In defense of iterated conditional mode for hyperspectral image classification
ICME(2014)
摘要
Hyperspectral image classification is one of the most significant topics in remote sensing. A large number of methods have been proposed to improve the classification accuracy. However, the improvement often comes at the cost of higher complexity. In this work, we mainly focus on the Markov Random Fields related paradigm, which involves a demanding energy minimization procedure. Traditional methods are prone to employ the advanced optimization techniques. On the contrary, this paper is in defense of a simple yet efficient method for hyperspectral image classification, Iterated Conditional Mode, which has been generally considered inferior to other state-of-the-art methods. Our purpose is successfully achieved by tackling two inherent drawbacks of ICM, sensitive label initialization and local minimum. We apply our method to three real-world hyperspectral images, and compare the results with those of state-of-the-art methods. The comparisons show that the proposed method outperforms its competitors.
更多查看译文
关键词
energy minimization procedure,remote sensing,hyperspectral image classification,sensitive label initialization,iterated conditional mode,svm,advanced optimization techniques,image classification,support vector machine,geophysical image processing,hyperspectral imaging,markov processes,support vector machines,markov random fields,real-world hyperspectral images
AI 理解论文
溯源树
样例
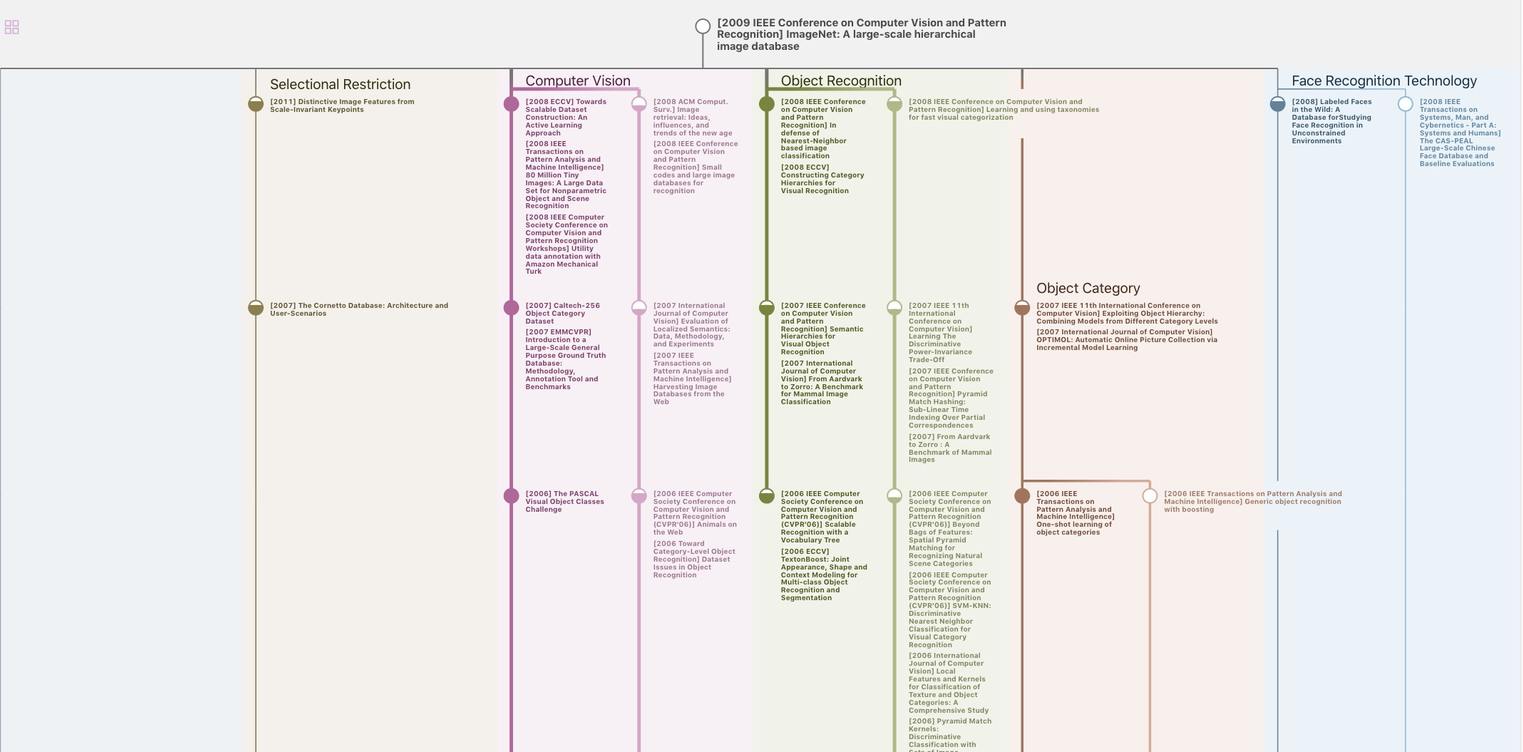
生成溯源树,研究论文发展脉络
Chat Paper
正在生成论文摘要