A Study On Multi-Objective Particle Swarm Optimization With Weighted Scalarizing Functions
Winter Simulation Conference(2014)
摘要
In literature, multi-objective particle swarm optimization (PSO) algorithms are shown to have great potential in solving simulation optimization problems with real number decision variables and objectives. This paper develops a multi-objective PSO algorithm based on weighted scalarization (MPSOws) in which objectives are scalarized by different sets of weights at individual particles while evaluation results are shared among the swarm. Various scalarizing functions, such as simple weighted aggregation (SWA), weighted compromise programming (WCP), and penalized boundary intersection (PBI) can be applied in the algorithm. To improve the diversity and uniformity of the Pareto set, a hybrid external archiving technique consisting of both KNN and epsilon-dominance methods is proposed. Numerical experiments on noise-free problems are conducted to show that MPSOws outperforms the benchmark algorithm and WCP is the most preferable strategy for the scalarization. In addition, simulation allocation rules (SARs) can be further applied with MPSOws when evaluation error is considered.
更多查看译文
关键词
Pareto optimisation,particle swarm optimisation,ε-dominance methods,KNN,MPSOws,PBI,Pareto set diversity,Pareto set uniformity,SAR,SWA,WCP,decision variables,evaluation error,hybrid external archiving technique,multiobjective PSO algorithm based on weighted scalarization,multiobjective particle swarm optimization,noise-free problems,penalized boundary intersection,simple weighted aggregation,simulation allocation rules,simulation optimization problems,weighted compromise programming,weighted scalarizing functions,
AI 理解论文
溯源树
样例
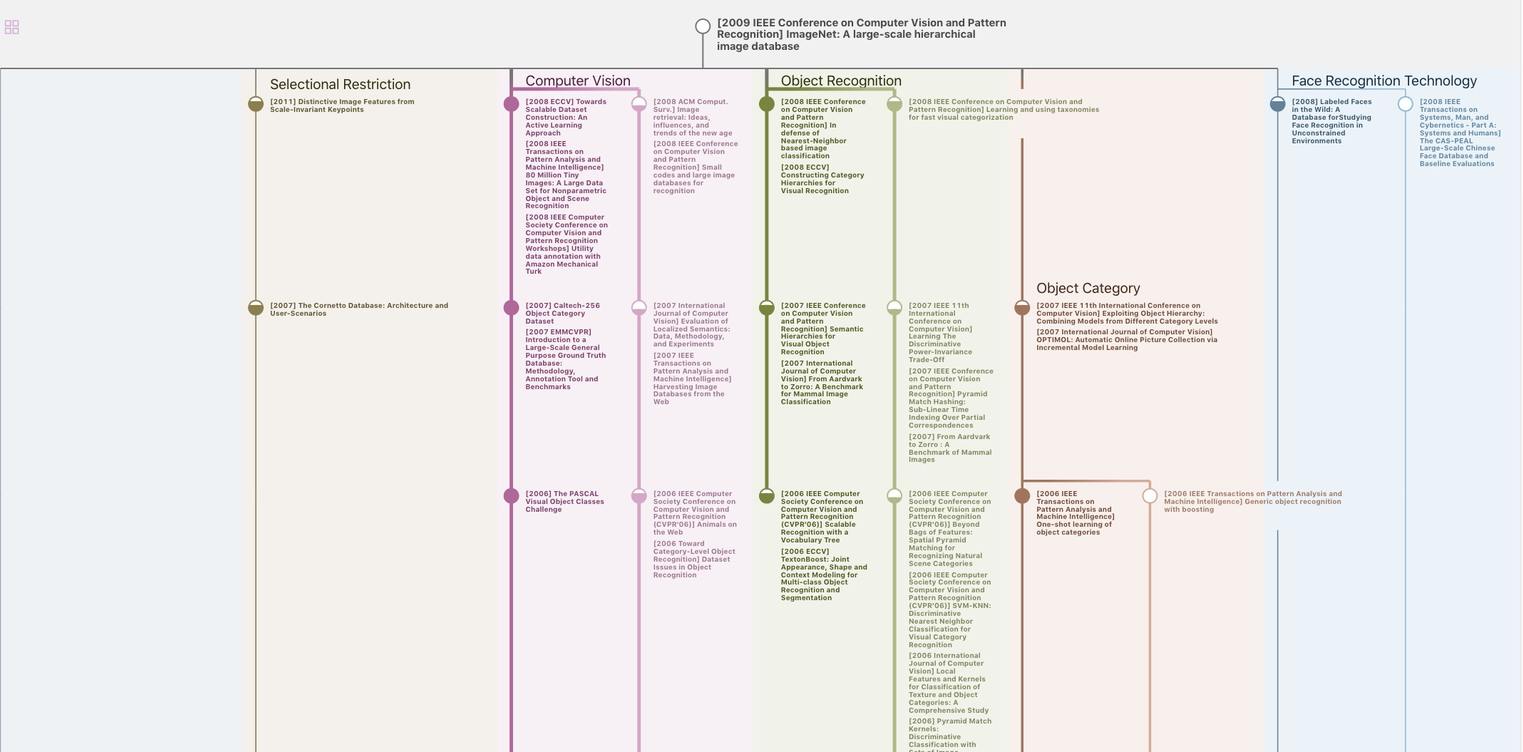
生成溯源树,研究论文发展脉络
Chat Paper
正在生成论文摘要