Improving Automatic Image Annotation with Google Semantic Link
SKG(2014)
摘要
During the past few years, there has been a massive explosion of multimedia content such as un-annotated images on the web. Automatic image annotation is an important task for multimedia retrieval. By automatically allocating semantic concepts to un-annotated images, image retrieval can be performed over annotation concepts. In this work, we address the problem of automatic image annotation, namely automatically describing semantic content of image by concept classifier. Traditional approaches mainly consider the link between image and concept, but ignore the link between annotation concepts. We propose a novel Google Semantic link based image Annotation Model (GSAM), which can leverage the associated concept network (ACN) to enhance automatic semantic annotation performance. When several concepts appear in training set with high co-occurrence frequency, our model utilizes Google semantic link to increase the chances of predicting one concept if there is strong visual evidence for others. Additionally, the fusion between Google concept link and local concept link, and semantic links between single-concepts and multi-concepts are employed to improve annotation performance. In order to investigate the feasibility and effectiveness of our approach, we conduct experiments on Corel and IAPR datasets. The experimental results show that our approach considering semantic link outperforms existing state-of-the-art methods.
更多查看译文
关键词
semantic link,google distance,multimedia content,corel dataset,google semantic link,gsam model,automatic image annotation,automatic semantic annotation,iapr dataset,concept classifier,image classification,image retrieval,automatic semantic annotation, semantic link, google distance, machine learning,machine learning,semantic concepts,multimedia retrieval,search engines,visualization,semantics,mathematical model,correlation
AI 理解论文
溯源树
样例
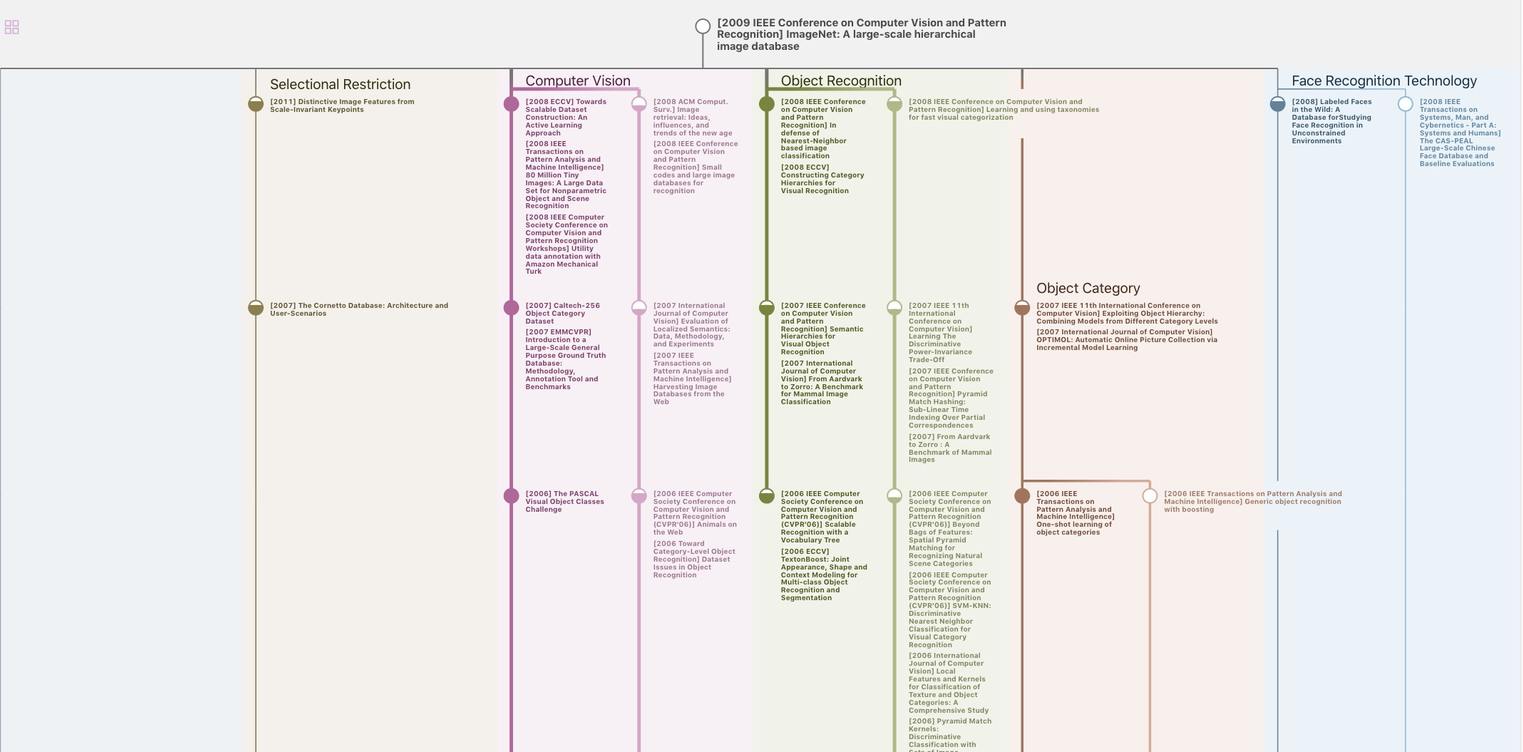
生成溯源树,研究论文发展脉络
Chat Paper
正在生成论文摘要