Efficient multi-fidelity simulation optimization
Winter Simulation Conference(2014)
摘要
Simulation models of different fidelity levels are often available for a complex system. High-fidelity simulations are accurate but time-consuming. Therefore, they can only be applied to a small number of solutions. Low-fidelity simulations are faster and can evaluate a large number of solutions. But their results may contain significant bias and variability. We propose an Multi-fidelity Optimization with Ordinal Transformation and Optimal Sampling (MO2TOS) framework to exploit the benefits of high- and low-fidelity simulations to efficiently identify a (near) optimal solution. MO2TOS uses low-fidelity simulations for all solutions and then assigns a fixed budget of high-fidelity simulations to solutions based on low-fidelity simulation results. We show the benefits of MO2TOS via theoretical analysis and numerical experiments with deterministic simulations and stochastic simulations where noise is negligible with sufficient replications. We compare MO2TOS to Equal Allocation (EA) and Optimal Computing Budget Allocation (OCBA). MO2TOS consistently outperforms both EA and OCBA.
更多查看译文
关键词
optimisation,stochastic processes,stochastic simulation,deterministic simulation,ocba,optimal computing budget allocation,multifidelity simulation optimization,ordinal transformation,digital simulation,optimal sampling,sampling methods,mo2tos framework
AI 理解论文
溯源树
样例
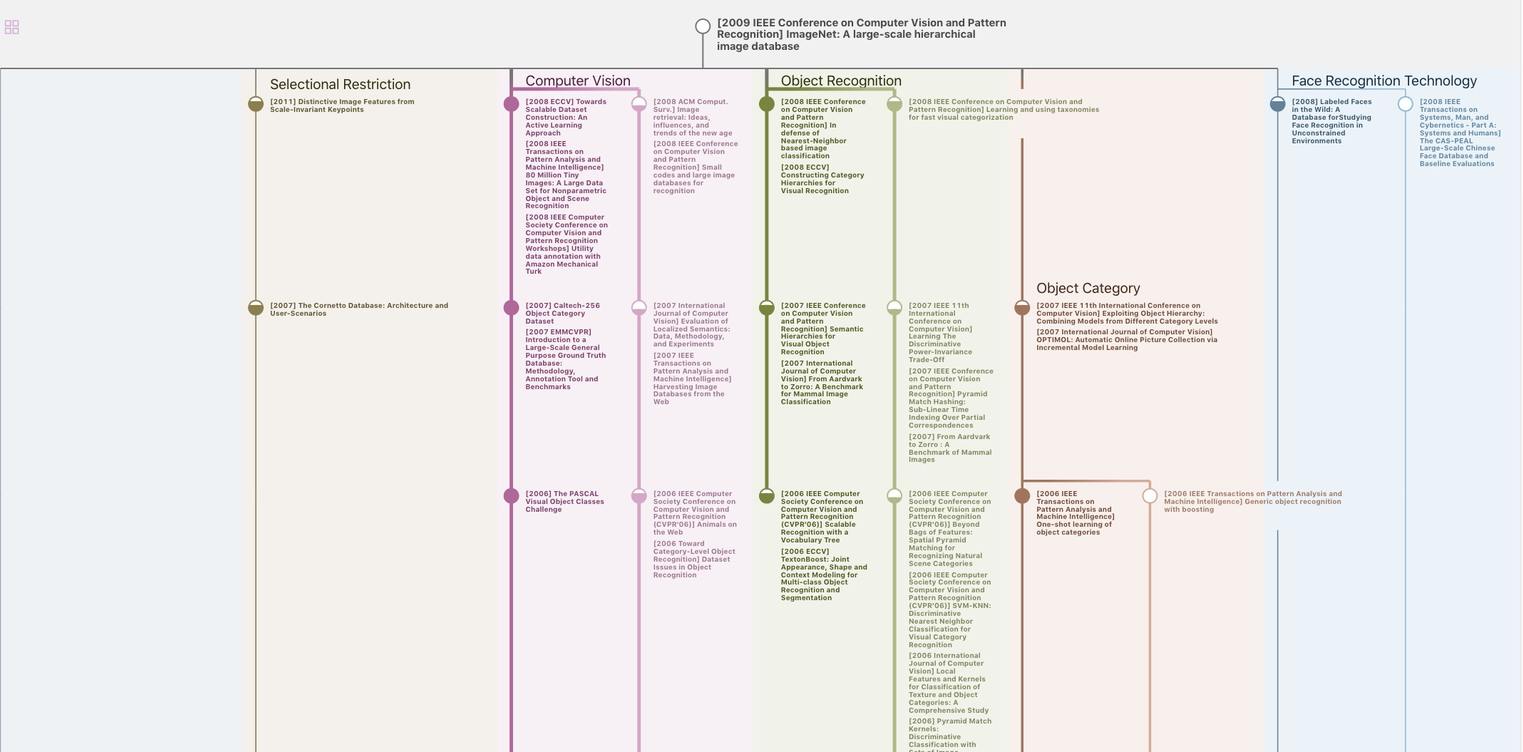
生成溯源树,研究论文发展脉络
Chat Paper
正在生成论文摘要