Mining Latent Entity Structures From Massive Unstructured And Interconnected Data
SIGMOD/PODS'14: International Conference on Management of Data Snowbird Utah USA June, 2014(2014)
摘要
The "big data" era is characterized by an explosion of information in the form of digital data collections, ranging from scientific knowledge, to social media, news, and everyone's daily life. Examples of such collections include scientific publications, enterprise logs, news articles, social media and general Web pages. Valuable knowledge about multi-typed entities is often hidden in the unstructured or loosely structured but interconnected data. Mining latent structured information around entities uncovers sematic structures from massive unstructured data and hence enables many high-impact applications.In this tutorial, we summarize the closely related literature in database systems, data mining, Web, information extraction, information retrieval, and natural language processing, overview a spectrum of data-driven methods that extract and infer such latent structures, from an interdisciplinary point of view, and demonstrate how these structures support entity discovery and management, data understanding, and some new database applications. We present three categories of studies: mining conceptual, topical and relational structures. Moreover, we present case studies on real datasets, including research papers, news articles and social networks, and show how interesting and organized knowledge can be discovered by mining latent entity structures from these datasets.
更多查看译文
关键词
Latent structure,Entity Knowledge Engineering
AI 理解论文
溯源树
样例
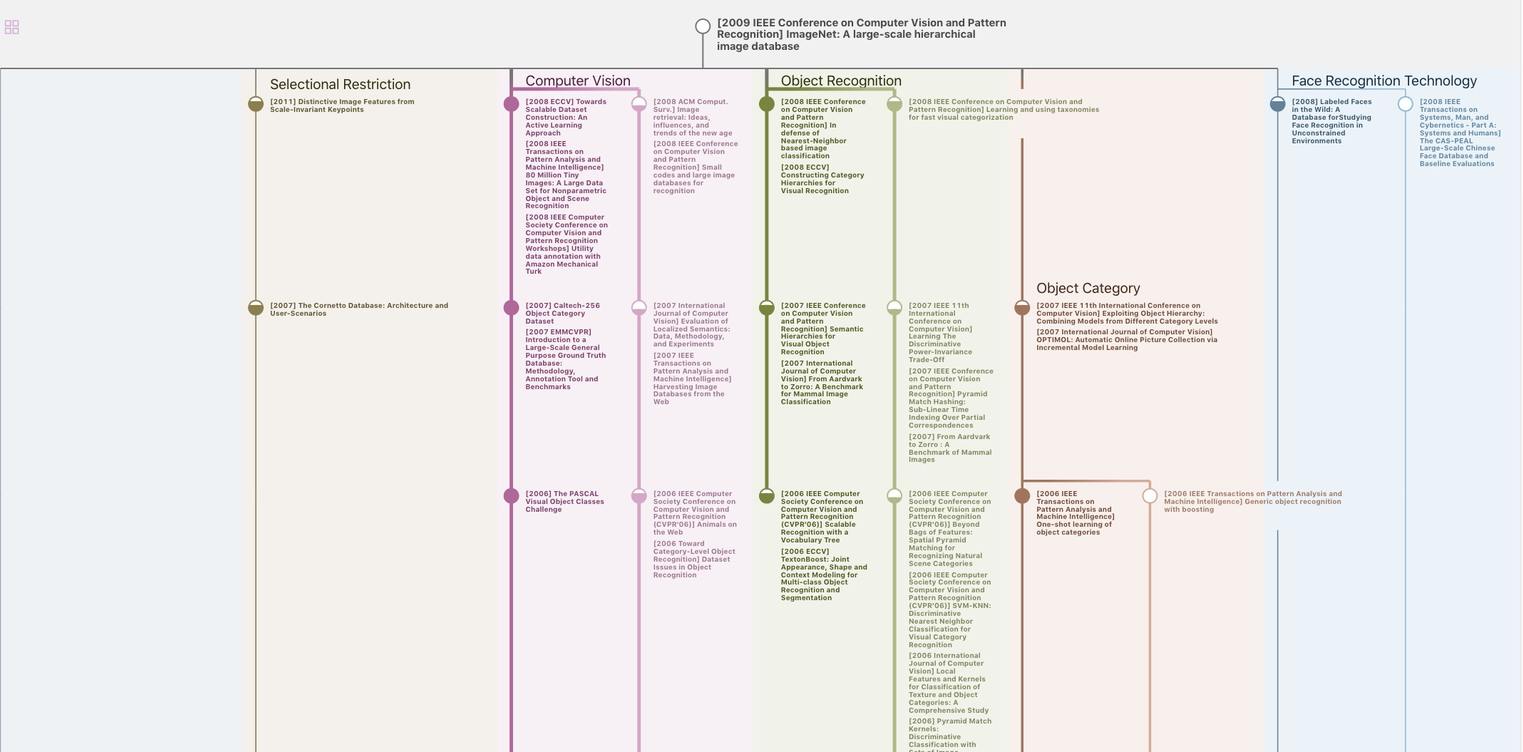
生成溯源树,研究论文发展脉络
Chat Paper
正在生成论文摘要