要
ABSTRACTWidespread availability of mobile devices is leading revolution in health monitoring. Smartphones have demonstrated strong potential as ubiquitous monitors, but it is still unclear what vital signs can be monitored. We present a novel machine learning model to track oxygen saturation from phone sensors. Oxygen saturation is perhaps the major composite vital sign, widely used as a single measure of health status during medical procedures. We compute spatio-temporal gait parameters from phone sensor data, then use these to predict oxygen saturation with a trained model. We compare alternative approaches in each step of model training to discover optimal solutions. The validation shows training models on cohorts performs better than training a universal model. We ran unconstrained 6 minute walk tests on 15 senior pulmonary patients to predict oxygen saturation. The absolute error rate is about 1% for the cohort models and about 2% for the universal model. This can be compared to the 1% resolution of the pulse oximeter serving as standard and the 4% drop in values signifying desaturation. We also find that characteristic patterns in oxygen saturation as predicted correlate closely with the pulmonary function status. Thus smart phones have great potential to monitor desaturation in chronic patients.
更多查看译文
AI 理解论文
溯源树
样例
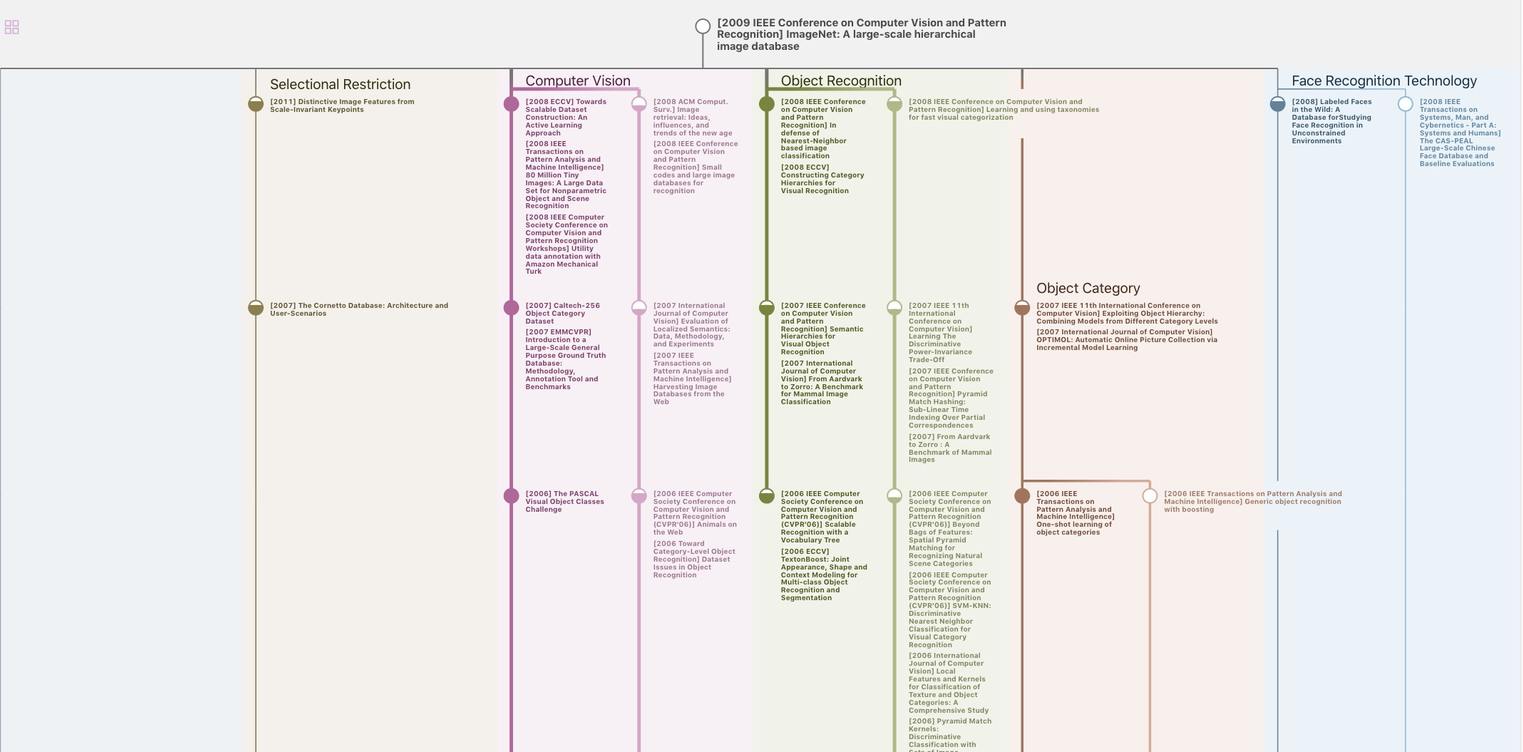
生成溯源树,研究论文发展脉络
Chat Paper
正在生成论文摘要