Singular value decomposition based low-footprint speaker adaptation and personalization for deep neural network
ICASSP(2014)
摘要
The large number of parameters in deep neural networks (DNN) for automatic speech recognition (ASR) makes speaker adaptation very challenging. It also limits the use of speaker personalization due to the huge storage cost in large-scale deployments. In this paper we address DNN adaptation and personalization issues by presenting two methods based on the singular value decomposition (SVD). The first method uses an SVD to replace the weight matrix of a speaker independent DNN by the product of two low rank matrices. Adaptation is then performed by updating a square matrix inserted between the two low-rank matrices. In the second method, we adapt the full weight matrix but only store the delta matrix - the difference between the original and adapted weight matrices. We decrease the footprint of the adapted model by storing a reduced rank version of the delta matrix via an SVD. The proposed methods were evaluated on short message dictation task. Experimental results show that we can obtain similar accuracy improvements as the previously proposed Kullback-Leibler divergence (KLD) regularized method with far fewer parameters, which only requires 0.89% of the original model storage.
更多查看译文
关键词
deep neural network,weight matrix,dnn adaptation,speaker personalization,kld,speaker independent dnn,speech recognition,kullback-leibler divergence,low rank matrices,svd,low-footprint speaker adaptation,matrix algebra,square matrix,storage cost,short message dictation task,delta matrix,asr,speaker adaptation,singular value decomposition,neural nets,automatic speech recognition,accuracy,hidden markov models,data models,matrix decomposition,kullback leibler divergence,neural networks,silicon
AI 理解论文
溯源树
样例
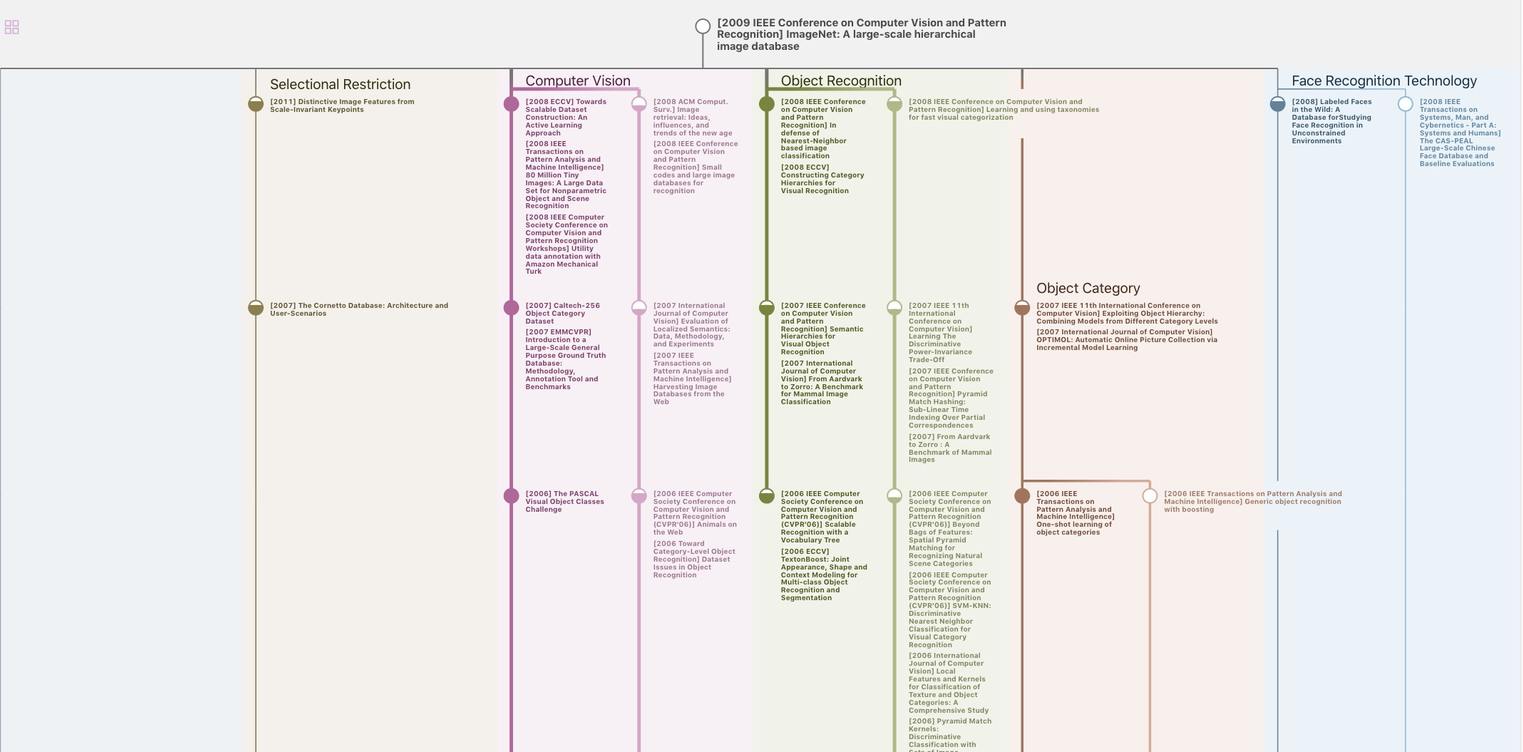
生成溯源树,研究论文发展脉络
Chat Paper
正在生成论文摘要