Primal-Dual Framework for Feature Selection using Least Squares Support Vector Machines.
COMAD '13: Proceedings of the 19th International Conference on Management of Data(2013)
摘要
Least Squares Support Vector Machines (LSSVM) perform classification using L 2 -norm on the weight vector and a squared loss function with linear constraints. The major advantage over classical L 2 -norm support vector machine (SVM) is that it solves a system of linear equations rather than solving a quadratic programming problem. The L 2 -norm penalty on the weight vectors is known to robustly select features. The zero-norm or the number of non-zero elements in a vector is an ideal quantity for feature selection. The L 0 -norm minimization is a computationally intractable problem. However, a convex relaxation to the direct zero-norm minimization was proposed recently. In this paper, we propose a combination of L 2 -norm penalty and the convex relaxation of the L 0 -norm penalty for feature selection in classification problems. We propose a primal-dual framework for feature selection using the combination of L 2 -norm and L 0 -norm penalty resulting in closed form solution. A series of experiments on microarray data and UCI data demonstrates that our proposed method results in better performance.
更多查看译文
AI 理解论文
溯源树
样例
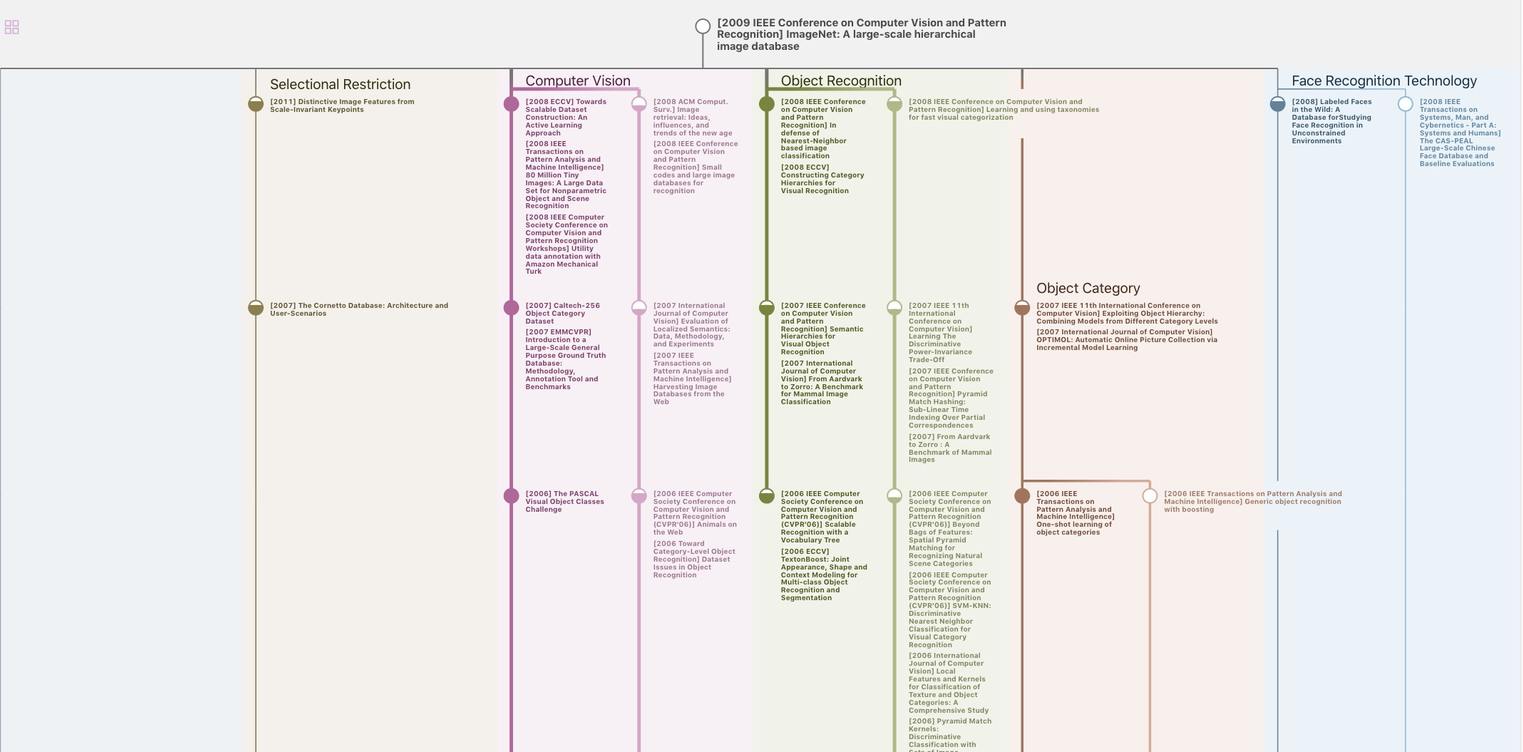
生成溯源树,研究论文发展脉络
Chat Paper
正在生成论文摘要