Attributes Make Sense On Segmented Objects
COMPUTER VISION - ECCV 2014, PT VI(2014)
摘要
In this paper we aim for object classification and segmentation by attributes. Where existing work considers attributes either for the global image or for the parts of the object, we propose, as our first novelty, to learn and extract attributes on segments containing the entire object. Object-level attributes suffer less from accidental content around the object and accidental image conditions such as partial occlusions, scale changes and viewpoint changes. As our second novelty, we propose joint learning for simultaneous object classification and segment proposal ranking, solely on the basis of attributes. This naturally brings us to our third novelty: object-level attributes for zero-shot, where we use attribute descriptions of unseen classes for localizing their instances in new images and classifying them accordingly. Results on the Caltech UCSD Birds, Leeds Butterflies, and an a-Pascal subset demonstrate that i) extracting attributes on oracle object-level brings substantial benefits ii) our joint learning model leads to accurate attribute-based classification and segmentation, approaching the oracle results and iii) object-level attributes also allow for zero-shot classification and segmentation. We conclude that attributes make sense on segmented objects.
更多查看译文
关键词
attributes, segmentation, zero-shot classification
AI 理解论文
溯源树
样例
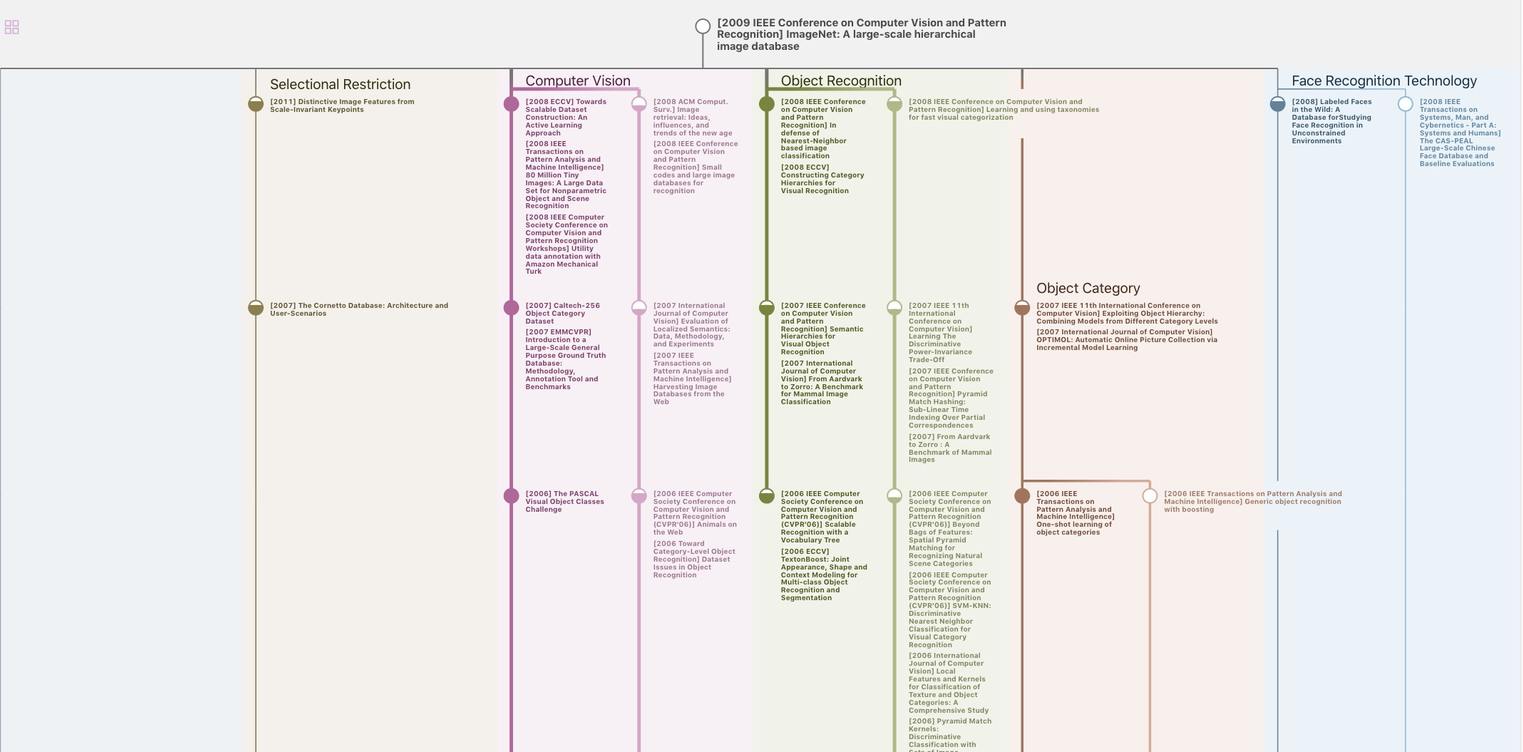
生成溯源树,研究论文发展脉络
Chat Paper
正在生成论文摘要