Adversarial Active Learning
AISec@CCS(2014)
摘要
Active learning is an area of machine learning examining strategies for allocation of finite resources, particularly human labeling efforts and to an extent feature extraction, in situations where available data exceeds available resources. In this open problem paper, we motivate the necessity of active learning in the security domain, identify problems caused by the application of present active learning techniques in adversarial settings, and propose a framework for experimentation and implementation of active learning systems in adversarial contexts. More than other contexts, adversarial contexts particularly need active learning as ongoing attempts to evade and confuse classifiers necessitate constant generation of labels for new content to keep pace with adversarial activity. Just as traditional machine learning algorithms are vulnerable to adversarial manipulation, we discuss assumptions specific to active learning that introduce additional vulnerabilities, as well as present vulnerabilities that are amplified in the active learning setting. Lastly, we present a software architecture, Security-oriented Active Learning Testbed (SALT), for the research and implementation of active learning applications in adversarial contexts.
更多查看译文
关键词
security and protection,user/machine systems,human in the loop,active learning,secure machine learning,learning
AI 理解论文
溯源树
样例
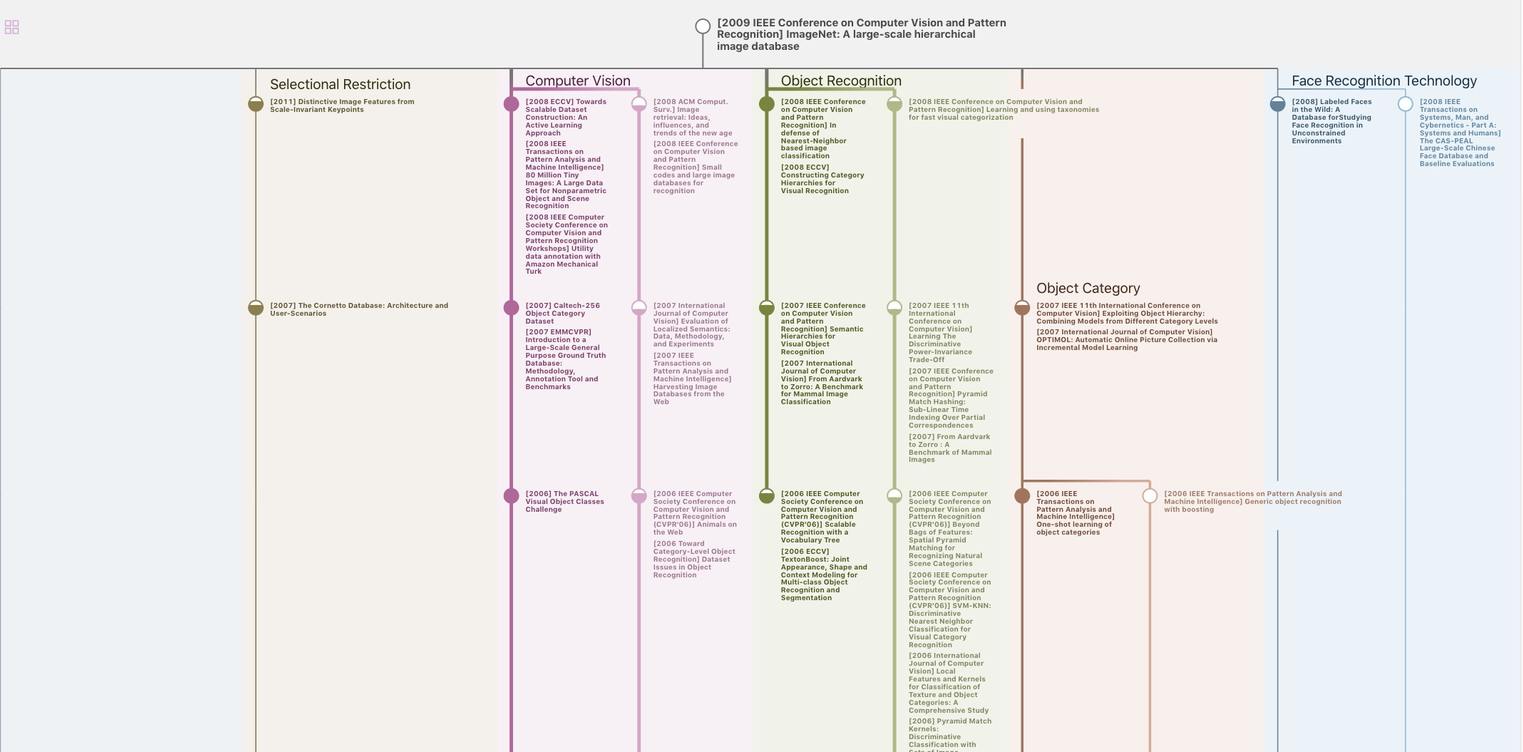
生成溯源树,研究论文发展脉络
Chat Paper
正在生成论文摘要