Emotion recognition of conversational affective speech using temporal course modeling-based error weighted cross-correlation model
APSIPA(2014)
摘要
A complete emotional expression in natural face-to-face conversation typically contains a complex temporal course. In this paper, we propose a temporal course modeling-based error weighted cross-correlation model (TCM-EWCCM) for speech emotion recognition. In TCM-EWCCM, a TCM-based cross-correlation model (CCM) is first used to not only model the temporal evolution of the extracted acoustic and prosodic features individually but also construct the statistical dependencies among paired acoustic-prosodic features in different emotional states. Then, a Bayesian classifier weighting scheme named error weighted classifier combination is adopted to explore the contributions of the individual TCM-based CCM classifiers for different acoustic-prosodic feature pairs to enhance the speech emotion recognition accuracy. The results of experiments on the NCKU-CASC corpus demonstrate that modeling the complex temporal structure and considering the statistical dependencies as well as contributions among paired features in natural conversation speech can indeed improve the speech emotion recognition performance.
更多查看译文
关键词
error weighted cross-correlation model,ncku-casc corpus,complex temporal course,speech recognition,statistical dependencies,bayes methods,statistical analysis,natural face-to-face conversation,conversational affective speech,emotion recognition,tcm-ewccm,speech emotion recognition,temporal course modeling,bayesian classifier weighting scheme,paired acoustic-prosodic features,speech,decision support systems,acoustics,feature extraction
AI 理解论文
溯源树
样例
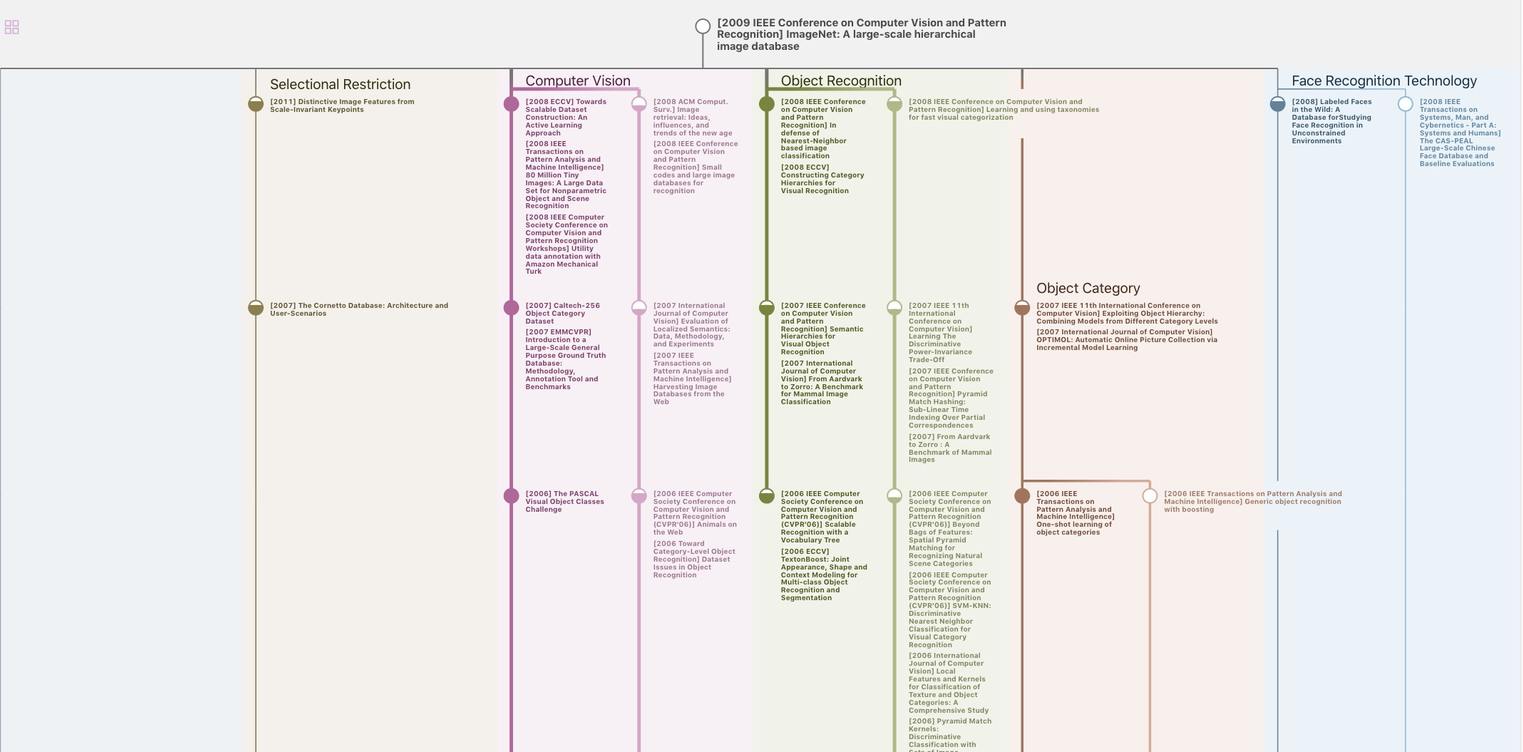
生成溯源树,研究论文发展脉络
Chat Paper
正在生成论文摘要