Storm: A Stochastic Recognition And Mining Processor
ISLPED(2014)
摘要
Recognition and Mining applications are becoming prevalent across the entire spectrum of computing platforms, and place very high demands on their capabilities. We propose a Stochastic Recognition and Mining processor (StoRM), which uses Stochastic Computing (SC) to efficiently realize computational kernels from these domains. Stochastic computing facilitates compact, power-efficient realization of arithmetic operations by representing and processing information as pseudo-random bit-streams. However, the overhead of conversion between representations, and the exponential relationship between precision and bit-stream length, are key challenges that limit the efficiency of stochastic designs. The proposed architecture for StoRM consists of a 2D array of Stochastic Processing Elements (StoPEs) with a streaming memory hierarchy, enabling binary-to-stochastic conversion to be amortized across rows or columns of StoPEs. We propose vector processing and segmented stochastic processing in the StoPEs to mitigate the unfavorable tradeoff between precision and bit-stream length. We also exploit the compactness of StoPEs to increase parallelism, thereby improving performance and energy efficiency. Finally, leveraging the resilience of RM applications to approximations in their computations, we design StoRM to support modulation of the stochastic bit-stream length, and utilize this capability to to optimize energy for a desired output quality. StoRM achieves 2-3X energy-delay improvements over a conventional design without sacrificing output quality, and upto 10X (20X) improvements when upto 5% (10%) loss in output quality is allowed. Our results also demonstrate that the proposed design techniques greatly enhance the applicability and benefits of stochastic computing.
更多查看译文
关键词
Stochastic Computing,Recognition and Mining,Approximate Computing,Energy Efficiency
AI 理解论文
溯源树
样例
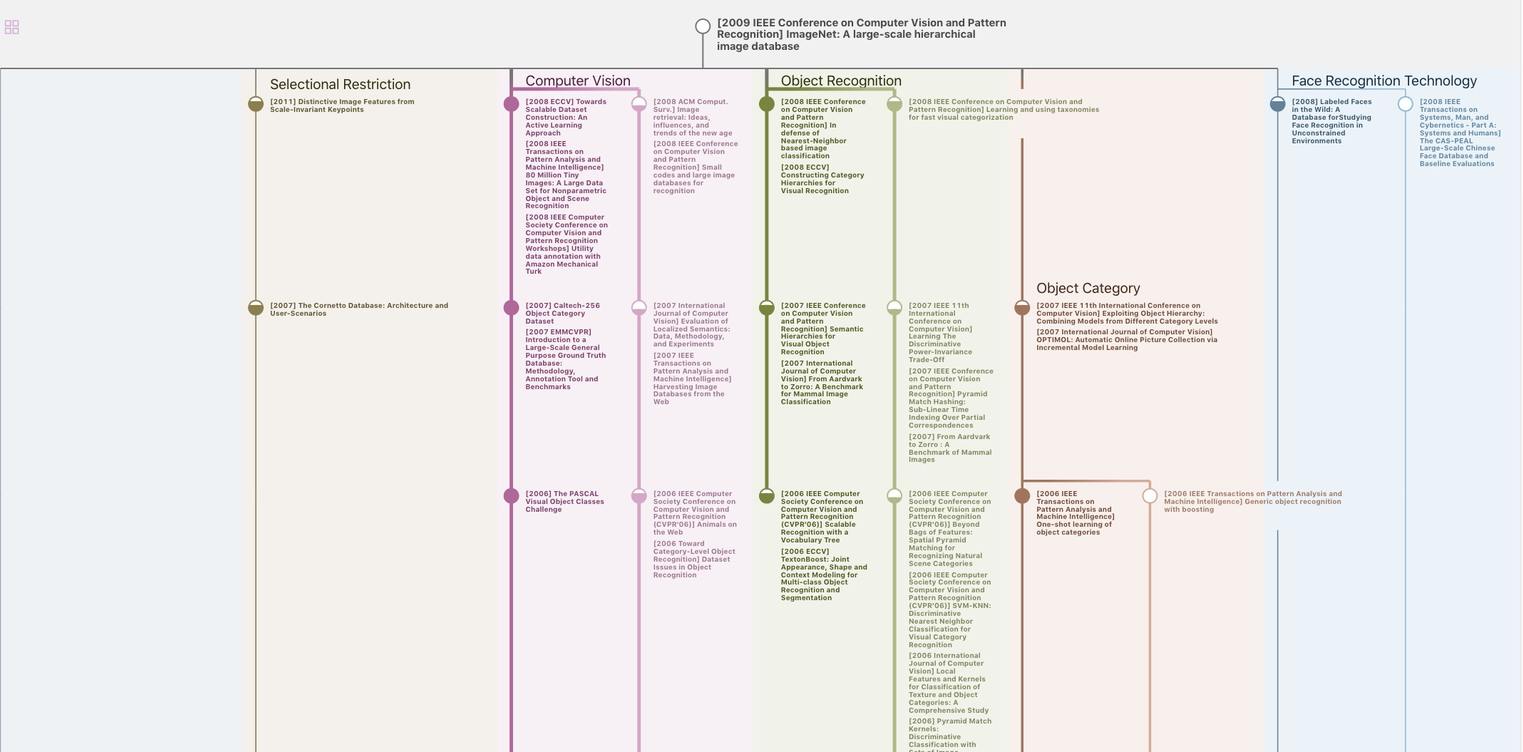
生成溯源树,研究论文发展脉络
Chat Paper
正在生成论文摘要