A state-space approach to modeling functional time series application to rail supervision
Signal Processing Conference(2014)
摘要
This article introduces a state-space model for the dynamic modeling of curve sequences within the framework of railway switches online monitoring. In this context, each curve has the peculiarity of being subject to multiple changes in regime. The proposed model consists of a specific latent variable regression model whose coefficients are supposed to evolve dynamically in the course of time. Its parameters are recursively estimated across a sequence of curves through an online Expectation-Maximization (EM) algorithm. The experimental study conducted on two real power consumption curve sequences from the French high speed network has shown encouraging results.
更多查看译文
关键词
condition monitoring,expectation-maximisation algorithm,power consumption,railways,recursive estimation,regression analysis,state-space methods,switches,time series,French high speed network,curve sequence dynamic modeling,functional time series modeling application,online EM algorithm,online expectation maximization algorithm,power consumption curve sequence,rail supervision,railway switches online monitoring,recursive estimation,specific latent variable regression model,state-space model,Kalman filtering,Time series of functional data,condition monitoring,online Expectation-Maximization (EM) algorithm,state-space model
AI 理解论文
溯源树
样例
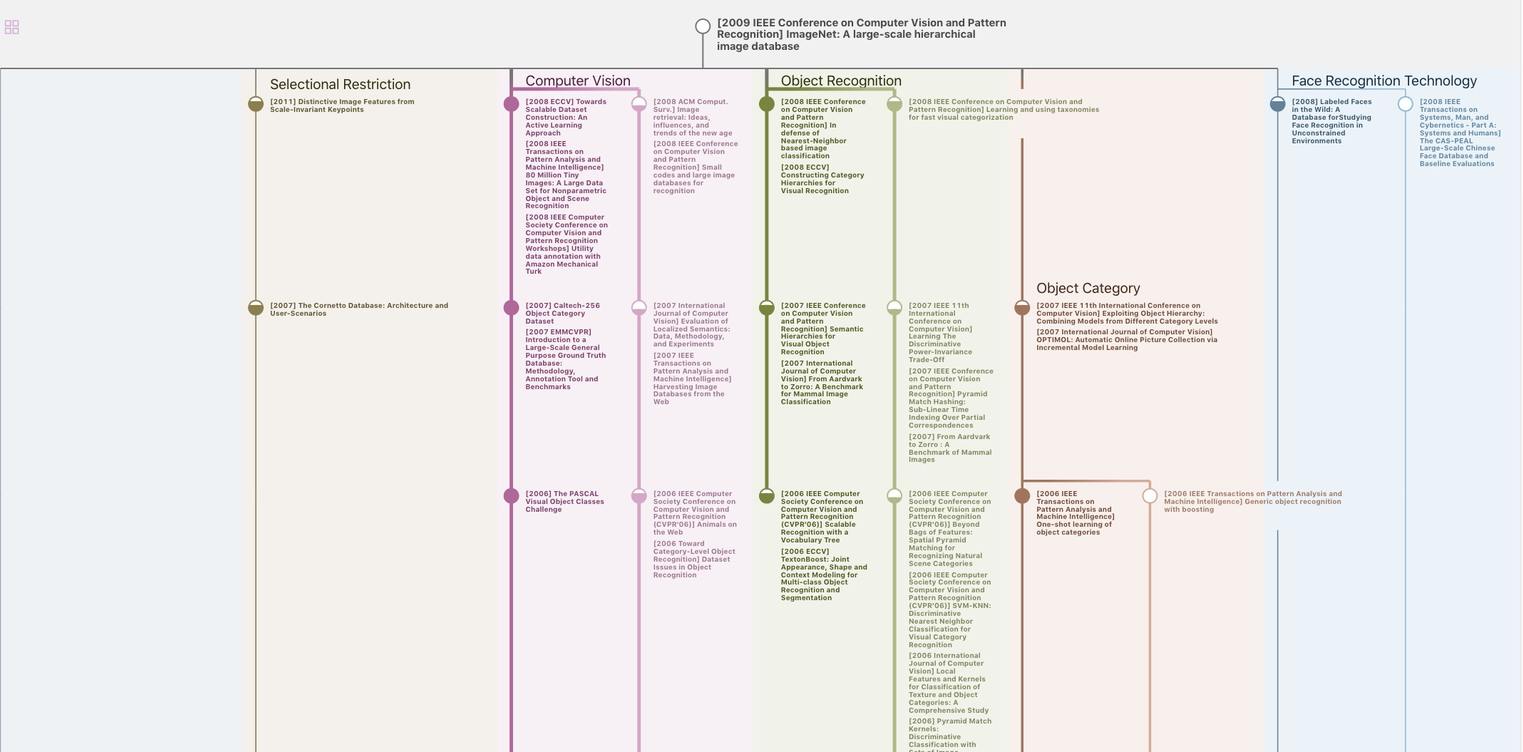
生成溯源树,研究论文发展脉络
Chat Paper
正在生成论文摘要