Multi-task Multi-view Learning for Heterogeneous Tasks.
CIKM '14: 2014 ACM Conference on Information and Knowledge Management Shanghai China November, 2014(2014)
摘要
Multi-task multi-view learning deals with the learning scenarios where multiple tasks are associated with each other through multiple shared feature views. All previous works for this problem assume that the tasks use the same set of class labels. However, in real world there exist quite a few applications where the tasks with several views correspond to different set of class labels. This new learning scenario is called Multi-task Multi-view Learning for Heterogeneous Tasks in this study. Then, we propose a Multi-tAsk MUlti-view Discriminant Analysis (MAMUDA) method to solve this problem. Specifically, this method collaboratively learns the feature transformations for different views in different tasks by exploring the shared task-specific and problem intrinsic structures. Additionally, MAMUDA method is convenient to solve the multi-class classification problems. Finally, the experiments on two real-world problems demonstrate the effectiveness of MAMUDA for heterogeneous tasks.
更多查看译文
AI 理解论文
溯源树
样例
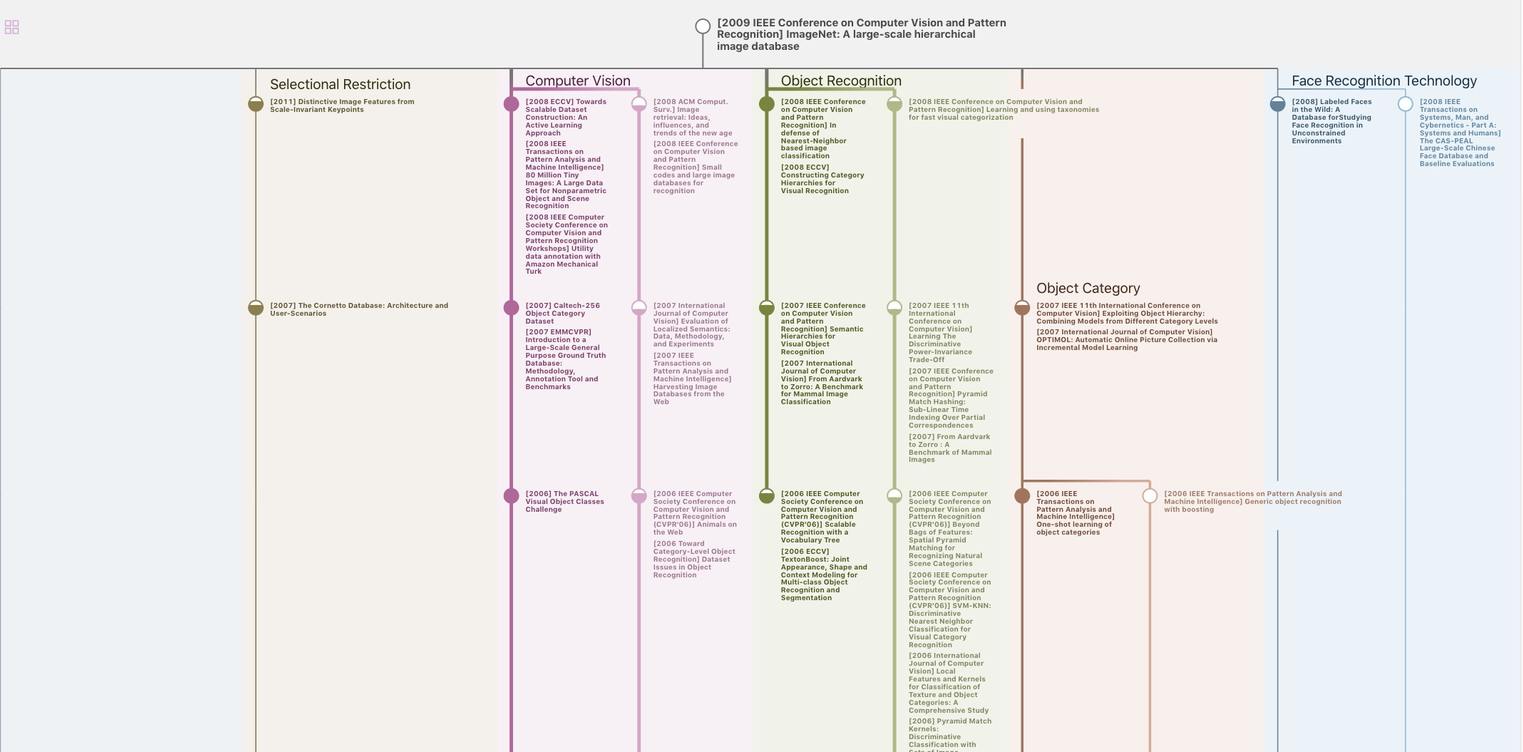
生成溯源树,研究论文发展脉络
Chat Paper
正在生成论文摘要