Articulatory features from deep neural networks and their role in speech recognition
Acoustics, Speech and Signal Processing(2014)
摘要
This paper presents a deep neural network (DNN) to extract articulatory information from the speech signal and explores different ways to use such information in a continuous speech recognition task. The DNN was trained to estimate articulatory trajectories from input speech, where the training data is a corpus of synthetic English words generated by the Haskins Laboratories' task-dynamic model of speech production. Speech parameterized as cepstral features were used to train the DNN, where we explored different cepstral features to observe their role in the accuracy of articulatory trajectory estimation. The best feature was used to train the final DNN system, where the system was used to predict articulatory trajectories for the training and testing set of Aurora-4, the noisy Wall Street Journal (WSJ0) corpus. This study also explored the use of hidden variables in the DNN pipeline as a potential acoustic feature candidate for speech recognition and the results were encouraging. Word recognition results from Aurora-4 indicate that the articulatory features from the DNN provide improvement in speech recognition performance when fused with other standard cepstral features; however when tried by themselves, they failed to match the baseline performance.
更多查看译文
关键词
cepstral analysis,feature extraction,natural language processing,neural nets,speech recognition,Aurora-4,DNN,Haskins Laboratories task-dynamic model,WSJ0 corpus,acoustic feature candidate,articulatory features,articulatory information extraction,articulatory trajectory estimation,cepstral features,continuous speech recognition task,deep neural networks,noisy Wall Street Journal corpus,speech production,speech signal,synthetic English words,training data,articulatory trajectories,automatic speech recognition,deep neural networks,vocal tract variables
AI 理解论文
溯源树
样例
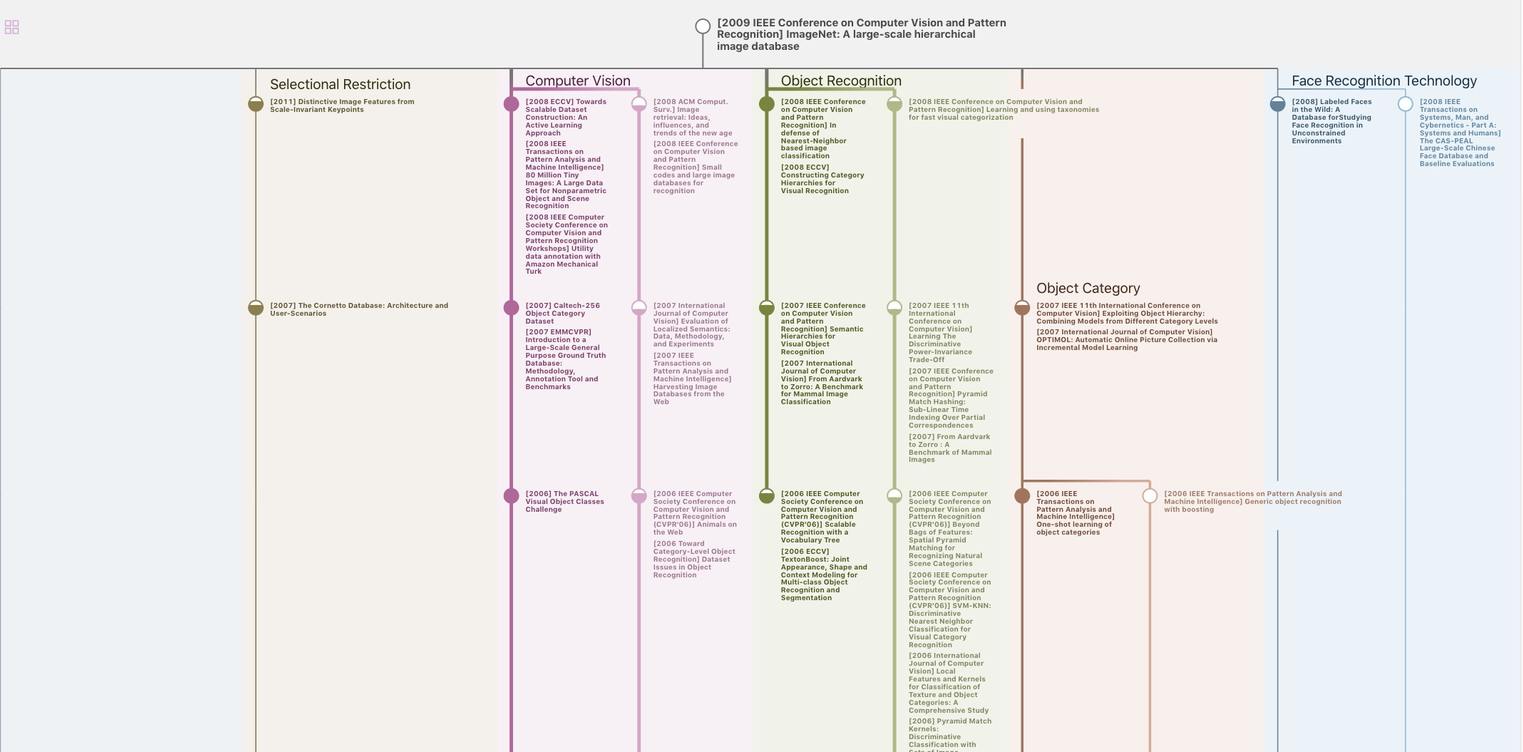
生成溯源树,研究论文发展脉络
Chat Paper
正在生成论文摘要