Robust Instance Recognition In Presence Of Occlusion And Clutter
COMPUTER VISION - ECCV 2014, PT II(2014)
摘要
We present a robust learning based instance recognition framework from single view point clouds. Our framework is able to handle real-world instance recognition challenges, i.e, clutter, similar looking distractors and occlusion. Recent algorithms have separately tried to address the problem of clutter [9] and occlusion [16] but fail when these challenges are combined. In comparison we handle all challenges within a single framework. Our framework uses a soft label Random Forest [5] to learn discriminative shape features of an object and use them to classify both its location and pose. We propose a novel iterative training scheme for forests which maximizes the margin between classes to improve recognition accuracy, as compared to a conventional training procedure. The learnt forest outperforms template matching, DPM [7] in presence of similar looking distractors. Using occlusion information, computed from the depth data, the forest learns to emphasize the shape features from the visible regions thus making it robust to occlusion. We benchmark our system with the state-of-the-art recognition systems [9,7] in challenging scenes drawn from the largest publicly available dataset. To complement the lack of occlusion tests in this dataset, we introduce our Desk3D dataset and demonstrate that our algorithm outperforms other methods in all settings.
更多查看译文
关键词
Point Cloud,Depth Image,Object Instance,Kinect Sensor,Dominant Orientation
AI 理解论文
溯源树
样例
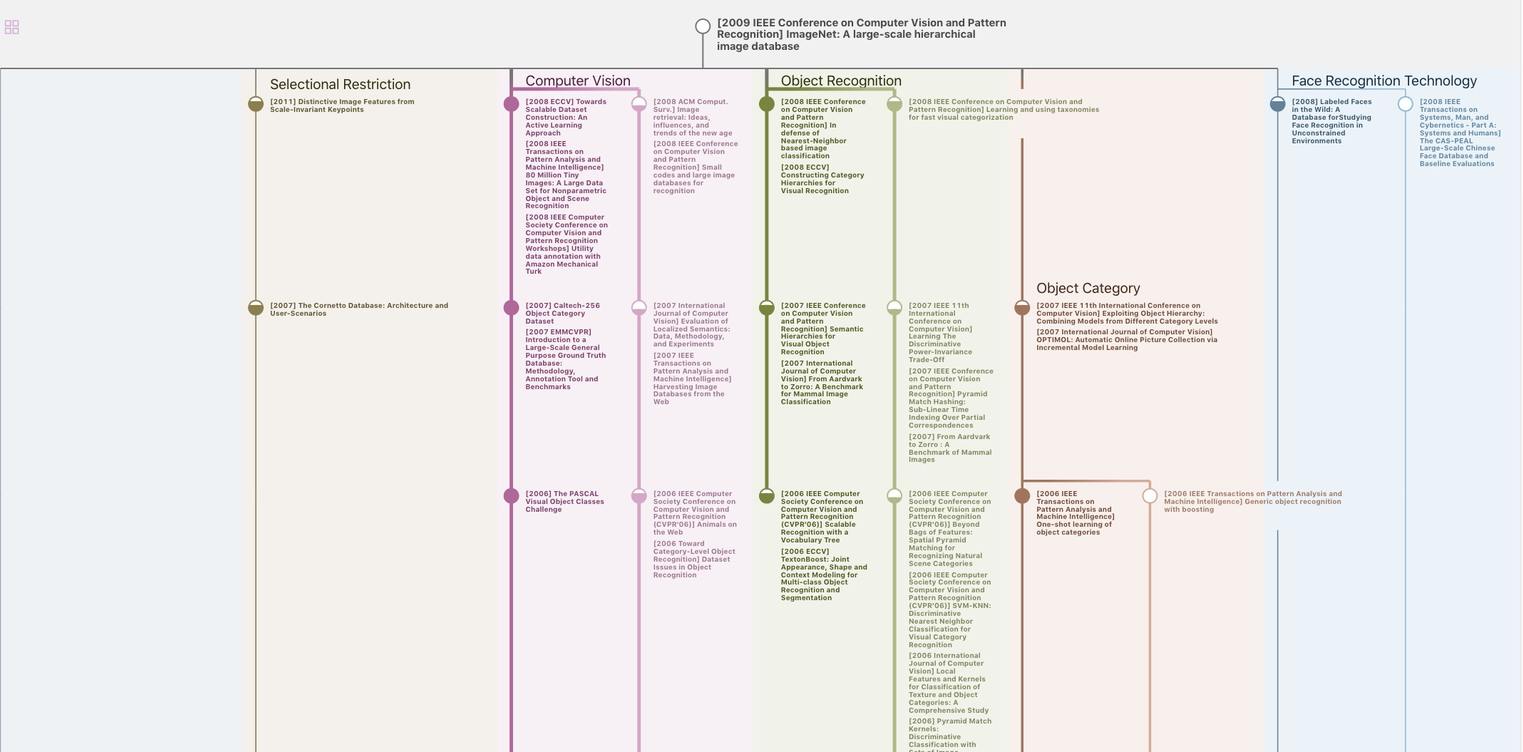
生成溯源树,研究论文发展脉络
Chat Paper
正在生成论文摘要