Community discovering guided cold-start recommendation: A discriminative approach
ICME(2014)
摘要
Recommendation for new users is a key challenge due to the lack of prior information from them, which is the well-known cold-start problem. Preference elicitation has been proposed as an efficient strategy for eliciting new users preference through an initial interview where new users are queried by elaborately selected items. In this paper, we propose a novel community discovering guided discriminative selection (CDDS) model for constructing query set. We exploit the community as an effective information which is not fully used in existing approaches. By integrating item selection and community discovery into one framework, our model selects most discriminative items for preference elicitation, with guidance of unsupervised community discovering process. To perform community discovering process, the model utilizes rating similarity graph and social network as a graph regular-ization. Experimental results on real-world datasets Flixster and Douban demonstrate that the proposed method outperforms traditional preference elicitation methods for cold-start recommendation.
更多查看译文
关键词
community discovering guided cold-start recommendation:,cdds,social network,douban,community discovering guided discriminative selection model,recommender systems,cold-start recommendation,discriminative approach,item selection,rating similarity graph,preference elicitation,flixster,graph theory,community discovering,social networking (online),graph regularization,query set construction,interviews,indexes,minimization,optimization,vectors
AI 理解论文
溯源树
样例
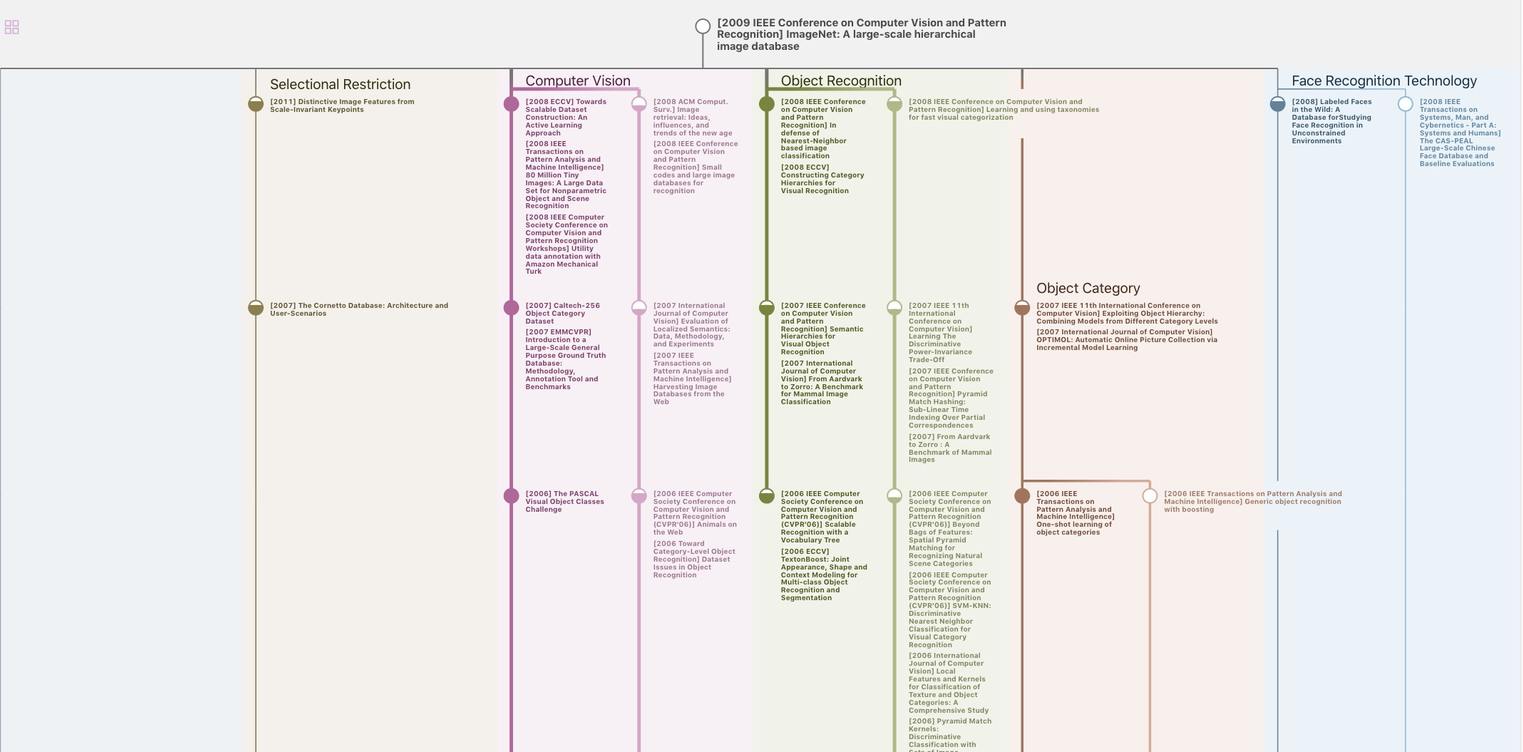
生成溯源树,研究论文发展脉络
Chat Paper
正在生成论文摘要