Learning Interactions for Social Prediction in Large-scale Networks.
CIKM '14: 2014 ACM Conference on Information and Knowledge Management Shanghai China November, 2014(2014)
摘要
Social networks have already emerged as inconceivably vast information repositories and have provided great opportunities for social connection and information diffusion. In light of these notable outcomes, social prediction is a critical research goal for analyzing and understanding social media and online social networks. We investigate underlying social theories that drive the characteristics and dynamics of social networks, including homophily, heterophily, and the structural hole theories. We propose a unified coherent framework, namely mutual latent random graphs (MLRGs), to exploit mutual interactions and benefits for predicting social actions (e.g., users' behaviors, opinions, preferences or interests) and discovering social ties (e.g., multiple labeled relationships between users) simultaneously in large-scale social networks. MLRGs introduce latent, or hidden factors and coupled models with users, users' actions and users' ties to flexibly encode evidences from both sources. We propose an approximate optimization algorithm to learn the model parameters efficiently. Furthermore, we speedup this algorithm based on the Hadoop MapReduce framework to handle large-scale social networks. We performed experiments on two real-world social networking datasets to demonstrate the validity and competitiveness of our approach.
更多查看译文
AI 理解论文
溯源树
样例
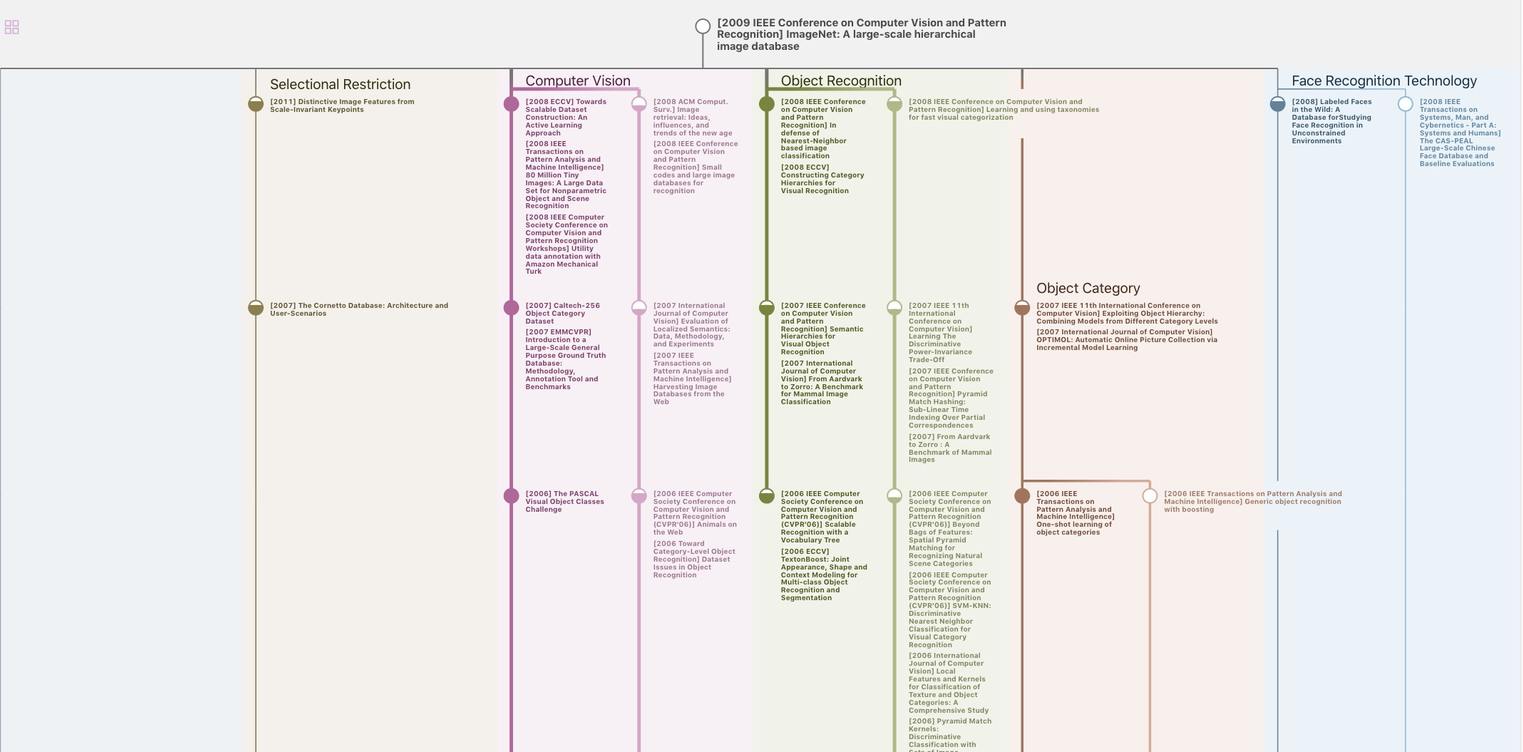
生成溯源树,研究论文发展脉络
Chat Paper
正在生成论文摘要