A multi-swarm particle swarm optimization with orthogonal learning for locating and tracking multiple optimization in dynamic environments
IEEE Congress on Evolutionary Computation(2014)
摘要
Due to the specificity and complexity of the dynamic optimization problems (DOPs), those excellent static optimization algorithms cannot be applied in these problems directly. So some special algorithms only for DOPs are needed. There is a multi-swarm algorithm with a better performance than others in DOPs, which utilizes a parent swarm to explore the search space and some child swarms to exploit promising areas found by the parent swarm. In addition, a static optimization algorithm OLPSO is so attractive, which utilize an orthogonal learning (OL) strategy to utilize previous search information (experience) more efficiently to predict the positions of particles and improve the convergence speed. In this paper, we bring the essence of OLPSO called OL strategy to the multi-swarm algorithm to improve its performance further. The experimental results conducted on different dynamic environments modeled by moving peaks benchmark show that the efficiency of this algorithm for locating and tracking multiple optima in dynamic environments is outstanding in comparison with other particle swarm optimization models, including MPSO, a similar particle swarm algorithm for dynamic environments.
更多查看译文
关键词
ol strategy,moving peak benchmark,learning (artificial intelligence),particle swarm optimisation,dop,multiple optimization tracking,static optimization algorithm olpso,multiple optimization locating,dynamic optimization problems,mpso,child swarms,multiswarm particle swarm optimization,dynamic programming,search space,dynamic environments,orthogonal learning,parent swarm
AI 理解论文
溯源树
样例
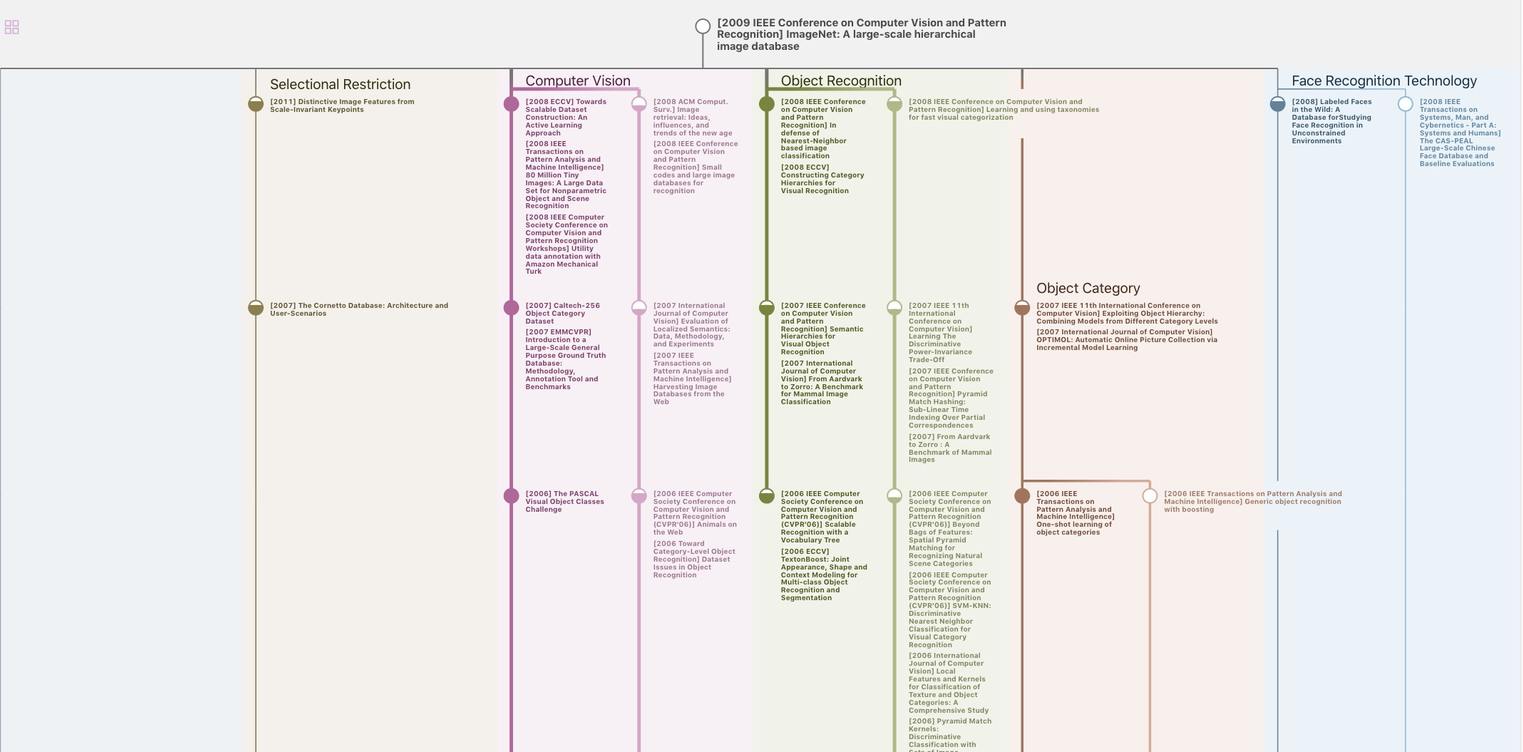
生成溯源树,研究论文发展脉络
Chat Paper
正在生成论文摘要