State-of-charge estimation for batteries: A multi-model approach
ACC(2014)
摘要
Monitoring the state-of-charge (SoC) for batteries is challenging, especially when a battery has time-varying parameters. We propose to improve SoC estimation using an adaptive strategy and multiple models in this study, developing a unique algorithm called MM-AdaSoC. Specifically, two submodels in state-space form are generated from a modified Nernst battery model. Both are shown to be locally observable under mild conditions. The iterated extended Kalman filter (IEKF) is then applied to each submodel in parallel, estimating simultaneously the SoC variable and certain unknown parameters. The SoC estimates obtained from the two separately implemented IEKFs are fused to yield the final overall SoC estimates, which tend to have higher accuracy than those obtained from a single-model. Its effectiveness is demonstrated via experiments.
更多查看译文
关键词
estimation,state-of-charge estimation,kalman filters,multimodel approach,battery management systems,time-varying systems,soc estimation,time-varying parameters,nernst battery model,thermomagnetic effects,batteries,mm-adasoc,iekf,emerging control applications,iterated extended kalman filter,kalman filtering,nonlinear filters,system on chip,observability,mathematical model
AI 理解论文
溯源树
样例
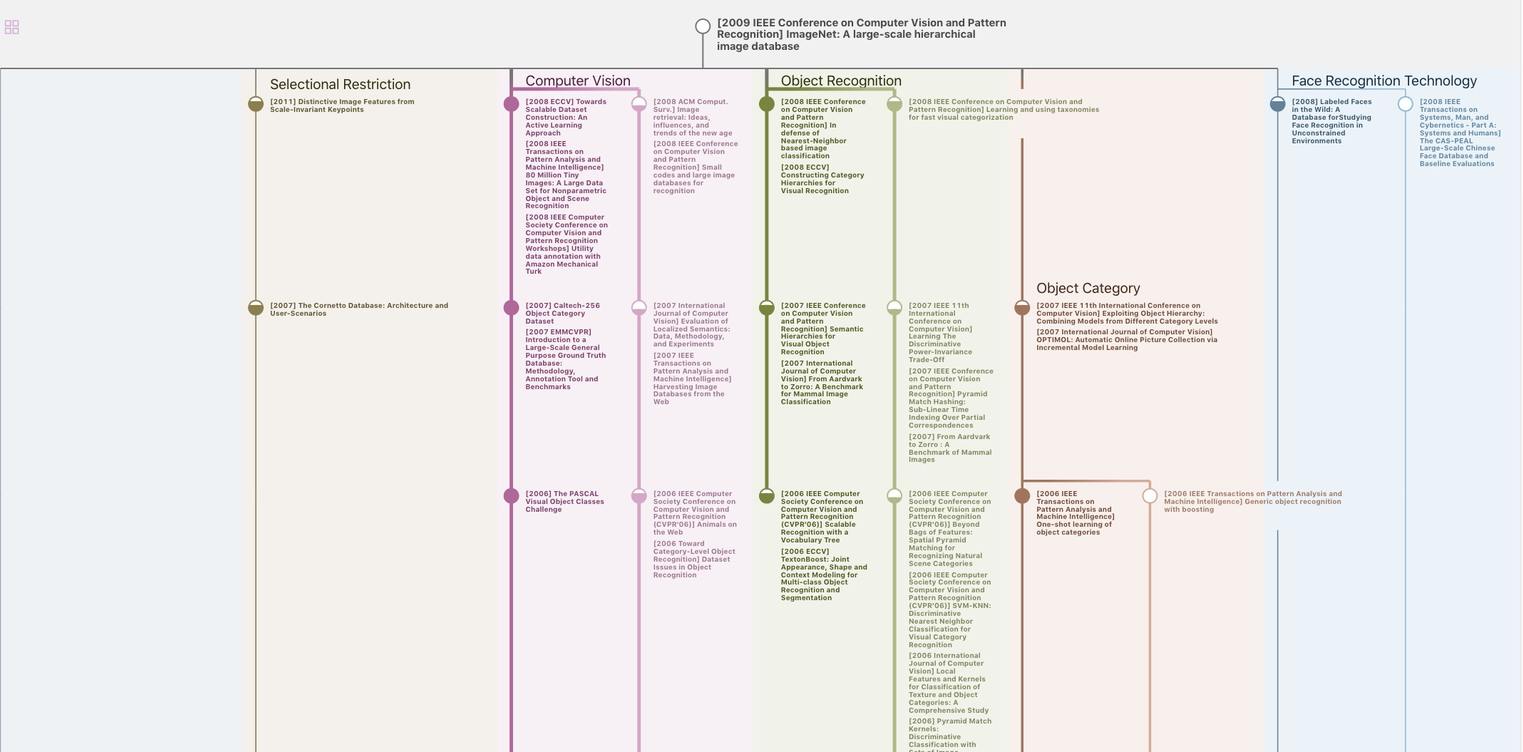
生成溯源树,研究论文发展脉络
Chat Paper
正在生成论文摘要