Dose and time relationship through probabilistic graphical models of gene expression time course toxicogenomics data.
BCB(2014)
摘要
ABSTRACTBackground A probabilistic graphical model is a representation of searched properties of random variables represented by nodes. The edges in the graph represent conditional independence properties used to obtain a number of valid factorizations of the joint probability distribution. There are different types of graphical models, Bayesian networks for instance, are directed graphical models but Markov random fields are undirected models. Dynamic Bayesian networks (DBN) are Bayesian networks that model time series where edges have a direction and point in the direction of time. The directed edge in a BN can encode either a random or a deterministic relationship between the two variables. The structure of a graphical model represents the way in which a set of random variables probabilistically represents natural and systematic processes such as noisy measurements of the observed gene expression levels at each time point, and how these variables interact in a form of a gene regulatory network. Motivation The liver is one of the organs involved in biotransformation, chemical reactions that alter the structure, aqueous solubility and eventual disposition of non- nutritive compounds that enter into the organism. Xenobiotic biotransformation aims at controlling the toxication or detoxication of xenobiotic substances. However, during the biotransformation reactive intermediates may be produced, these could interact with critical cellular macromolecules and trigger the events that promote either tissue injury and cell death, permanent genomic changes, leading potentially to cancer[4]. The liver decomposes some of the drugs that can be acquired without prescription. These drugs may cause hepatic damage if the liver is exposed to them in high doses or the intake is continuous. The process of drug decomposition in the liver varies and is slower in some people, this way the next intake of the drug may happen before the last one is successfully thrown away. Drugs that contain acetaminophen, such as painkillers, are one of the most frequent causes of liver inflammation. The continuous inflammation of this organ caused by low doses of these drugs may trigger a greater damage than ingest higher doses in short time lapses[6]. To understand how drugs work it is important to analyze how they interact with some of the genes and how these interact among them as well. By knowing how the genes interact with the drug and its dose, it is possible to infer how toxic the drug is. Also, it is important the time the drug is active and the time that it stays in the body. If the interactions of the drug with the body are understood, the prescriptions can be done more carefully. Data We used gene expression data from the Japanese toxicogenomics project (TGP)[5], a 5-year project that was completed in 2007 with a collection of 17,657 AffymetrixTM microarrays from both in vitro human and rat samples. The data was delimited to only in vivo samples, these were analyzed using the GeneChip Rat Genome 230 2.0 which is known to be a powerful tool for toxicology[1]. A total of 131 compounds were studied at 4 different dosage levels: None, low, medium and high, the first one was used as control. (Figure 1) The in-vivo data has the larger number of time points where measurements across time were a one day study with a single dosage with 4 time points (3, 6, 9, 24 hrs) then a 29-days study with a daily dose also with 4 time points (4, 8, 15, 29 days). Each of these has 3 replicates with a total of 24 microarrays per compound. Data were pre-processed and genes ranked according to a multi-Bayes statistic implemented using the timecourse tool by Chuan and Speed[3]. Compounds were analyzed and some were selected by their toxicity. Model description To analyze the drug interactions over time we decided to use DBNs, as they help in the understanding of some of the underlying interactions among the variables represented by gene expressions. We also explored other models that are used to understand interactions that occur along a time lapse, such as the Hidden Markov Model (HMM). The advantage of using HMMs is the capability of having unobserved states or latent variables that can be inferred by other observed variables[2]. The transitions between states are determined by a transition matrix and Bayesian conditional probability; therefore, the next state is determined by the most probable one. One method to profile the genes behavior is to cluster them according to their travel path over states, this is called profile HMM. By profiling the best ranking genes given their dose and compound the toxicity of this couple can be inferred. If in different compounds with similar chemical structure the genes have the same profiles, then the toxicity of drugs that share similar structure could be inferred.
更多查看译文
AI 理解论文
溯源树
样例
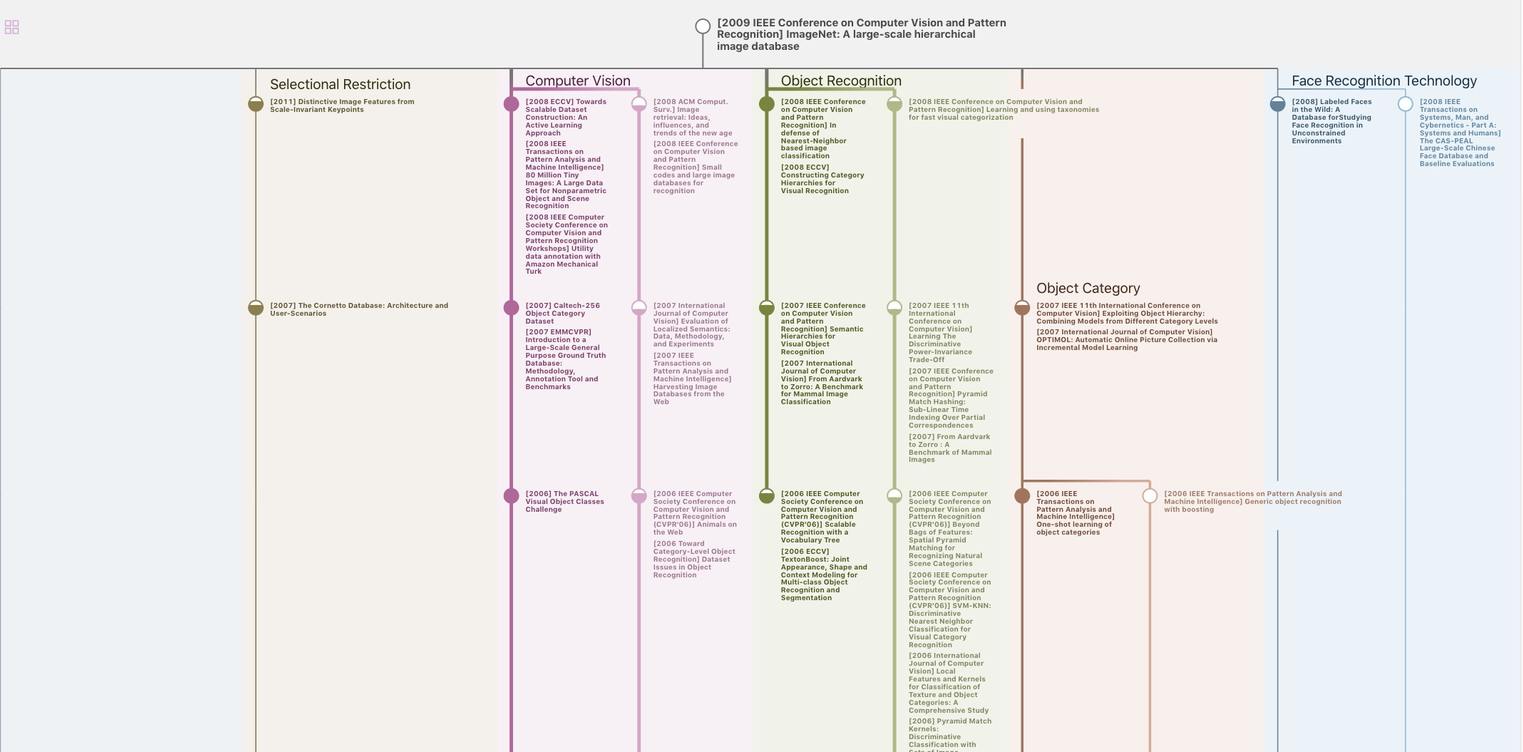
生成溯源树,研究论文发展脉络
Chat Paper
正在生成论文摘要