A pairwise algorithm for training multilayer perceptrons with the normalized risk-averting error criterion
IJCNN(2014)
摘要
Proper use of the normalized risk-averting error (NRAE) criterion has been shown to avoid nonglobal local minima effectively in the mean squared error (MSE) criterion. For training on large datasets, a pairwise algorithm for the NRAE criterion similar to the widely-used least mean square algorithm for the MSE criterion is proposed. The gradual deconvexification method employing this pairwise algorithm is tested on examples with built-in nonglobal local minima that are difficult to avoid and on recognition of handwritten numerals with the MNIST dataset. Numerical experiments show that the pairwise algorithm for the NRAE criterion is computationally more economical than the corresponding batch algorithm and delivers multilayer perceptrons with better performances than training methods based on the MSE criterion.
更多查看译文
关键词
normalized risk-averting error criterion,learning (artificial intelligence),mean squared error criterion,pairwise algorithm,multilayer perceptrons,nrae criterion,least mean squares methods,multilayer perceptron training,mse,batch algorithm,nonglobal local minima,mnist dataset,least mean square algorithm,gradual deconvexification method,handwritten numeral recognition,learning artificial intelligence,vectors,training data,function approximation
AI 理解论文
溯源树
样例
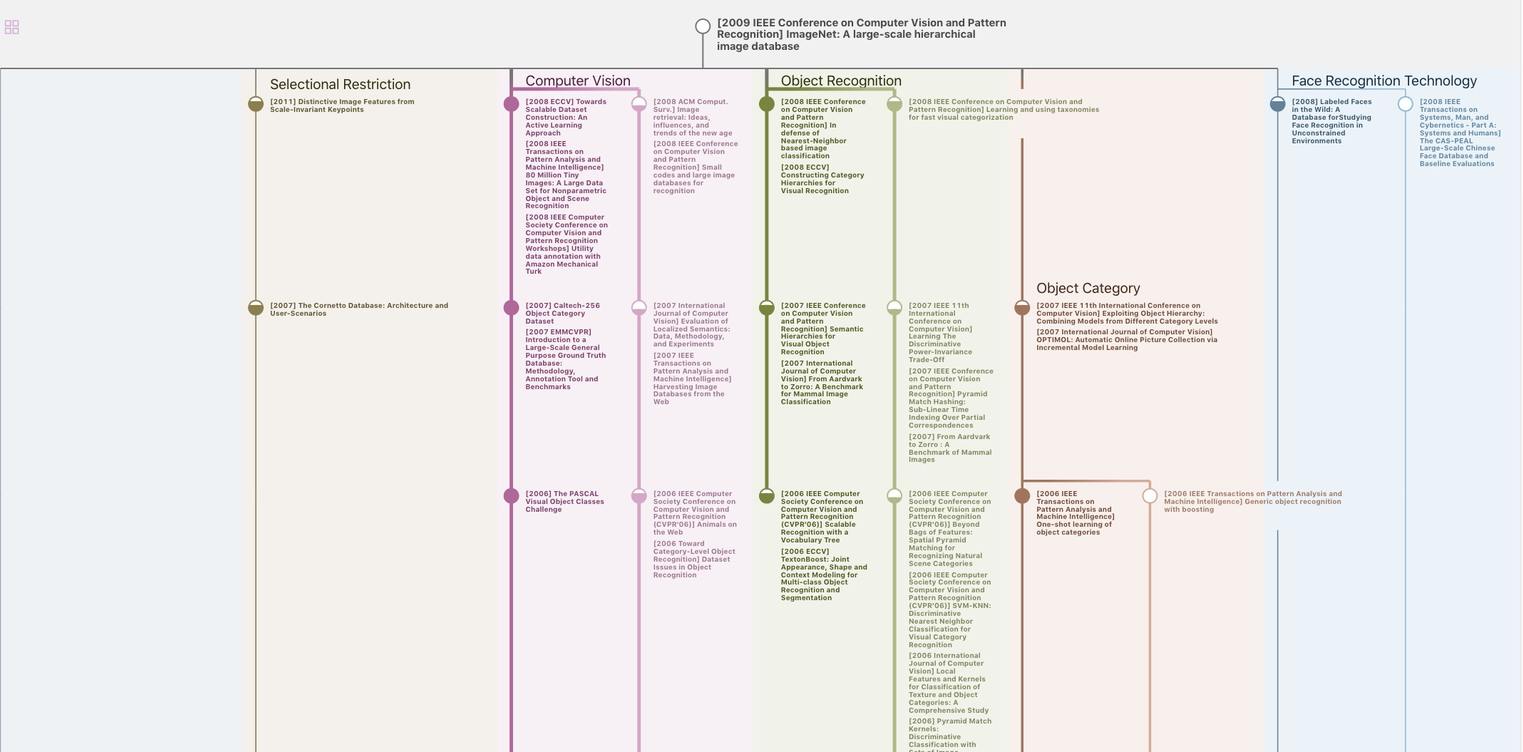
生成溯源树,研究论文发展脉络
Chat Paper
正在生成论文摘要