Leveraging Machine Learning to Improve Unwanted Resource Filtering.
CCS(2014)
摘要
ABSTRACTAdvertisements simultaneously provide both economic support for most free web content and one of the largest annoyances to end users. Furthermore, the modern advertisement ecosystem is rife with tracking methods which violate user privacy. A natural reaction is for users to install ad blockers which prevent advertisers from tracking users or displaying ads. Traditional ad blocking software relies upon hand-crafted filter expressions to generate large, unwieldy regular expressions matched against resources being included within web pages. This process requires a large amount of human overhead and is susceptible to inferior filter generation. We propose an alternate approach which leverages machine learning to bootstrap a superior classifier for ad blocking with less human intervention. We show that our classifier can simultaneously maintain an accuracy similar to the hand-crafted filters while also blocking new ads which would otherwise necessitate further human intervention in the form of additional handmade filter rules.
更多查看译文
AI 理解论文
溯源树
样例
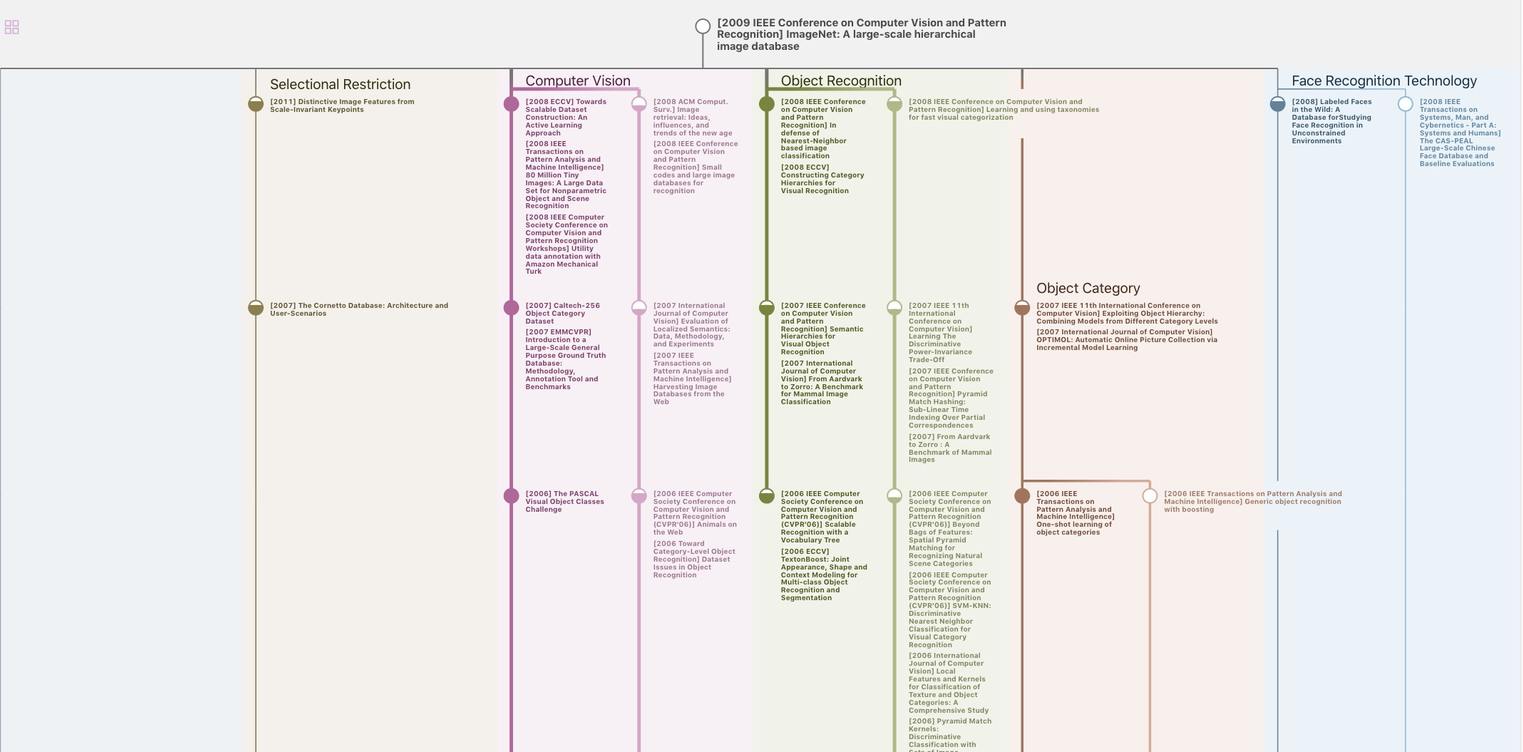
生成溯源树,研究论文发展脉络
Chat Paper
正在生成论文摘要