A novel scheme for speaker recognition using a phonetically-aware deep neural network
ICASSP(2014)
摘要
We propose a novel framework for speaker recognition in which extraction of sufficient statistics for the state-of-the-art i-vector model is driven by a deep neural network (DNN) trained for automatic speech recognition (ASR). Specifically, the DNN replaces the standard Gaussian mixture model (GMM) to produce frame alignments. The use of an ASR-DNN system in the speaker recognition pipeline is attractive as it integrates the information from speech content directly into the statistics, allowing the standard backends to remain unchanged. Improvement from the proposed framework compared to a state-of-the-art system are of 30% relative at the equal error rate when evaluated on the telephone conditions from the 2012 NIST speaker recognition evaluation (SRE). The proposed framework is a successful way to efficiently leverage transcribed data for speaker recognition, thus opening up a wide spectrum of research directions.
更多查看译文
关键词
deep neural network,speech content,statistic extraction,frame alignment,standard gaussian mixture model,speaker recognition,2012 nist speaker recognition evaluation,asr-dnn system,2012 nist sre,asr,gaussian processes,standard gmm,phonetically-aware deep neural network,speaker recognition pipeline,i-vector model,neural nets,automatic speech recognition,mathematical model,nist,hidden markov models,statistics,speech recognition,speech
AI 理解论文
溯源树
样例
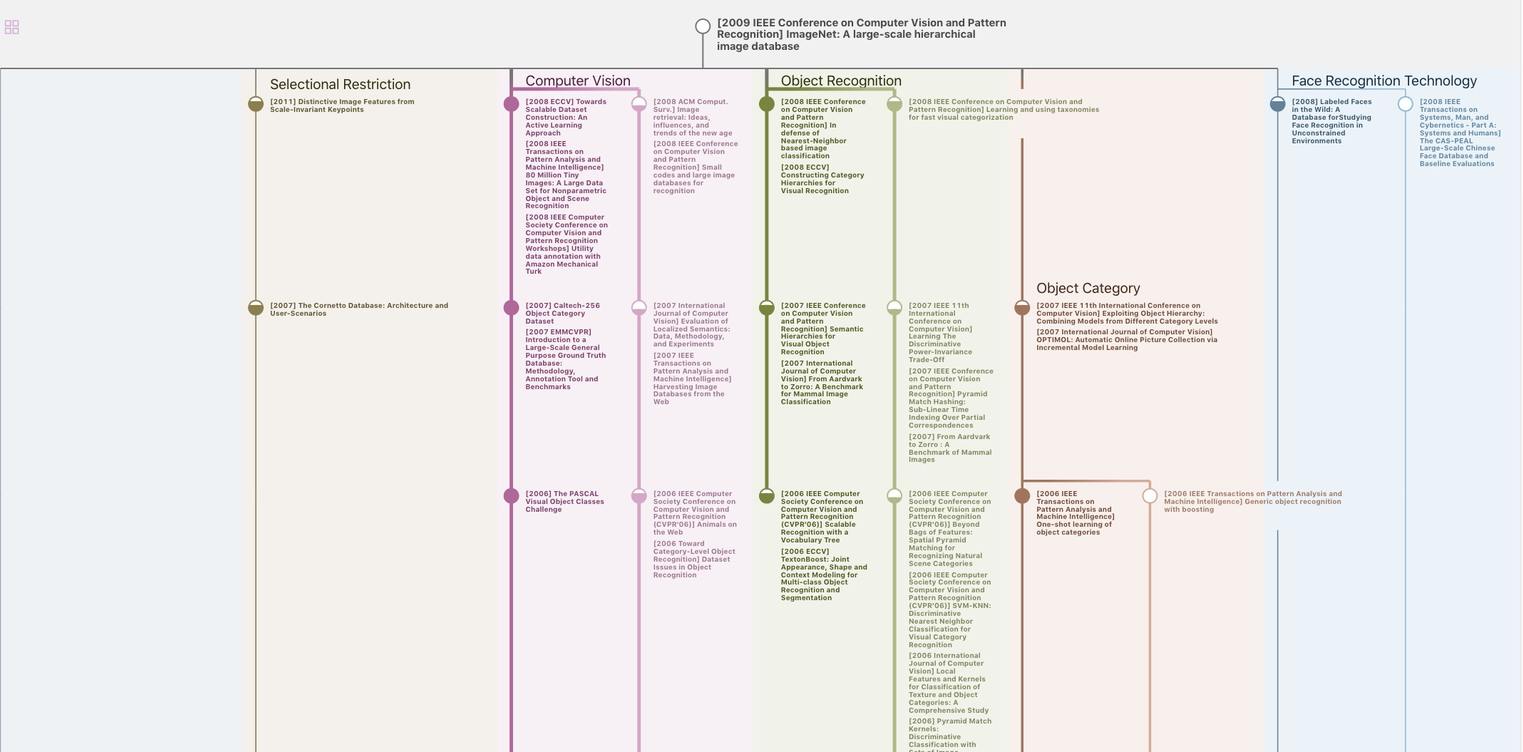
生成溯源树,研究论文发展脉络
Chat Paper
正在生成论文摘要