Regression Ensemble With Pso Algorithms Based Fuzzy Integral
2014 IEEE CONGRESS ON EVOLUTIONARY COMPUTATION (CEC)(2014)
摘要
Similar to the ensemble learning for classification, regression ensemble also tries to improve the prediction accuracy through combining several "weak" estimators which are usually high-variance and thus unstable. In this paper, we propose a new scheme of fusing the weak Priestley-Chao Kernel Estimators (PCKEs) based on Choquet fuzzy integral, which differs from all the existing models of regressor fusion. The new scheme uses Choquet fuzzy integral to fuse several target outputs from different PCKEs, in which the optimal bandwidths are obtained with cross-validation criteria. The key of applying fuzzy integral to PCKE fusion is the determination of fuzzy measure. Considering the advantage of particle swarm optimization (PSO) algorithm on convergence rate, we use three different PSO algorithms, i.e., standard PSO (SPSO), Gaussian PSO (GPSO) and GPSO with Gaussian jump (GPSOGJ), to determine the general and lambda fuzzy measures. The finally experimental results on 6 standard testing functions show that the new paradigm for regression ensemble based on fuzzy integral is more accurate and stable in comparison with any individual PCKE. This demonstrates the feasibility and effectiveness of our proposed regression ensemble model.
更多查看译文
关键词
testing,learning artificial intelligence,classification,regression analysis,fuzzy set theory,ensemble learning,bandwidth,convergence rate,kernel
AI 理解论文
溯源树
样例
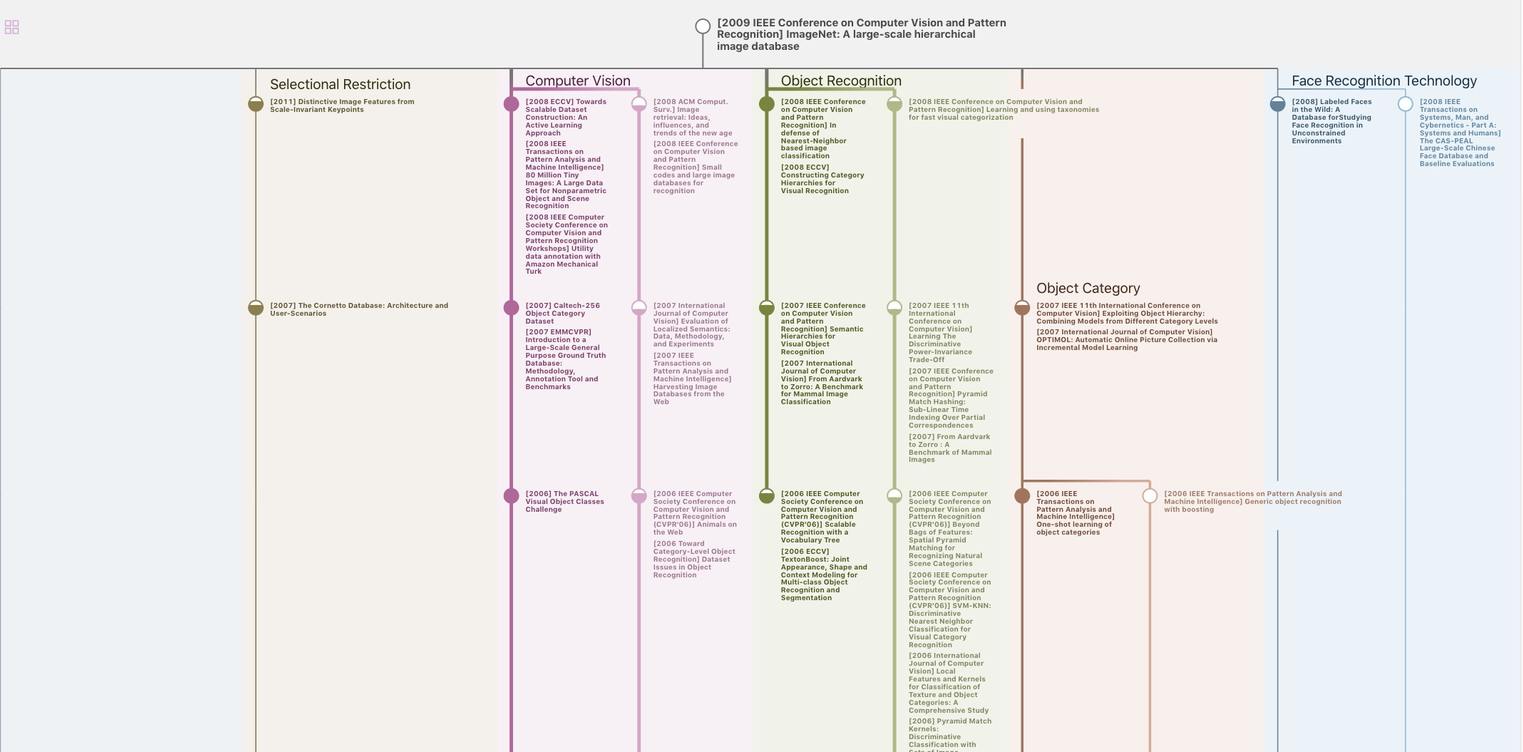
生成溯源树,研究论文发展脉络
Chat Paper
正在生成论文摘要