Online Evolution of Deep Convolutional Network for Vision-Based Reinforcement Learning.
Lecture Notes in Artificial Intelligence(2014)
摘要
Dealing with high-dimensional input spaces, like visual input, is a challenging task for reinforcement learning (RL). Neuroevolution (NE), used for continuous RL problems, has to either reduce the problem dimensionality by (1) compressing the representation of the neural network controllers or (2) employing a pre-processor (compressor) that transforms the high-dimensional raw inputs into low-dimensional features. In this paper we extend the approach in [16]. The Max-Pooling Convolutional Neural Network (MPCNN) compressor is evolved online, maximizing the distances between normalized feature vectors computed from the images collected by the recurrent neural network (RNN) controllers during their evaluation in the environment. These two interleaved evolutionary searches are used to find MPCNN compressors and RNN controllers that drive a race car in the TORCS racing simulator using only visual input.
更多查看译文
关键词
deep learning,neuroevolution,vision-based TORCS,reinforcement learning,computer games
AI 理解论文
溯源树
样例
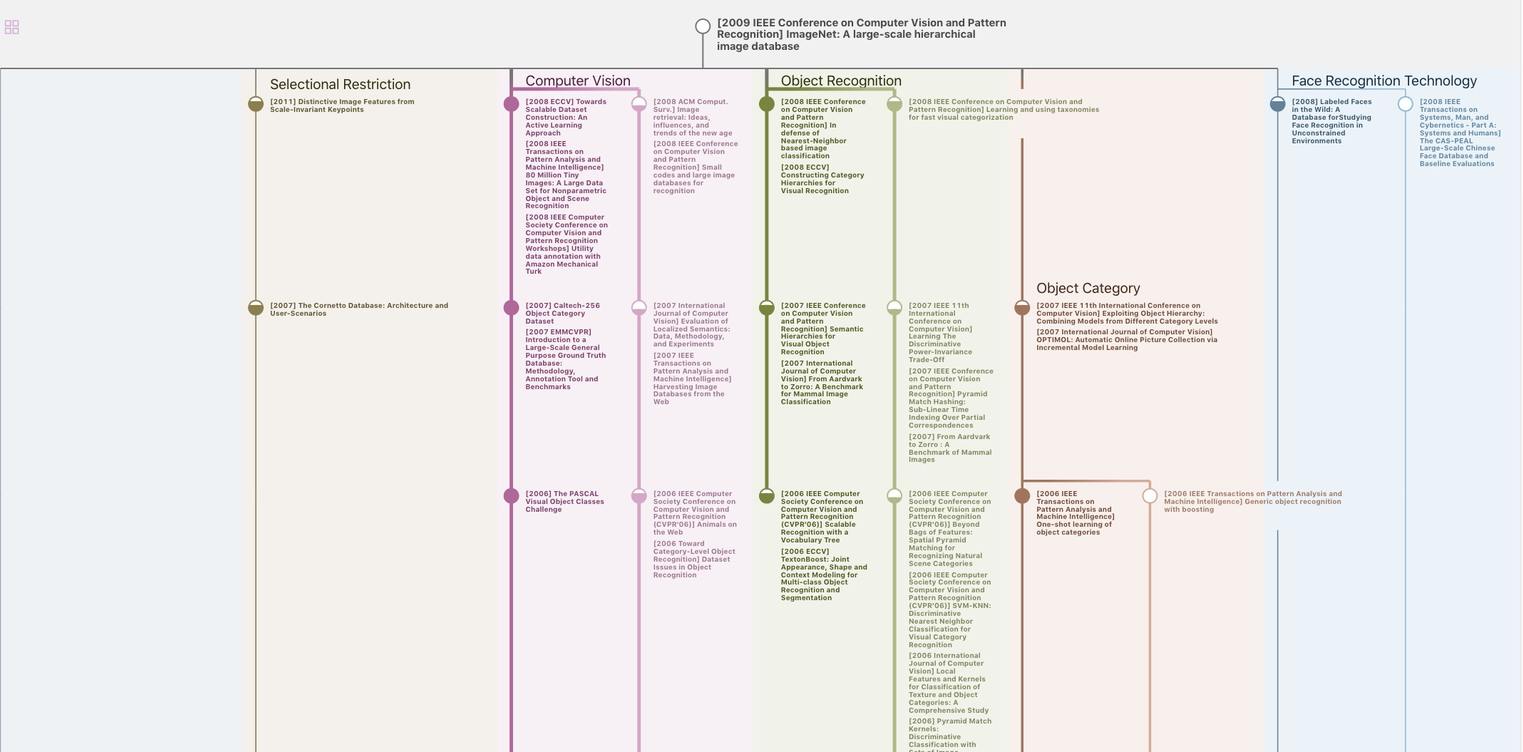
生成溯源树,研究论文发展脉络
Chat Paper
正在生成论文摘要