Fast LSTD using stochastic approximation: Finite time analysis and application to traffic control
Machine Learning and Knowledge Discovery in Databases(2014)
摘要
We propose a stochastic approximation based method with randomisation of samples for policy evaluation using the least squares temporal difference (LSTD) algorithm. Our method results in an $O(d)$ improvement in complexity in comparison to regular LSTD, where $d$ is the dimension of the data. We provide convergence rate results for our proposed method, both in high probability and in expectation. Moreover, we also establish that using our scheme in place of LSTD does not impact the rate of convergence of the approximate value function to the true value function. This result coupled with the low complexity of our method makes it attractive for implementation in big data settings, where $d$ is large. Further, we also analyse a similar low-complexity alternative for least squares regression and provide finite-time bounds there. We demonstrate the practicality of our method for LSTD empirically by combining it with the LSPI algorithm in a traffic signal control application.
更多查看译文
AI 理解论文
溯源树
样例
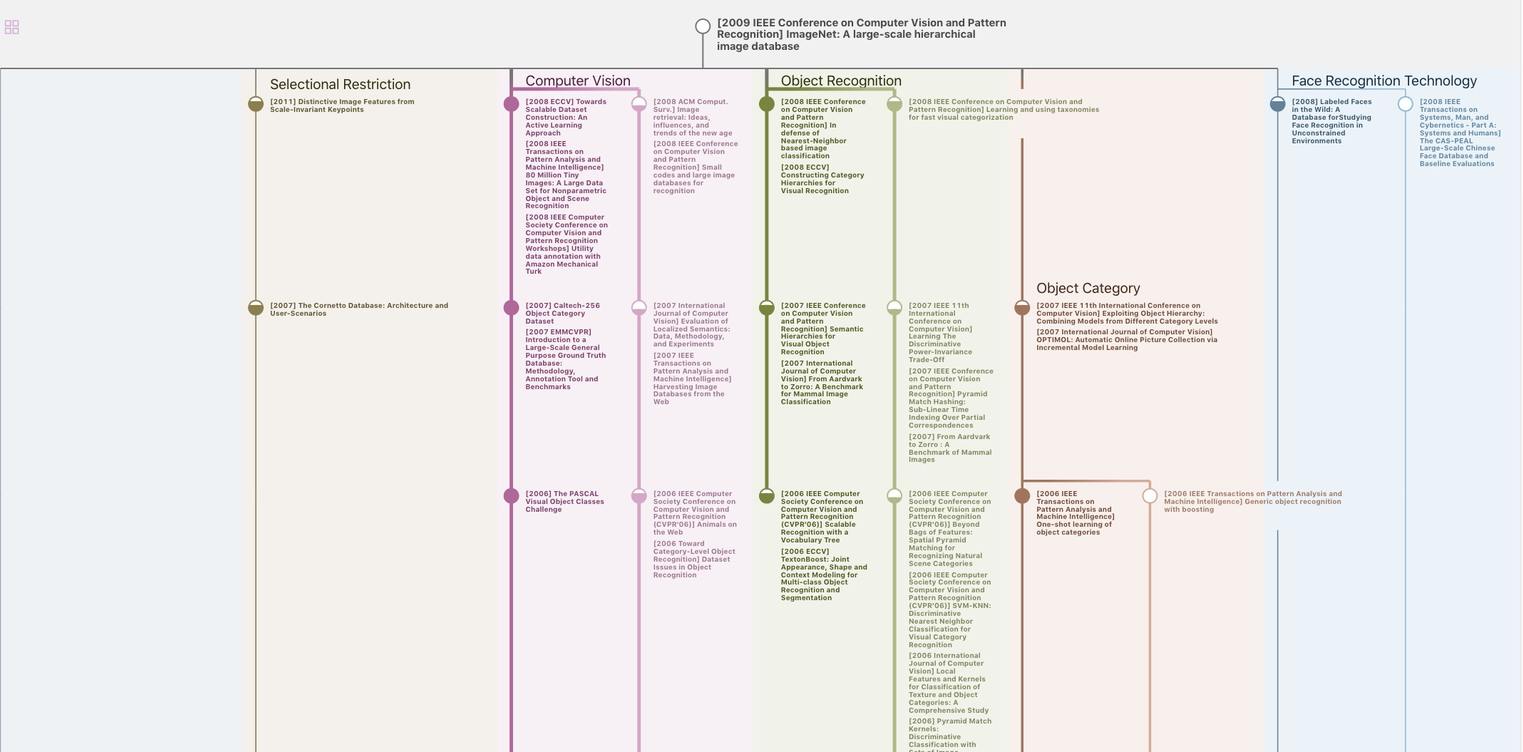
生成溯源树,研究论文发展脉络
Chat Paper
正在生成论文摘要