Is Multi-model Feature Matching Better for Endoscopic Motion Estimation?
COMPUTER-ASSISTED AND ROBOTIC ENDOSCOPY, CARE 2014(2014)
摘要
Camera motion estimation is a standard yet critical step to endoscopic visualization. It is affected by the variation of locations and correspondences of features detected in 2D images. Feature detectors and descriptors vary, though one of the most widely used remains SIFT. Practitioners usually also adopt its feature matching strategy, which defines inliers as the feature pairs subjecting to a global affine transformation. However, for endoscopic videos, we are curious if it is more suitable to cluster features into multiple groups. We can still enforce the same transformation as in SIFT within each group. Such a multi-model idea has been recently examined in the Multi-Affine work, which outperforms Lowe's SIFT in terms of re-projection error on minimally invasive endoscopic images with manually labelled ground-truth matches of SIFT features. Since their difference lies in matching, the accuracy gain of estimated motion is attributed to the holistic Multi-Affine feature matching algorithm. But, more concretely, the matching criterion and point searching can be the same as those built in SIFT. We argue that the real variation is only the motion model verification. We either enforce a single global motion model or employ a group of multiple local ones. In this paper, we investigate how sensitive the estimated motion is affected by the number of motion models assumed in feature matching. While the sensitivity can be analytically evaluated, we present an empirical analysis in a leaving-one-out cross validation setting without requiring labels of ground-truth matches. Then, the sensitivity is characterized by the variance of a sequence of motion estimates. We present a series of quantitative comparison such as accuracy and variance between Multi-Affine motion models and the global affine model.
更多查看译文
关键词
Motion Model, Scale Invariant Feature Transform, Feature Match, Functional Endoscopic Sinus Surgery, Feature Pair
AI 理解论文
溯源树
样例
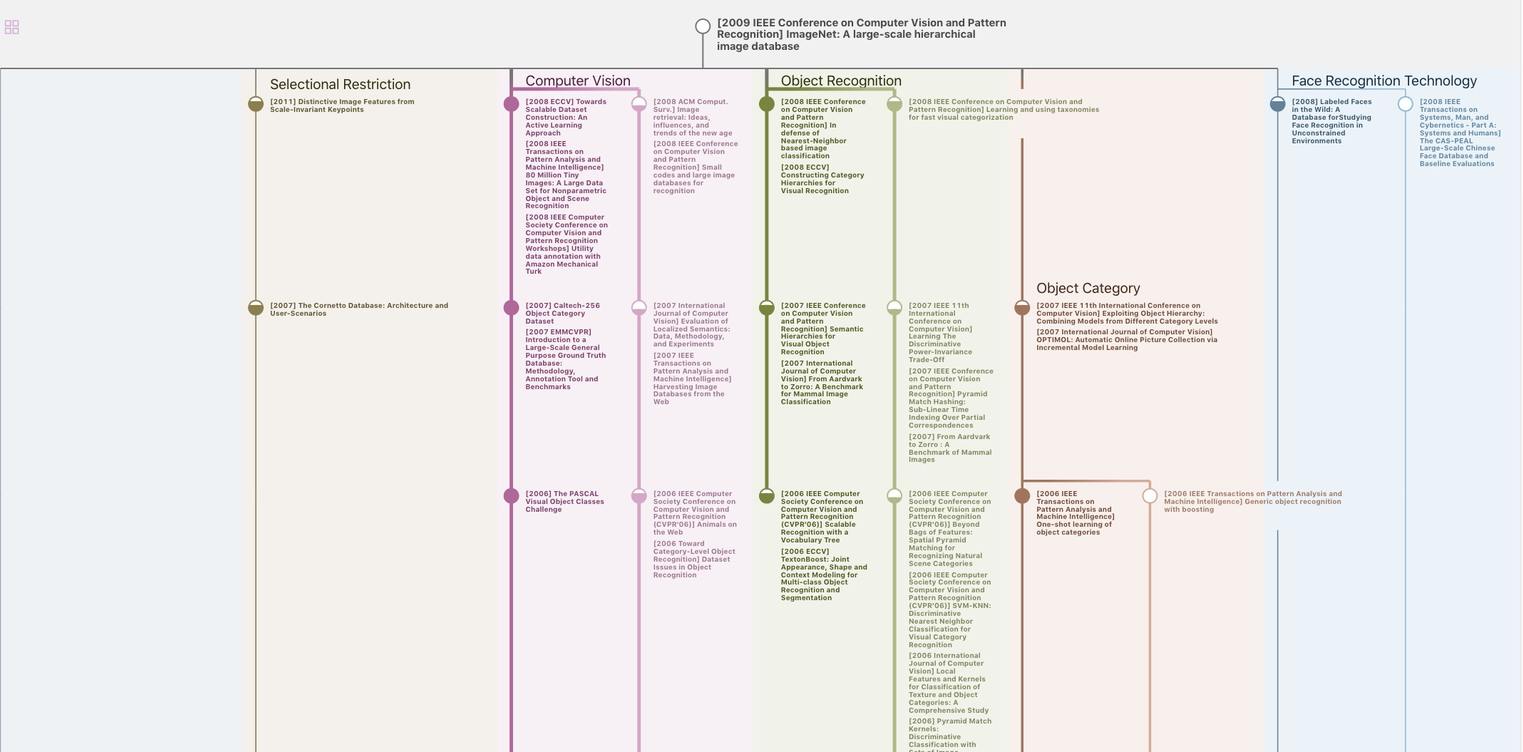
生成溯源树,研究论文发展脉络
Chat Paper
正在生成论文摘要