A block coordinate descent method of multipliers: Convergence analysis and applications
ICASSP(2014)
摘要
In this paper, we consider a nonsmooth convex problem with linear coupling constraints. Problems of this form arise in many modern large-scale signal processing applications including the provision of smart grid networks. In this work, we propose a new class of algorithms called the block coordinate descent method of multipliers (BCDMM) to solve this family of problems. The BCDMM is a primal-dual type of algorithm. It optimizes an (approximate) augmented Lagrangian of the original problem one block variable per iteration, followed by a gradient update for the dual variable. We show that under certain regularity conditions, and when the order for which the block variables are either updated in a deterministic or a random fashion, the BCDMM converges to the set of optimal solutions. The effectiveness of the algorithm is illustrated using large-scale basis pursuit and smart grid problems.
更多查看译文
关键词
smart grid networks,bcdmm,convex programming,nonsmooth convex problem,augmented lagrangian,large-scale basis pursuit,gradient methods,smart power grids,block variables,primal-dual type algorithm,large-scale signal processing,linear coupling constraints,block coordinate descent method of multipliers,gradient update,convex functions,convergence,approximation algorithms,optimization,smart grids,minimization
AI 理解论文
溯源树
样例
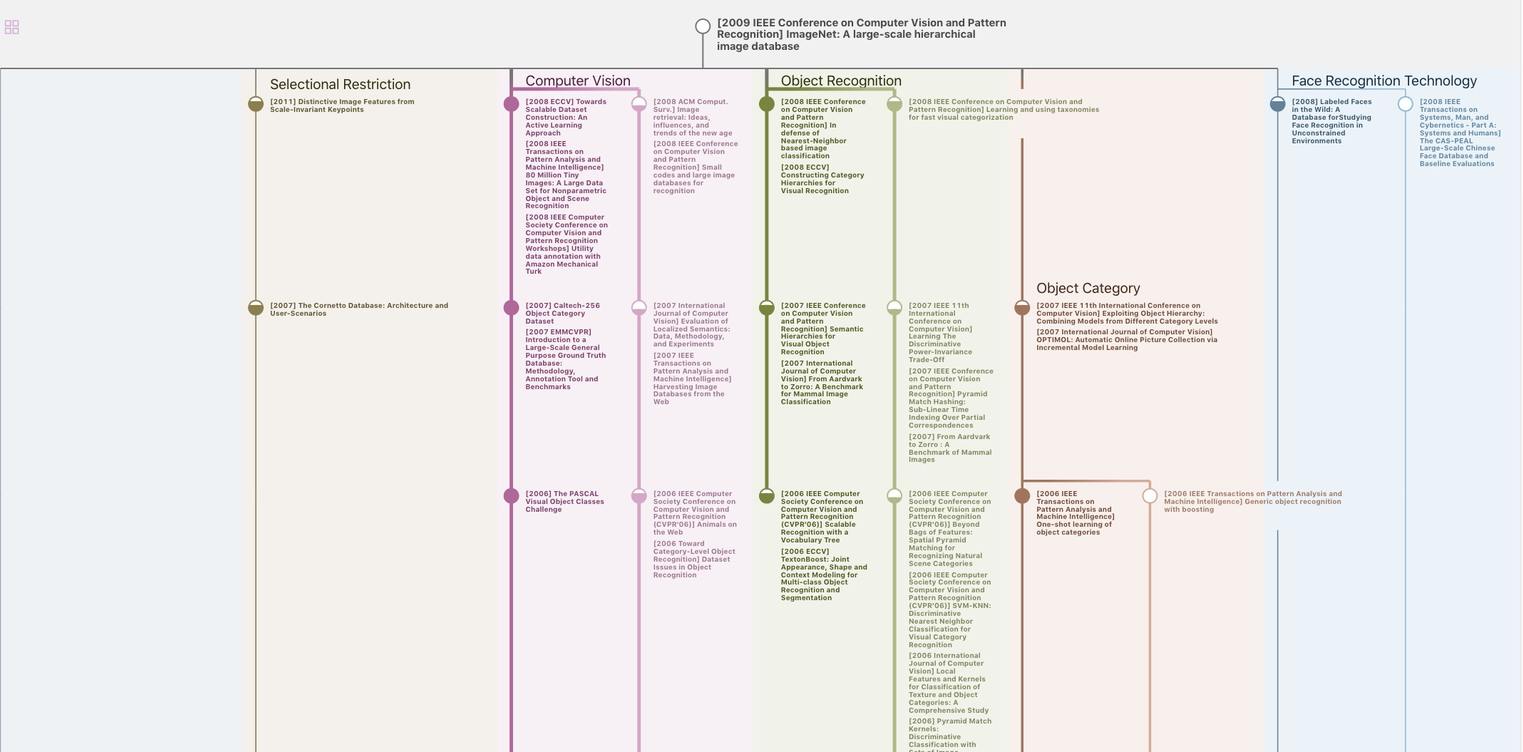
生成溯源树,研究论文发展脉络
Chat Paper
正在生成论文摘要