Target setting with consideration of target-induced operation variability for performance improvement of semiconductor fabrication
CASE(2014)
摘要
Production target setting is common in practice to guide operations such as machine allocation and lot dispatching to achieve master production schedule (MPS). As targets affect operations and hence wafer flows, wafer flow estimation under given daily production targets is a basis of adjusting targets and machine allocation. This paper presents an innovative design of target setting algorithm (TaSIV) that develops the target-tracking service model by characterizing target-induced mean and variability and designs a hybrid flow time approximation by exploiting transient tendon queue analysis between two stages to set targets for improving production performance. The design first adopts a Bernoulli trial with proportional-to-target probability to model the target-tracking machine allocation and FIFO dispatching and then characterize the target-induced variability (TIV). To capture the effect of TIV on wafer flows, the design then approximates the time for the last wafer in initial WIP of a stage to finish processing at the next stage, named two-stage penetration time approximation, APT-2, by using Markov chain analysis of tandem queues with given initial number of wafers. By Integrating APT-2 into a recursive algorithm, SOPEA, the design estimates penetration time of multiple stages and wafer flows in a fab. Finally, our design integrates APT-2/SOPEA into a fixed-point iteration between wafer flow estimation and capacity allocation for target setting with consideration of TIV. Over a mini-Fab example and given targets generated by TaSIV, simulation of proportional-to-target machine allocation and FIFO dispatching demonstrates that targets generated by TaSIV reduces over-optimism and close to actual moves by 30.7% of bottleneck machine groups as compared to a mean-value based scheme frequently adopted by practitioners of fab operation management. TaSIV also leads to reductions of 1.2% in mean cycle time and 15.4% in cycle time variance at 1.1% throughput increase.
更多查看译文
关键词
fifo dispatching,target-tracking service model,daily target setting,target-tracking machine allocation,bernoulli trial,capacity allocation,proportional-to-target probability,fixed-point iteration,semiconductor fabrication,transient tendon queue analysis,performance improvement,daily wafer flow estimation,water,recursive algorithm,hybrid flow time approximation,wafer flow estimation,sopea,target setting,machine allocation variability,two-stage penetration time approximation,apt-2,markov chain analysis,markov processes,target-induced operation variability,semiconductor industry,iterative methods,algorithm design and analysis,resource management,production,estimation
AI 理解论文
溯源树
样例
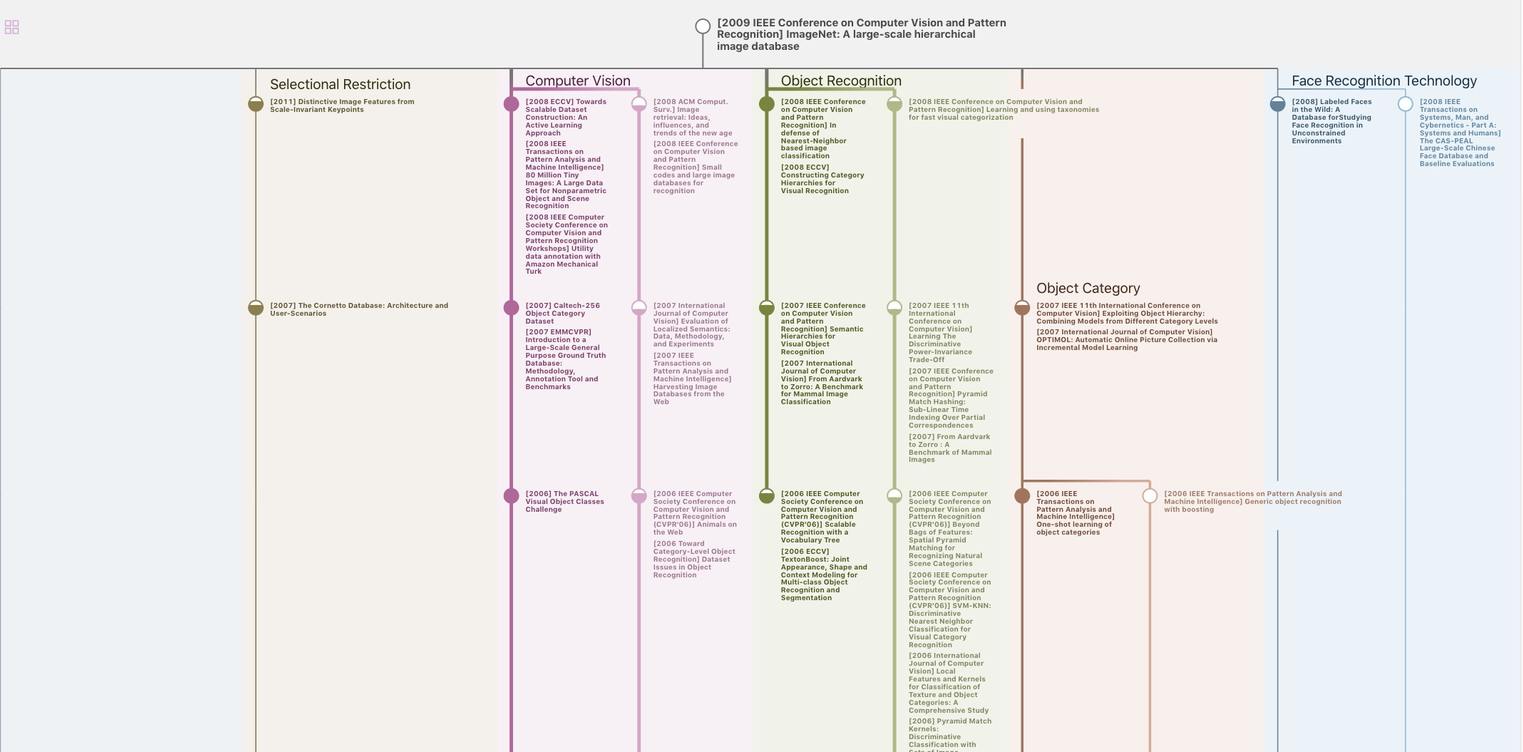
生成溯源树,研究论文发展脉络
Chat Paper
正在生成论文摘要