Cluster cascades: Infer multiple underlying networks using diffusion data
ASONAM(2014)
摘要
Information diffusion and virus propagation are the fundamental processes often taking place in networks. The problem of devising a strategy to facilitate or block such process has received a considerable amount of attention. A major challenge therein is that the underlying network of diffusion is often hidden. Most researchers dealing with this issue assume only one underlying network over which cascades spread. However, in the real world, whether the transmission pathways of a contagion, a piece of information, emerge or not depends on many factors, such as the topic of the information and the time when the information is first mentioned. In our opinion, it is impractical to model the diffusion processes by using only a single network when information is of all kind and diffuses in different underlying topic-specific networks. In this paper, we formulate a problem of K-network inference, inferring K underlying diffusion networks, based on a proposed probabilistic generative mixture model that models the generation of cascades. We further propose an algorithm that could cluster similar cascades and infer the corresponding underlying network for each cluster in the Expectation-Maximization framework. Finally, in experiments, we show that our algorithm could cluster cascades and infer the underlying networks effectively.
更多查看译文
关键词
expectation-maximisation algorithm,diffusion,inference mechanisms,transmission pathways,social network,topic-specific networks,information dissemination,expectation-maximization framework,cluster cascades,clustering,information diffusion,diffusion data,probabilistic generative mixture model,k-network inference,virus propagation,network theory (graphs),multiple underlying networks
AI 理解论文
溯源树
样例
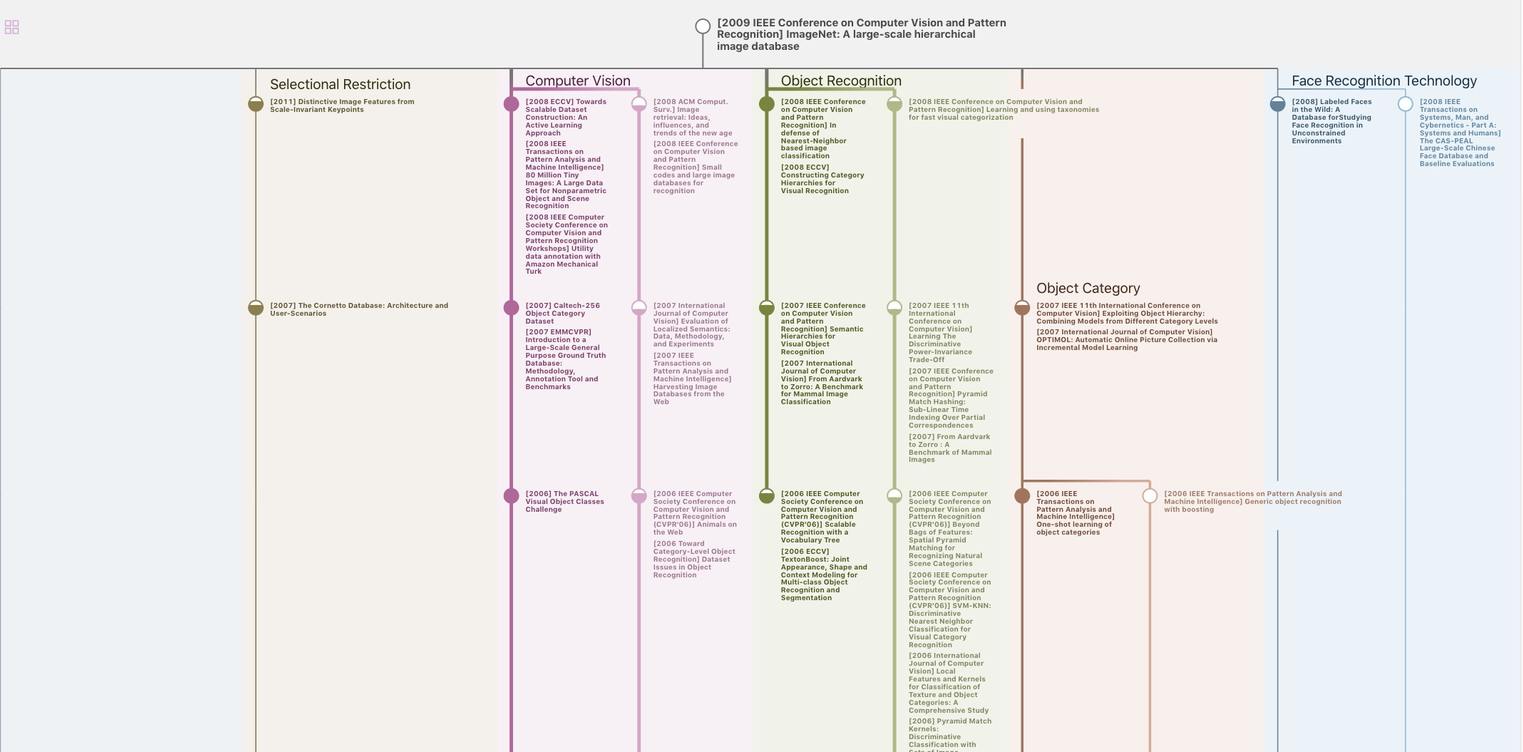
生成溯源树,研究论文发展脉络
Chat Paper
正在生成论文摘要