Kernel Sparse Subspace Clustering
2014 IEEE INTERNATIONAL CONFERENCE ON IMAGE PROCESSING (ICIP)(2014)
摘要
Subspace clustering refers to the problem of grouping data points that lie in a union of low-dimensional subspaces. One successful approach for solving this problem is sparse subspace clustering, which is based on a sparse representation of the data. In this paper, we extend SSC to non-linear manifolds by using the kernel trick. We show that the alternating direction method of multipliers can be used to efficiently find kernel sparse representations. Various experiments on synthetic as well real datasets show that non-linear mappings lead to sparse representation that give better clustering results than state-of-the-art methods.
更多查看译文
关键词
Subspace clustering, sparse subspace clustering, kernel methods, non-linear subspace clustering
AI 理解论文
溯源树
样例
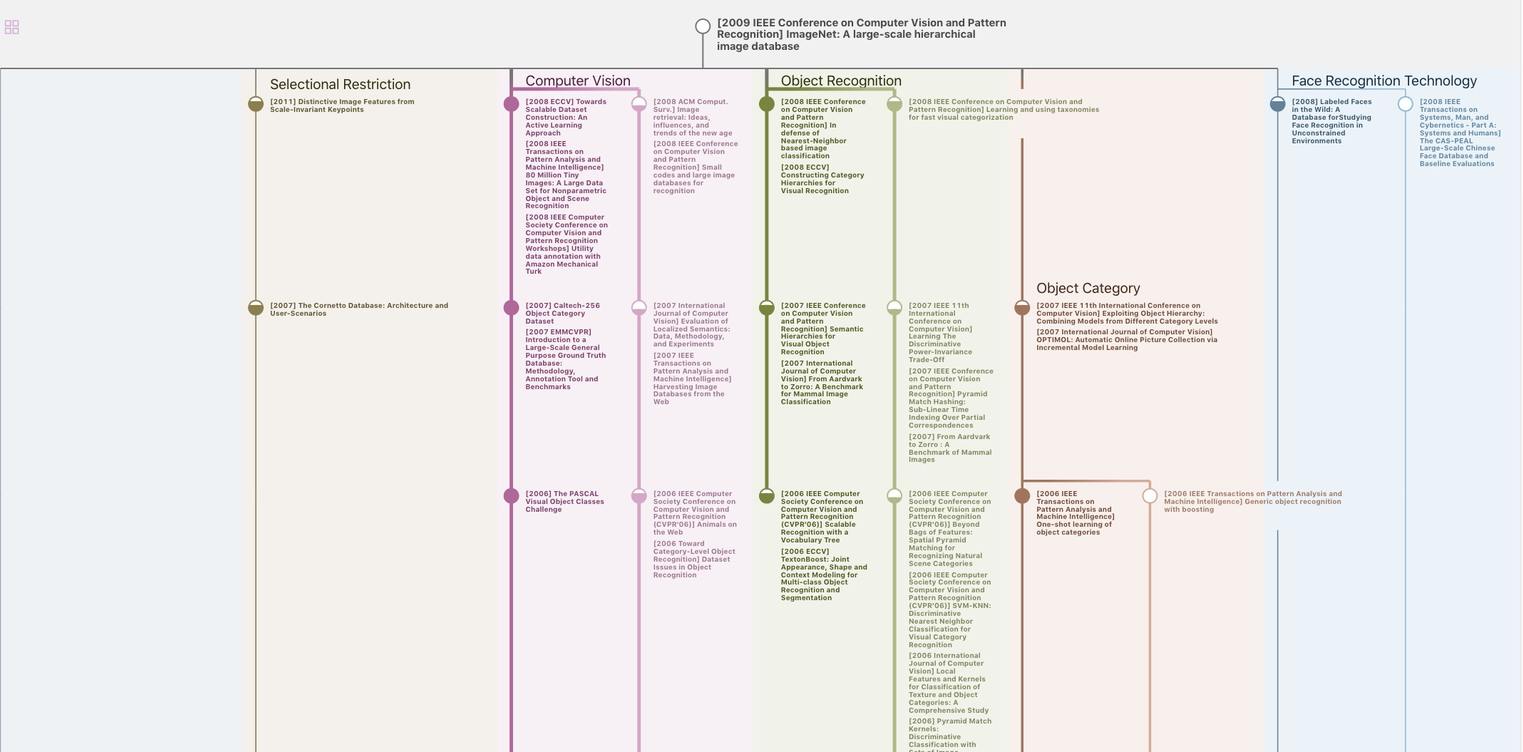
生成溯源树,研究论文发展脉络
Chat Paper
正在生成论文摘要