Recursive Low-rank and Sparse Recovery of Surveillance Video using Compressed Sensing
ICDSC(2014)
摘要
This paper focuses on surveillance video processing using Compressed Sensing (CS). The CS measurements are used for recovery of the video frame into a low-rank background component and sparse component that corresponds to the moving object. The spatial and temporal low-rank features of the video frame, e.g., the nonlocal similar patches within the single video frame and the low-rank background component residing in multiple frames, are successfully exploited. We propose rLSDR that consists of three major components. First we develop an efficient single frame CS recovery algorithm, called NLDR, that operates on the nonlocal similarity patches within each frame to solve the low-rank optimization problem with the CS measurements constraint using Douglas-Rachford splitting method. Second, after obtaining a few NLDR recovered frames as training, a fast bilateral random projections (BRP) scheme is adopted for quick low-rank background initialization. Third, rLSDR then incorporates real-time single video frame to recursively recover the sparse component and update the background, where the proposed NLDR algorithm can also be used here for sparse component estimation. Experimental results on standard surveillance videos demonstrate that NLDR performs the best for single frame CS recovery compared with the state-of-the-art and rLSDR could successfully recover the background and sparse object with less resource consumption.
更多查看译文
关键词
algorithms,compressed sensing,design,distributed applications,experimentation,low-rank approximation,measurement,performance,restoration,sparse recovery,surveillance video processing
AI 理解论文
溯源树
样例
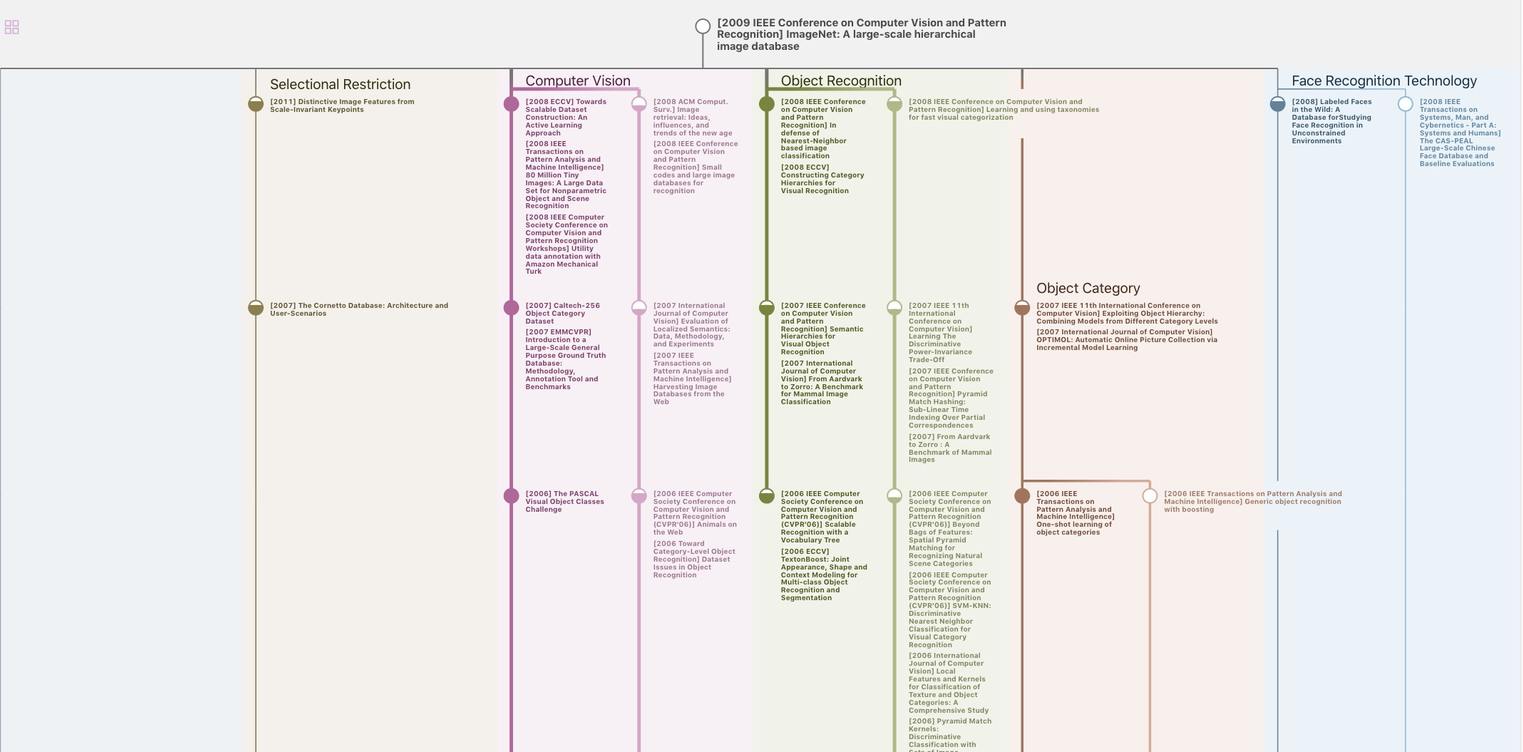
生成溯源树,研究论文发展脉络
Chat Paper
正在生成论文摘要