Compressed dictionary learning for detecting activations in fMRI using double sparsity
GlobalSIP(2014)
摘要
This paper focuses on detecting activated voxels in fMRI data by exploiting the sparsity of the BOLD signal. Due to the large volume of the data, we propose to learn a dictionary from the compressed measurements of the BOLD signal. The solution to the inverse problem induced by the General Linear Model is then sought through sparse coding using the double sparsity model, where sparsity is imposed on both the learnt dictionary and the generated coefficients. The estimated sparse coefficients are then used to decide whether or not a stimulus is presented in the observed BOLD signal. Experimental results on real fMRI data demonstrate that the proposed method leads to similar activated regions as compared to those activated by the Statistical Parametric Mapping (SPM) software but with much less samples needed.
更多查看译文
关键词
statistical parametric mapping,sparse coefficients,double sparsity model,compressed sensing,learning (artificial intelligence),bold signal sparsity,spm software,dictionary learning,blood-oxygenation-level- dependent signal,general linear model,activated voxel detection,functional magnetic resonance imaging,biomedical mri,compressed dictionary learning,object detection,double sparsity,fmri activation detection,medical image processing,fmri,dictionaries,vectors,time series analysis,sparse matrices,encoding
AI 理解论文
溯源树
样例
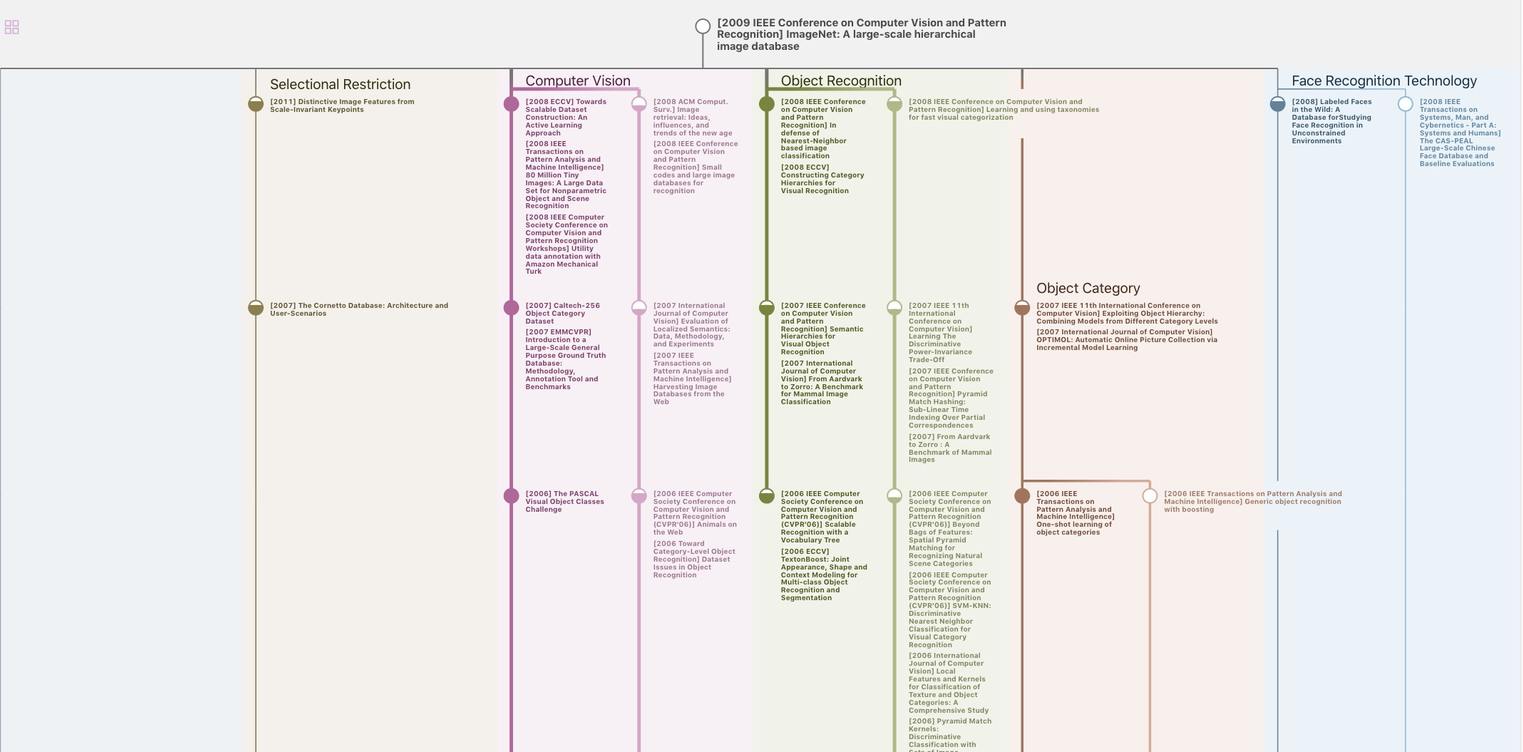
生成溯源树,研究论文发展脉络
Chat Paper
正在生成论文摘要